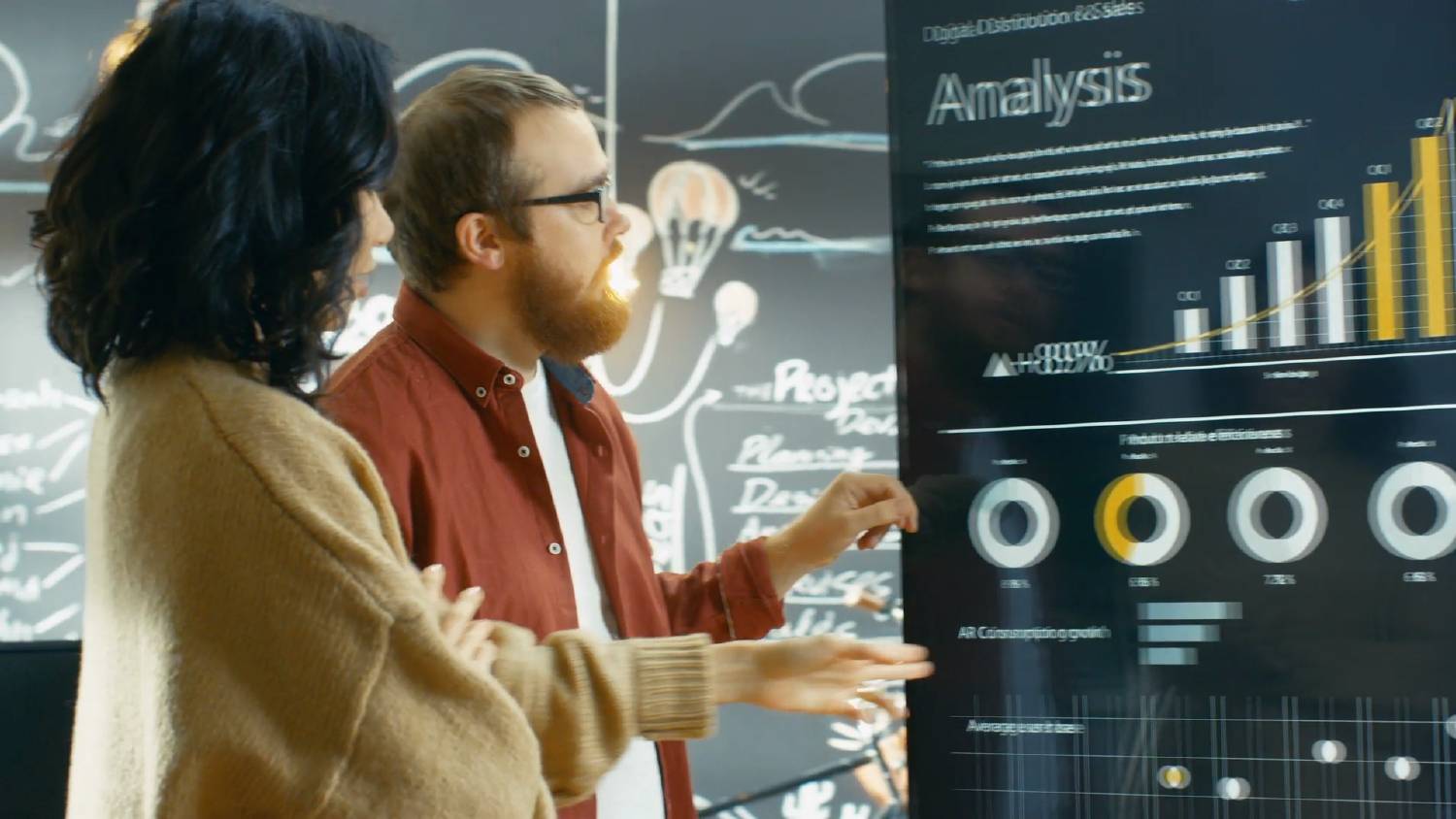
Jim Harris shows how data, analytics and humans work together to form the "insight equation."
Jim Harris shows how data, analytics and humans work together to form the "insight equation."
Learn how to get started with self-service data prep in these go-to articles.
Jeff Stander helps us understand the different options of preparing data for analytics.
Proper data prep means faster, better analytics. Guest blogger Jenine Milum shares tips.
.@philsimon chimes in about data-related accomplishments and challenges.
Phil Simon flip-flops from his last post.
Jim Harris says data stewards are essential to analytics, providing life cycle management for data across the enterprise.
Joyce Norris-Montanari cites five things she considers essential to data management for analytics.
Phil Simon chimes in with some tips on how to set these folks loose.
How should a data trust process work? David Loshin elaborates.
Focus on data governance, quality and storage if you want to do data management for analytics right.
Jim Harris says learn the lineage of the data that fed the analysis before you get dazzled by visualizations or algorithms.
David Loshin raises questions about what needs to be done to ensure quality analytics.
Phil Simon shares some lessons from his consulting career.
Jim Harris warns against allowing your data lake to become a poorly managed and ungoverned data dumping ground.
Avoid frustrations by following these 5 tips from David Loshin to create a successful data management strategy for analytics.
If you need more than just well-mixed data, take a look at data preparation from SAS.
@philsimon chimes in about how success in one area can lead to success in another.
To get full value from analytics programs, Todd Wright says be sure you can first access, integrate, cleanse and govern your data.
To do their jobs well, Joyce Norris-Montanari says users doing analytical data preparation need access to both standardized and raw data.
Phil Simon weighs in on the value of getting your own hands dirty using self-service data prep.
Phil Simon chimes in on the immediacy of enterprise data.
Helmut Plinke explains why modernizing your data management is essential to supporting your analytics platform.
As the application stack supporting big data has matured, it has demonstrated the feasibility of ingesting, persisting and analyzing potentially massive data sets that originate both within and outside of conventional enterprise boundaries. But what does this mean from a data governance perspective?
One aspect of high-quality information is consistency. We often think about consistency in terms of consistent values. A large portion of the effort expended on “data quality dimensions” essentially focuses on data value consistency. For example, when we describe accuracy, what we often mean is consistency with a defined source
.@philsimon on the need to adopt agile methodologies for data prep and analytics.
In Part 1 of this two-part series, I defined data preparation and data wrangling, then raised some questions about requirements gathering in a governed environment (i.e., ODS and/or data warehouse). Now – all of us very-managed people are looking at the horizon, and we see the data lake. How do
Lately I've been binge-watching a lot of police procedural television shows. The standard format for almost every episode is the same. It starts with the commission or discovery of a crime, followed by forensic investigation of the crime scene, analysis of the collected evidence, and interviews or interrogations with potential suspects. It ends
.@philsimon chimes in on new data-gathering methods and what they mean for analytics.
I'm a very fortunate woman. I have the privilege of working with some of the brightest people in the industry. But when it comes to data, everyone takes sides. Do you “govern” the use of all data, or do you let the analysts do what they want with the data to