For the data scientists and developers who use analytics to extract insights from massive volumes of data, it’s easy to see the appeal of operating in a metacloud environment.
After all, analytics workloads are not only resource-intensive – they’re also highly variable and differ in terms of importance and urgency. The concept of a metacloud offers the ability to spin out different workloads to the right cloud environment for the job or even back to mainframes.
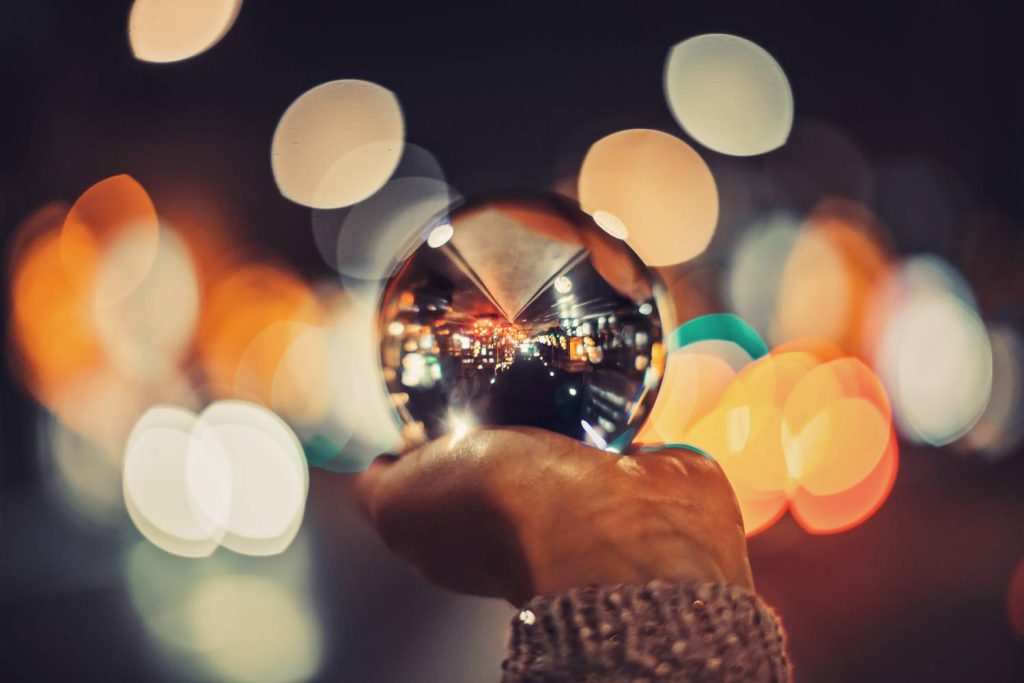
This is an exciting proposition for data scientists, who could prioritize large-scale, mission-critical jobs by moving them from desktop machines and on-prem servers to a more flexible, scalable cloud environment at a moment’s notice, getting much faster results. Easy? Not necessarily. But certainly smoother and faster than whatever happens in most large organizations today.
Green lights – and warning signs
For other stakeholders, though, the prospect of running analytics through a metacloud is more complicated – and maybe even dangerous. Finance leaders and C-level executives, for example – people who regularly make decisions based on analytics-driven insights – are keenly aware that the meter is running every second an analytics workload is being run through the cloud. The bigger the workload and the longer the runtime, the more it will cost. Nobody wants to be left holding the bill for a massive, unplanned analytics-in-the-cloud exercise. So, decisions regarding metacloud-driven analytics workloads must be made with all these variables in mind in order to deliver the best results at the right cost.
While the metacloud is not a practical reality yet, it’s coming.
- Jay Upchurch, SAS CIO
Fortunately, this is exactly the type of business challenge that analytics tools were built to address. As organizations find smarter ways to manage their reliance on several cloud environments at once, analytics, AI, machine learning, and automation capabilities will help inform these decisions. Experienced users of analytics will be given choices regarding where to run analytics workloads, based on several factors, like the type of analytics model being run; the size of the job and how long it will take; the best cloud environment for that job; and cost.
Of course, human decision makers will ultimately be making these decisions, not machines. And while machines will be able to determine where analytics workloads can run with the least cost, humans will maintain responsibility for weighing a higher cost against an organization’s business goals.
Help is on the way
So are these analytics capabilities – many of which evolved from more rudimentary tools like schedulers, which have been used by all types of analytics teams for years. And that’s very good news for analytics decision makers who are expected to deliver the world without blowing up IT budgets.