Small data is akin to algebra; big data is like calculus.
Tag: data quality
In the movie Big, a 12-year-old boy, after being embarrassed in front of an older girl he was trying to impress by being told he was too short for a carnival ride, puts a coin into an antique arcade fortune teller machine called Zoltar Speaks, makes a wish to be big,
If you are looking for a way to fund your data quality objectives, consider looking in the closets of the organization. For example, look for issues that cost the company money that could have been avoided by better availability of data, better quality of the data or reliability of the
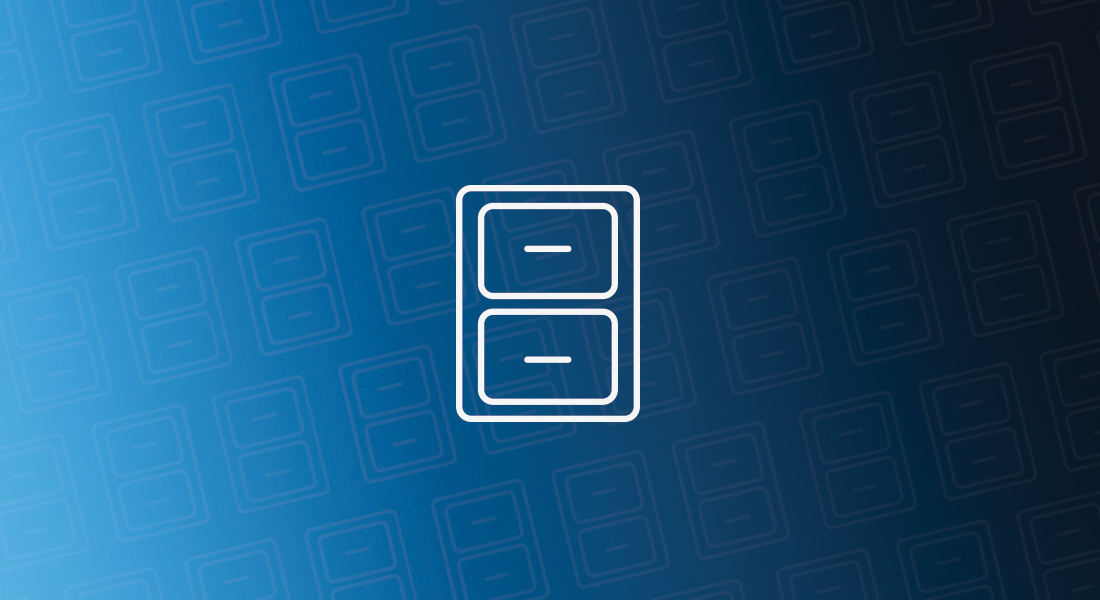
Data Management has been the foundational building block supporting major business analytics initiatives from day one. Not only is it highly relevant, it is absolutely critical to the success of all business analytics projects. Emerging big data platforms such as Hadoop and in-memory databases are disrupting traditional data architecture in
In this blog series, I am exploring if it’s wise to crowdsource data improvement, and if the power of the crowd can enable organizations to incorporate better enterprise data quality practices. In Part 1, I provided a high-level definition of crowdsourcing and explained that while it can be applied to a wide range of projects
.@philsimon on the reliability of social numbers.
Once in a while, people run into an issue with the data that doesn't really need to be fixed right to ensure success of a specific project. So, the data issues are put into production and forgotten. Everyone always says, “We will go back and correct this later.” But that
Regulatory compliance is a principal driver for data quality and data governance initiatives in many organisations right now, particularly in the banking sector. It is interesting to observe how many financial institutions immediately demand longer timeframes to help get their 'house in order' in preparation for each directive. To the
In this blog series, I am exploring if it’s wise to crowdsource data improvement, and if the power of the crowd can enable organizations to incorporate better enterprise data quality practices. In Part 1, I provided a high-level definition of crowdsourcing and explained that while it can be applied to a wide range of projects
There are companies that have no data quality initiative, and truly do believe that if they see no data problem. In effect, they say that if it does not interfere with day-to-day business, then there is no data quality problem. From what I have seen in my consulting experience, it usually
Over my last two posts, I suggested that our expectations for data quality morph over the duration of business processes, and it is only at a point that the process has completed that we can demand that all statically-applied data quality rules be observed. However, over the duration of the
One of the significant problems data quality leaders face is changing people's perception of data quality. For example, one common misconception is that data quality represents just another data processing activity. If you have a data warehouse, you will almost certainly have some form of data processing in the form
In my last post, I pointed out that we data quality practitioners want to apply data quality assertions to data instances to validate data in process, but the dynamic nature of data must be contrasted with our assumptions about how quality measures are applied to static records. In practice, the

Utilizing big data analytics is currently one of the most promising strategies for businesses to gain competitive advantage and ensure future growth. But as we saw with “small data analytics,” the success of “big data analytics” relies heavily on the quality of its source data. In fact, when combining “small” and “big” data
@philsimon on the need to recognize DQ differences.
It’s common at the start of a new year to create a long list of resolutions that we hope to achieve. The reality, of course, is by February those resolutions will likely be a distant memory. The key to making any resolution stick is to start small. Create one small
After working in the data quality industry for a number of years, I have realized that most practitioners tend to have a rather rigid perception of the assertions about the quality of data. Either a data set conforms to the set of data quality criteria and is deemed to be acceptable
This isn't Kansas anymore. Oz has become a sprawling, smart metropolis filled with sensor data. How do we make sense of, clean, govern and glean value from this big data so we can get Dorothy home? The answer is SAS Data Management. With the latest portfolio updates, customers will be
James Surowiecki wrote a book about The Wisdom of Crowds. Jeff Howe, who co-coined the term crowdsourcing, wrote a book about Why the Power of the Crowd Is Driving the Future of Business. In this blog series, I explore if it’s wise to crowdsource data improvement, and if the power of the crowd can
.@philsimon on the different folks you'll encounter in many large organizations.
Since now is the time when we reflect on the past year and make resolutions for next year, in this post I reflect on my Data Roundtable posts from the past year and use them to offer a few New Year’s data resolutions for you and your organization to consider in
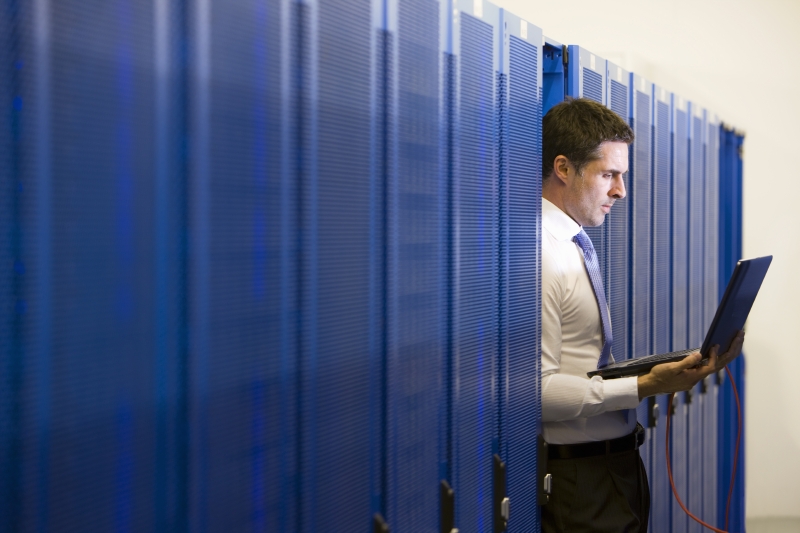
I have participated in many discussions about master data management (MDM) being “just” about improving the quality of master data. Although master data management includes the discipline of data quality, it has a much broader scope. MDM introduces a new approach for managing data that isn't in scope of traditional data quality
As this is the week of Christmas, many, myself included, have Christmas songs stuck in their head. One of these jolly jingles is Santa Claus Is Coming To Town, which includes the line: “He knows if you’ve been bad or good, so be good for goodness sake!” The lyric is a
I have a rule – any conversion or upgrade will require the creation of a decommission plan. A decommission plan should include the following: A list and definition of each database, table and column (source and target). A list and definition of each of the current programs in use (you
The physical data model should represent exactly the way the tables and columns are designed in the in the database management system. I recommend keeping storage, partitioning, indexing and other physical characteristics in the data model if at all possible. This will make upkeep and comparison with the development, test
We've explored data provenance and the importance of data lineage before on the Data Roundtable (see here). If you are working in a regulated sector such as banking, insurance or healthcare, it is especially important right now and one of the essential elements of data quality that they look for
I have a question --- do we need a logical data model for a conversion? Here are my thoughts. I believe the answer is yes if the conversion has any of the following characteristics: The target application is created in-house. This application will more than likely be enhanced in the
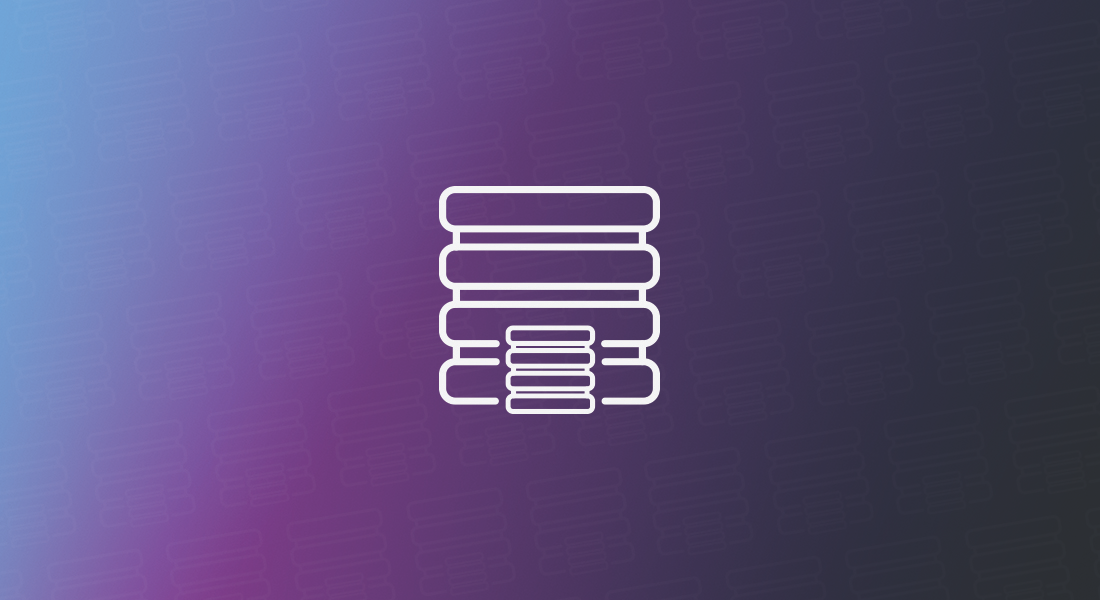
La grandeza de sus datos probablemente no es la característica más importante. De hecho, puede que ni siquiera figure dentro de los aspectos relevantes por los cuales usted debería preocuparse. La calidad, la integración de los silos, la manipulación y la extracción de valor de los datos no estructurados siguen
In my previous post I explained that even if your organization does not have anyone with data steward as their official job title, data stewardship plays a crucial role in data governance and data quality. Let’s assume that this has inspired you to formally make data steward an official job title. How
To perform a successful data conversion, you have to know a number of things. In this series, we have uncovered the following about our conversion: Scope of the conversion Infrastructure for the conversion Source of the conversion Target for the conversion Management for the conversion Testing and Quality Assurance for