Rapid demand response forecasting techniques are forecasting processes that can incorporate key information quickly enough to act upon in real time by agile supply chains.
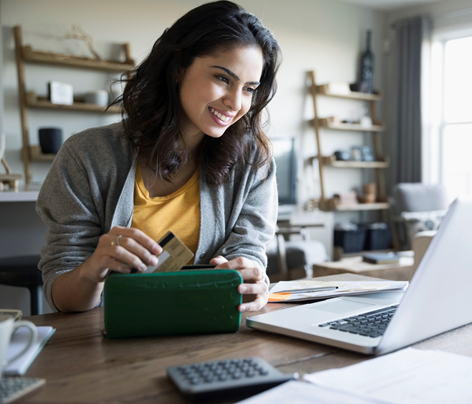
Retailers and consumer goods suppliers are urgently trying to determine how changes in consumer behavior will affect their regions, channels, categories, brands and products during and beyond the COVID-19 crisis, and what actions they can take now. They must find new ways to adapt to the shifting consumer behaviors in response to the pandemic. The key to success is adjusting strategies immediately to improve business performance while preparing for the new normal.
The length of time it takes for the demand forecasting process to incorporate rapid changes or short-term spikes in demand is a challenge for most companies. Short-term spikes can occur with retail store promotions, sudden changes in weather conditions, social media ambiances, and, of course, pandemics.
The concept of rapid demand response (RDR) forecasting is based on updating demand forecasts to reflect real and rapid changes in demand, both during and between planning cycles. In this case, reacting to a pandemic – responding, recovering and reimagining the new normal.
Another challenge is enabling RDR forecasting. It's not easy given the explosion of digital data and the enormous amount of information available regarding automated consumer engagement. When data expands as a result of the Internet of Things (IoT), streaming data from devices can make it difficult to decipher demand signals from the noise. In many cases, these short-term spikes exceed the projected baseline demand, and are not always a result of planned sales promotions or marketing events -- like an unforeseen pandemic.
Success story
The following is a success story of how SAS helped a regional European online grocer implement an RDR forecasting process using advanced analytics and machine learning to solve its short-term forecasting challenges.
Overview: As a result of COVID-19, normal consumer patterns have changed, to say the least. Activities like a trip to the grocery store or dining out with friends are difficult at best, or even prohibited. While the hospitality industry has been hit hard, online shopping doesn't appear to be slowing down as we move from responding to recovering, and finally reimagining the new normal.
Situation: In several places around the world, grocers are among the few businesses that remain open. This has resulted in unprecedented increases in sales. According to Progressive Grocer, e-commerce grocery sales for home delivery and/or store pickup reached $5.3 billion in April, a 37% increase over March sales, which were already a new record.
COVID-19 thrust online retail grocers into the position of trying to fulfill urgent demand while meeting customers’ needs on the fly. The estimated percentages vary, but more consumers are turning to online grocery purchases, as they stay at home out of fear of the coronavirus.
Due to this situation, a regional online grocer and SAS customer experienced an increase in online grocery purchases of more than 200% virtually overnight. This generated substantial supply chain bottlenecks and product substitution challenges, creating order cancellations as consumers turn to digital channels to purchase food and other goods. It's likely that this shift to digital consumer experiences will continue to grow in popularity even after the coronavirus subsides. Companies that act quickly and modernize their delivery models to help consumers navigate the pandemic safely and effectively will have an advantage over their competitors.
Key challenges: Continuing shifts in consumer spending for groceries has forced this regional online grocer to work carefully in applying historical sales data to forecast future demand and accurately align products and categories with shoppers’ needs. Understanding true demand – and those disruptive events that impact each product and location – is critically important. Sales orders alone will not account for the changing demand patterns resulting from the COVID-19 pandemic. It will require investing in new data, advanced analytics and technology to make the necessary adjustments both now and in the future. Grocers that adapt to meet online consumer demand will rise above the crisis as community lifelines, ready for a larger shift in online sales.
Approach: Short-term demand sensing
SAS started by listening to the customer to understand its business process, help identify its challenges and ultimately determine the best possible solutions. This required an understanding of affected KPIs, categories, products and departments, and identification of the data necessary to solve the problem. The best approach was to implement a short-term forecasting process using advanced analytics and machine learning with internal and external data to predict detailed weekly demand across the product portfolio. Figure 1 (below) provides a summary of the data used in the models.
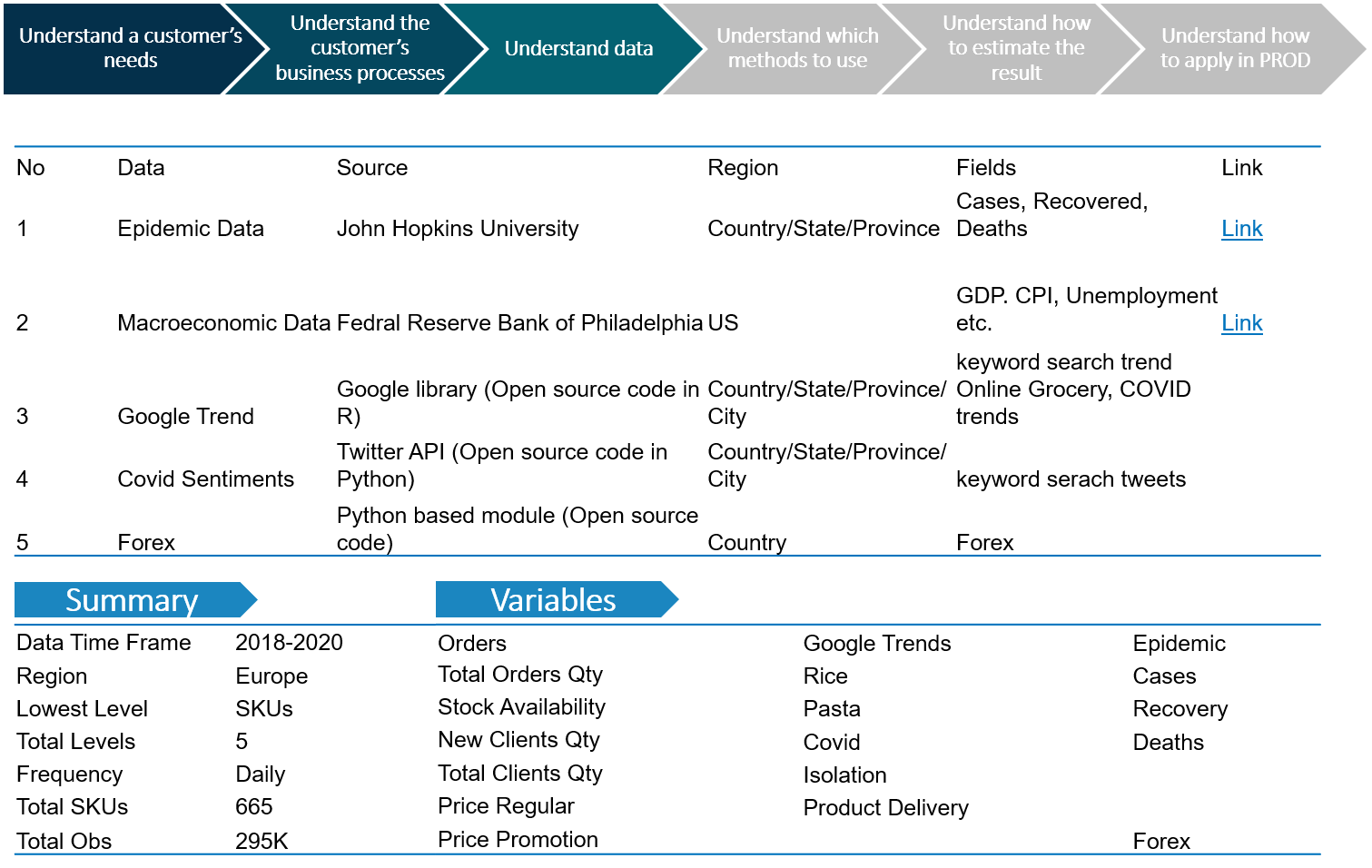
Note: The SASEFRED interface engine dramatically reduces the amount of time and effort required to include economic variables in your time series analysis.
Data investigation
Demand increased as consumers fearing shortages loaded their pantries. Public health concerns caused by rising exchange rates and government actions to fight COVID-19 had an inflationary effect and contributed to further increases in consumption for the region. A trend that will likely continue into recovery. Subsequently, the availability of goods decreased, creating low availability across many product categories.
Consumers reacted to the fear of a shortage by initially purchasing more goods, such as pet food and toilet paper. However, consumption eventually returned to previous levels after recovery, resulting in the post-crisis volume declines. Work-from-home and shelter-in-place policies forced consumers to shift from visiting food-service outlets to ordering from online retailers. The result is increased consumption of these products during and potentially after the crisis. See Figure 2 below.
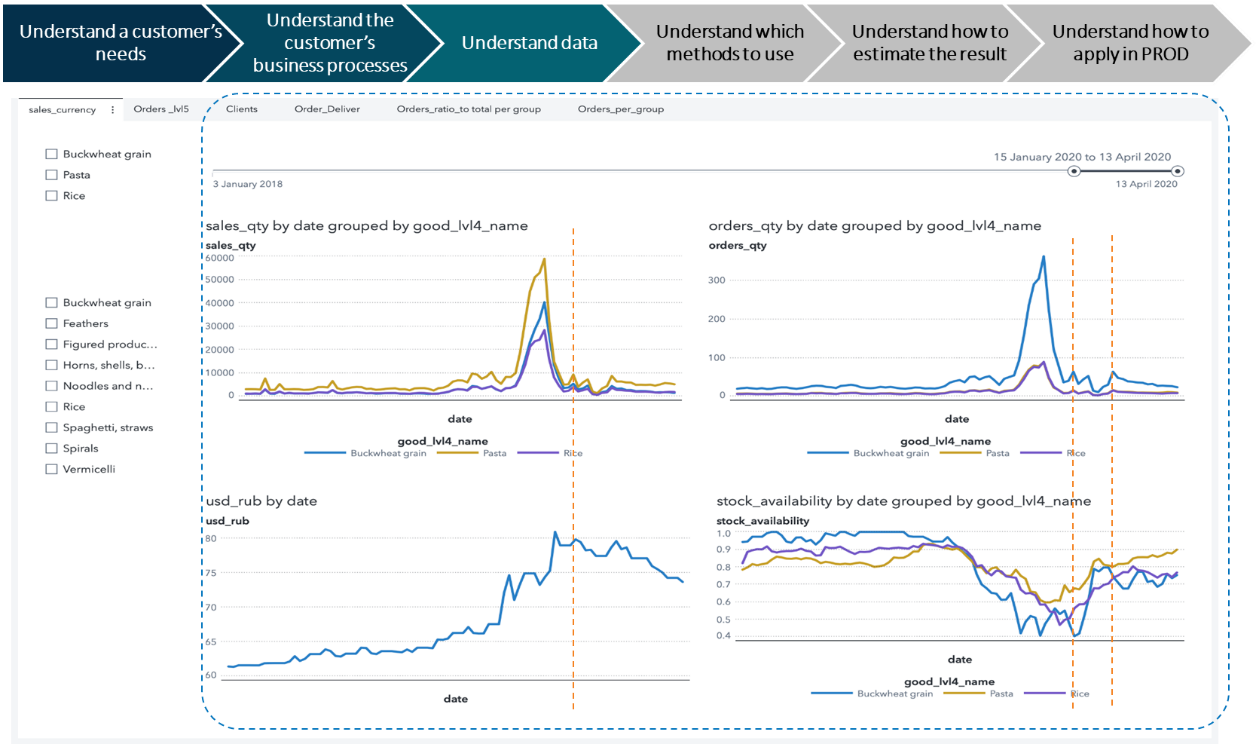
Analytics approach
Forecasting demand for grocery products is hard enough during normal conditions, let alone during a pandemic. Simple time series models that take historical demand for a product and extrapolate those patterns (trend and seasonality) into the future no longer work well under these conditions. It was necessary to build models using more advanced time series and machine learning models. These models can integrate additional information – like epidemiological data, exchange rates, Google trends and stringency index – that can influence future demand or improve the predictability of the models.
Fortunately, public health organizations have a good understanding of how pandemics spread, which SAS combined with other key data sources to take advantage of advanced analytics and machine learning models. The data was readily available to estimate the various parameters in the models and then run “what if” simulations to determine what will happen under various scenarios. Some of the parameter estimates were not as significant but allowed the grocer to test what happens to demand forecasts by varying the future values within a likely range. A simple model using demand history alone will be misleading unless it can incorporate the various actions by local governments, the medical community and changing consumer demand patterns in reaction to the pandemic.
By applying machine learning to product attribute data and other external data in combination with historical demand, the approach proved to be the most accurate way to predict demand by product and location – particularly where there was sparse sales history, or if sales history was disrupted. Implementing a hierarchical forecasting approach allowed additional external data and casual factors to be deployed at different levels of data aggregation – category and store levels. Additionally, it allowed the estimation of internal cannibalization effects from product out-of-stocks at various levels of the hierarchy, as well as capturing halo effects across categories and products.
The approach made it possible to estimate and predict the impact of the disruptive events influencing future demand through advanced causal modeling, with the flexibility to configure various event occurrences, duration and recurrence factors. The strategy of modeling the impact of the disruptive event at the product and location level, then testing multiple algorithms for best fit was significantly more accurate than using a one-model-fits-all approach. SAS was able to estimate event impacts and quantify the unique effects for each product and location, up and down the hierarchy. The retailer was able to consider multiple scenarios regarding the outcome of the disruptive events on future demand using robust real-time “what if” capabilities.
Results
Several categories and products were selected to model and forecast including noodles and nests, buckwheat grain, pastas horns, shells, butterfly and bows product groups. A four-step process was applied to test the accuracy of the models:
- Only use internal historical demand data.
- Include Google Trends.
- Include epidemiological data.
- Include exchange rates.
By adding various casual factors, the retailer could determine which factors improved the predictability of the models using MAPE (Mean Absolution Percentage Error) as the default performance metric. In other words, the lower the MAPE, the more accurate the model performed with those additional factors incorporated. See Figure 3 below.
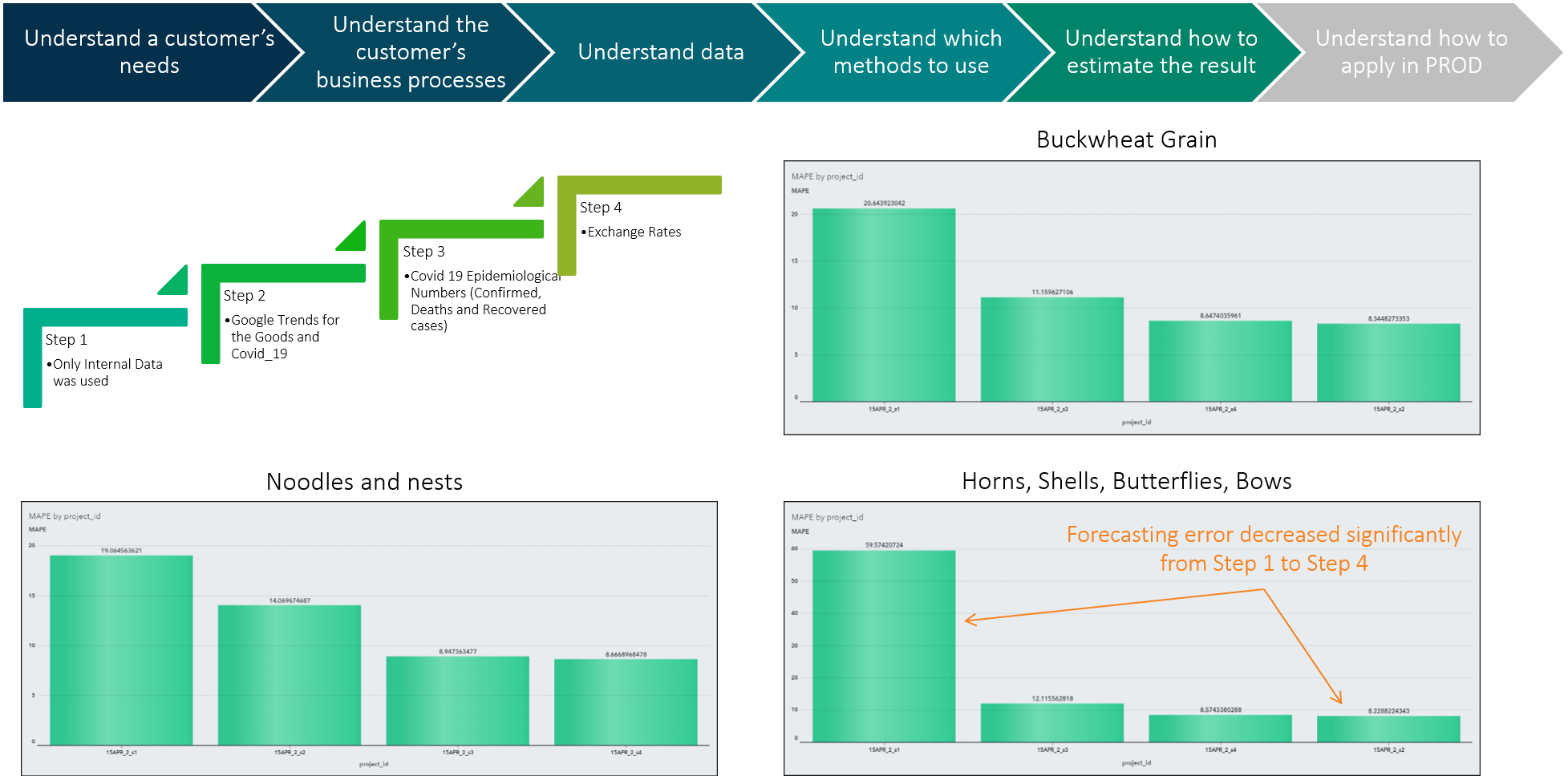
Starting with just historical demand data, the models’ average MAPE was anywhere between 20%-60%, a very high forecast error (low forecast accuracy). However, as additional causal factors were added, the forecast accuracy improved significantly. MAPE decreased significantly to between 4%-9%.
SAS delivered a real-time feed and automation of data, providing the demand planners an up-to-date view of global and regional patterns. SAS also delivered additional “what if” scenarios to adjust product forecasts based on key consumer sentiment. SAS can replicate this capability on any dedicated public cloud environment that a customer is using, or as a SaaS in the SAS cloud environment using standard SAS software, and deploy it in days. It would include an interactive end-to-end COVID-19 dashboard. See Figure 4 below.
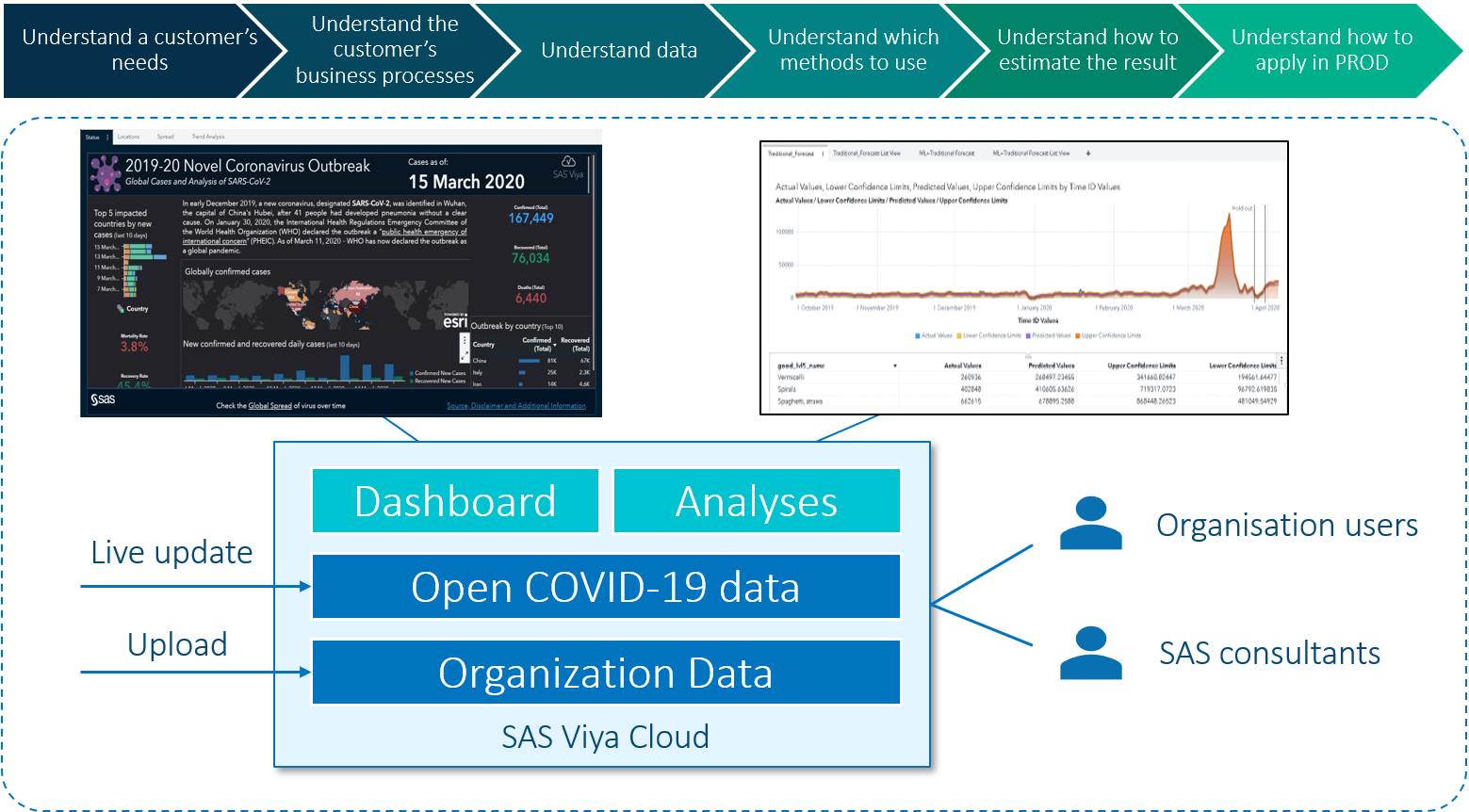
This advanced analytics approach is a proven methodology and straightforward solution to adopt and implement across a product portfolio. It is also a valuable practice to have in place for demand planning agility and execution.
Summary
Retail and CPG executives should plan to rapidly adapt their marketing and demand plans to reflect changing consumer demand patterns and sentiment. Additionally, they should quickly optimize their e-commerce channels, re-calibrating product volumes with the new demand patterns, emphasizing the fastest-selling SKUs. Retailers should expect daily resets to their demand forecasts, shifting online assortments accordingly, and adjusting supply chain logistics and distribution centers to meet online consumer demands.
Learn more by downloading the white paper, "Using Advanced Analytics to Model, Predict and Adapt to Changing Consumer Demand Patterns Affected by COVID-19" , Retail forecasting through a pandemic and by exploring SAS Retail & Consumer Goods Solutions in the Age of COVID-19.