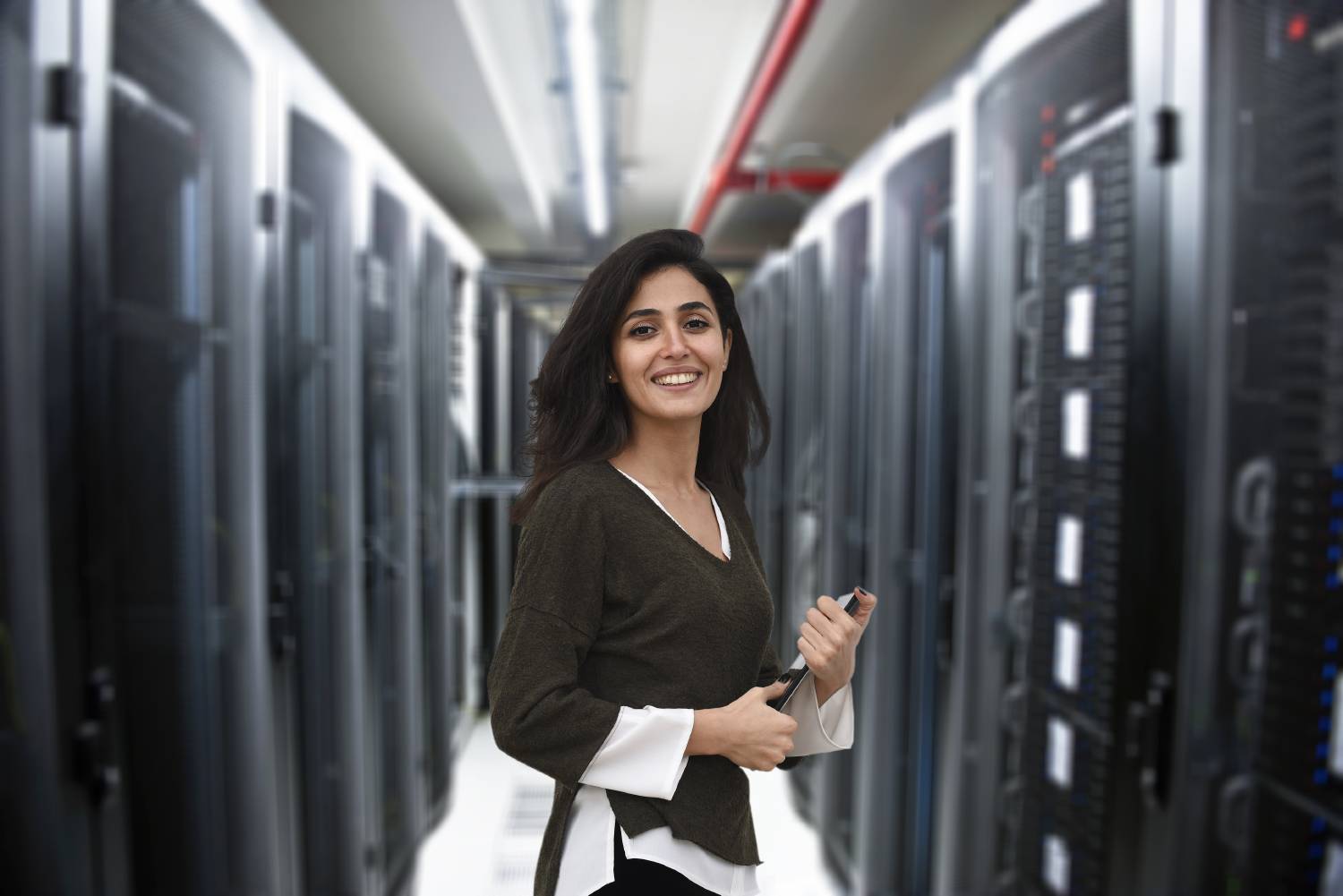
Jim Harris takes a deep dive into data lakes and how they relate to the cloud.
Jim Harris takes a deep dive into data lakes and how they relate to the cloud.
Minnesota's longitudinal data system integrates early childhood education, K-12, postsecondary and workforce data to create a panoramic view of education outcomes. The merging of systems results in data linking and improves the overall data quality and performance of the P-20 Statewide Longitudinal Education Data System (SLEDS) and Early Childhood Longitudinal
Guest blogger Khari Villela shares tips to help you skip common pitfalls of building a data lake.
Datenmodellierung ist sicher eine der komplexesten Aufgaben beim Aufbau eines Data Warehouse (DWH). Dies liegt vor allem daran, dass in der Phase der Modellierung unterschiedlichste Analyseanforderungen zu berücksichtigen sind. Und teilweise ändern sich diese Anforderungen schneller, als man mit dem Datenmodellieren vorankommt. Aktuelle Gründe für ständige Änderungen sind zum Beispiel
Guest blogger Khari Villela says data lakes are not a cure-all – they're just one part of a comprehensive, strategic architecture.
Focus on data governance, quality and storage if you want to do data management for analytics right.
In the extended enterprise, data integration challenges abound. David Loshin explains.
David Loshin explores considerations for organizations gradually making the transition to Hadoop.
Do you know how master data management and data warehouses are different? Jim Harris explains.
It's that time of year again where almost 50 million Americans travel home for Thanksgiving. We'll share a smorgasbord of turkey, stuffing and vegetables and discuss fun political topics, all to celebrate the ironic friendship between colonists and Native Americans. Being part Italian, my family augments the 20-pound turkey with pasta –
In my last post, we explored the operational facet of data governance and data stewardship. We focused on the challenges of providing a scalable way to assess incoming data sources, identify data quality rules and define enforceable data quality policies. As the number of acquired data sources increases, it becomes
In Part 1 of this two-part series, I defined data preparation and data wrangling, then raised some questions about requirements gathering in a governed environment (i.e., ODS and/or data warehouse). Now – all of us very-managed people are looking at the horizon, and we see the data lake. How do
Data governance can encompass a wide spectrum of practices, many of which are focused on the development, documentation, approval and deployment of policies associated with data management and utilization. I distinguish the facet of “operational” data governance from the fully encompassed practice to specifically focus on the operational tasks for
I'm a very fortunate woman. I have the privilege of working with some of the brightest people in the industry. But when it comes to data, everyone takes sides. Do you “govern” the use of all data, or do you let the analysts do what they want with the data to
It's the age of big data and the internet of things (IoT), but how will that change things for insurance companies? Do insurers still need to consider classic data warehouse concepts based on a relational data model? Or will all relevant data be stored in big data structures and thus
Why they will still play a valuable role in organizational data-management and -integration efforts.
Auditability and data quality are two of the most important demands on a data warehouse. Why? Because reliable data processes ensure the accuracy of your analytical applications and statistical reports. Using a standard data model enhances auditability and data quality of your data warehouse implementation for business analytics.
It's a common problem in any industry: getting a large number of similar requests for information. But with limited resources and an already overburdened staff, how do you handle it? At El Paso Community College, analysts from the Institutional Research (IR) team enlisted the help of IT to create a data
Back before storage became so affordable, cost was the primary factor in determining what data an IT department would store. As George Dyson (author and historian of technology) says, “Big data is what happened when the cost of storing information became less than the cost of making the decision to
While setting up meetings with business consumers developing a data warehouse environment, I was involved in some very interesting conversations. Following are some of the assumptions that were made during these conversations, as well as a few observations. To get a well-rounded view of this topic, read my earlier post that focuses on the IT perspective.
The other day I was in a meeting with a client and there was an argument about who owns the data. Those arguing were IT people. In this scenario, the assumption was that data from source systems would flow into and integrate with a data warehouse. I found the discussion very interesting. Here are some of the
Twenty-five years ago (when I was 12 years old), we realized that data, across the corporation, was not integrated. Nor did our data let us predict the future by looking at the past. So we started creating these stores of historical data soon to be called “data warehouse.” Here are
The other day I was chatting with an ETL developer and he said he always pushes queries into the database instead of dragging data across the network. I thought “Hmm, I remember talking about those topics when I was a DBA.” I'd like to share those thoughts with you now.
Many people perceive big data management technologies as a “cure-all” for their analytics needs. But I would be surprised if any organization that has invested in developing a conventional data warehouse – even on a small scale – would completely rip that data warehouse out and immediately replace it with an NoSQL
“Field of dreams warehouse”– a historic phrase I used in the early days of data warehouse development. It describes the frenzy of activity that took place to create enterprise data infrastructure, before the business rationale for the data use was even understood. Those were the early days. In some ways
Determining the life cycle of event stream data requires us to first understand our business and how fast it changes. If event data is analyzed, it makes sense that the results of that analysis would feed another process. For example, a customer relationship management (CRM) system or campaign management system like
Hadoop is increasingly being adopted as the go-to platform for large-scale data analytics. However, it is still not necessarily clear that Hadoop is always the optimal choice for traditional data warehousing for reporting and analysis, especially in its “out of the box” configuration. That is because Hadoop itself is not
Hadoop recently turned eight years old, but it was only 3-4 years ago that Hadoop really started gaining traction. It had many of us “older” BI/DW folks scratching our heads wondering what Hadoop was up to and if our tried-and-true enterprise data warehouse (EDW) ecosystems were in jeopardy. You didn't
Working out where Hadoop might fit alongside, or where it might replace components, of existing IT architectures is a question on the minds of every organization that is being drawn towards the promises of Hadoop. That is the main focus of this blog along with discussions of some of the reasons they
Demand for analytics is at an all-time high. Monster.com has rated SAS as the number one skill to have to increase your salary and Harvard Business Review continues to highlight why the data scientist is the sexiest job of the 21st century. It is clear that if you want to be