In this post, Ajay Agrawal, professor at Toronto's Rotman School of Management, discusses the challenges of unlocking the full potential of AI and ML for businesses and banks. Agrawal explains how the taxi industry in London, UK provides a cautionary tale of the potential impediments to driving value from AI, despite the extensive training London cabbies undergo. He also touches on the impact of ChatGPT and its potential to transform the world, as well as the importance of considering regulation of the adoption and use of AI.
If you want to understand the impediments to driving value from artificial intelligence, the taxi industry provides an object lesson, says Ajay Agrawal, professor at Toronto’s Rotman School of Management and co-author of Prediction Machines: The Simple Economics of Artificial Intelligence. Specifically the taxi industry in London, U.K.
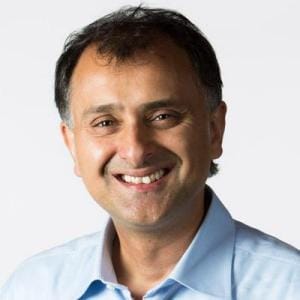
Unlike in North American cities, London cabbies spend three years preparing for their licenses, Agrawal said in an interview ahead of his appearance at the AI and the Shift of Power - Understanding the Dominance of New Technologies in Banking roundtable on March 2.
They spend the first year studying maps of the metropolis’s infernally complex road system. The second is spent tracing routes on mopeds. Their exams include questions like, “It’s 4:00 on a Thursday afternoon in November, and your passenger wants to go from the Churchill War Rooms to the Royal Botanical Gardens. What route do you take?”
It seems like a perfect application for predictive analytics. And in fact, a study handed some cabbies navigational AI. Expecting a huge improvement? Inexperienced cabbies got seven percent more productive; experienced drivers got zero. Meanwhile, Uber has four million drivers, most with no experience, all able to navigate the most efficient route in real time, thanks to AI.
The weight of existing infrastructure and process stand in the way of value-driven artificial intelligence.
– Ajay Agrawal
If four million drivers drive a car worth an average price of $25,000, Agrawal says that the system has unlocked an approximate $100 billion capital expenditure. It’s a formula business across all industries would love to replicate, but this is easier said than done. And in North America, where banking executives don’t consider AI and ML essential to stay ahead, the technologies’ ability to unlock this level of value remains elusive.
Q: What do you think remains the biggest barrier for businesses and banks to be equipped to adopt AI?
Agrawal: Five years ago, or even three years ago, we would probably have said the greatest barrier was access to data for training the models. But as we’ve seen this field develop and mature, we’ve come to the view now that the biggest barrier is the inertia in organizations that prevents them from the system-level redesign required to fully utilize this powerful new technology. Fifteen years ago, banks had data scientists that were doing fraud detection. Taxi drivers were not using statistics to optimize their route decisions.
We can now make some reasonably high-fidelity predictions in an environment not designed to utilize predictions like that. You can’t just pull out the old predictions and drop in the new ones; everything works better. You have to redesign the system.
The banks already had sophisticated predictive analytics before these recent advances in machine intelligence. So, when these new capabilities came along, they were able to almost surgically go in, pull out the old predictive analytic tools and drop in these new predictive analytic tools. Still, the rest of the business stays the same.
Q: Everybody’s talking about ChatGPT [a new conversational technology for interacting with AI]. Where do you see it making the greatest positive impact on the economy?
Agrawal: [ChatGPT] has made the power of these foundation models and what’s referred to as generative AI understandable to laypeople. People have worked on these large language models for many years, but nobody outside the field has paid any attention.
Most people experience ChatGPT and they just can’t understand how statistics can generate language. And yet those same statistics will be used to generate video, graphics and things in the real world.
Let’s say I’m in a warehouse and ask a robot, “Oh, can you unpack those boxes and put the shoes on a shelf?” That’s a simple command issued in English. But there are lots of steps you and I don’t even think about. A robot has to take that simple sentence and break it into many steps using verbs like “open grasp,” “move,” and “release.” Something like ChatGPT can transcribe a simple sentence, transforming it into a much more detailed set of instructions that a robot can execute. Even though ChatGPT seems like it creates words on a computer, it can actually have quite big implications for transforming things in the physical world that are beyond words.
Q: What is the most important element of drafted legislation regulating AI adoption and use?
Agrawal: That depends on what the regulatory motivation is. Highly regulated industries—financial services is one, healthcare is another, transportation is another—in each case, we’re worrying about different things. In banking we might be worried about preventing fraud, enhancing stability, and preventing discrimination.
Let me just take the one issue of discrimination, which is a big one. Today, the common narrative is, we must be very careful. In fact, many believe we should severely curtail the use of artificial intelligence because it amplifies bias. And furthermore, it hides bias because you can’t interpret what these AIs are doing and how they make their decisions.
Five to 10 years from now, I think many will view AIs as the safest way to make decisions that minimize discrimination. Most people don’t understand this yet: While you can’t open up the black box and understand the details of how the neural network works, what you can do with AI that you can’t do with a human is ask them an infinite number of questions and they will always answer.
Imagine you were talking to a bank loan officer and said, “You denied this person a loan.” And they were, let’s say, a particular race, a marginalized race, and you were concerned that this was maybe even unconscious discrimination. And you might ask the loan officer, “Would you have denied that loan if the person was exactly the same, except they were of a different race?” No human would admit, “Oh, yes, I would have given them this loan if they had been white instead of black.” But AI will.
You can just give the AI the data and say, “Hey, you denied this loan. If I give you the exact same person and the only thing different is their race, would you give them the loan?” And the AI will say, “Yep, I’d give it to them.” You can ask a million questions like that. AI is infinitely scrutable in a way humans are not. That means we can detect discrimination in ways we can’t do with humans.
This is an area where governments can set regulations in a manner that is as lightweight as possible so it doesn’t create lots of extra encumbrances. For example, criteria for how we test AIs for bias. Or that every AI can be subjected to various tests designed and implemented by government or government representatives. We can standardize and bring these to market in a way we currently do not do with people.