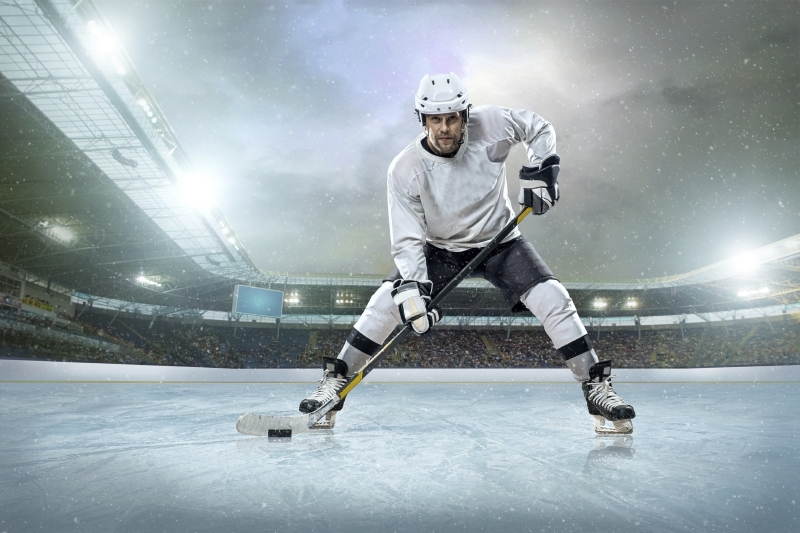
"I skate to where the puck is going to be, not where it has been." - Wayne Gretzky I love this quote from Wayne Gretzky. It sums up how most organizations approach data strategy. Data strategy typically starts with a strategic plan laid down by the board. The CEO will