With rapid advances in AI, it's natural to search for the next "big thing." But what if the real breakthrough isn't about looking ahead, but instead looking back – toward the vast amounts of information stored in paper and images? Much of this data may be digitized, but much of it still goes ignored and unused.
In addition to countless records sitting in filing cabinets and on shelves in agencies nationwide, the US National Archives maintains a vast network of underground caves in Kansas and Illinois, filled with millions of paper documents with records dating back over a century.
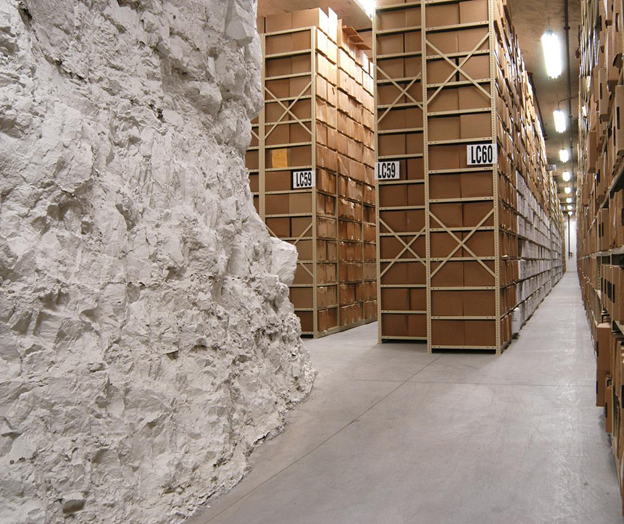
Despite rapid advances in AI and technological progress, most government agencies and business organizations are bogged down by the overwhelming task of processing paper documents. It consumes considerable time and resources.
The manual handling of applications, permits, licenses, and various forms of paperwork leads to inefficiencies, errors, and delays in service delivery. This cumbersome process hinders prompt response to customers' needs, adds to the difficulty in maintaining accurate and up-to-date records, and increases the risk of data loss and security breaches.
And it is expensive too! According to a 2022 report from the US Chamber of Commerce, processing paper-based forms costs the US government more than $38 billion annually. Document analysis techniques can help expedite, if not completely automated, much of the expensive drudgery associated with document processing and manual data entry workflows.
What is document intelligence?
So, how can we unlock the value of this data and utilize it to drive productivity and greater efficiency? The answer is via intelligent document processing (IDP), also known as Document Intelligence. Document Intelligence uses traditional techniques along with multimodal AI systems. It combines approaches such as computer vision (CV), optical recognition (OCR), and large language models (LLMs) to automate the "reading" and processing of scanned documents.
This method extracts structured and actionable information from scanned document images for use in analytics, reporting, and automated decision-making.
How will document intelligence help?
Substantial improvements have been made in CV and OCR model accuracy. They enable computers to transcribe scanned document images into machine-readable text with incredible accuracy, even in handwriting and very poor-quality document images. Meanwhile, over the past two years, LLMs and agent-based modeling have achieved impressive AI breakthroughs. They provide new tools for humans to interface with computer systems via natural language.
The next logical development involves synthesizing these technologies (along with additional text-to-speech and speech-to-text models) to produce genuinely multi-modal AI models. These models would be able to ingest both structured and unstructured data in various formats and perform logical reasoning across these sources to make decisions that otherwise require subjective judgment. There has been a justifiable flurry of excitement surrounding LLM developments.
However, it is important to note that these models tend to be biased towards digitally native documents. They also struggle to properly understand the OCR process's raw output.
The information trapped in decades of paper government documents is also a potential treasure trove for LLMs who have primarily been trained on digitally native documents. This ignores the vast amounts of information gathered and stored in paper-based documents and scanned document images and can introduce bias since much of the data tends to be text created during the recent Internet Age.
Robust IDP systems can expand the data available for advanced LLMs and other emerging technologies and enable users to leverage insights and rapid decisioning capabilities based upon these previously locked data sources.
Document Intelligence Solutions will provide the capability to automate much of the existing mundane, inefficient, and error-prone manual data entry and review workflow processes that currently exist. To be clear, the intention is not to remove humans from the process entirely but rather augment their existing practices and capabilities while automating routine, tedious tasks so that individuals can focus on situations that require their experience and subject matter expertise.
There will still be a need for some sort of human-in-the-loop (HITL) to guide the AI system, provide it with valuable feedback, and ensure that it produces the intended outputs and decisions. Additionally, as such solutions are more widely adopted, tracking and benchmarking such systems' reliability, performance, and ethical implications will become increasingly important.
Who will benefit most from this innovation?
Both businesses and governmental organizations will benefit financially from improved efficiencies and greater employee productivity. Additionally, the employees whose current jobs might be replaced by these developments will also stand to benefit from enabling the AI system to handle the most mundane aspects of their current roles.
Agencies and organizations will have the opportunity to re-assign these employees away from duties characterized by rote, mind-numbing drudgery and instead assign them duties that can adequately leverage their subject matter expertise and creativity.
For example, SAS engaged with a large US health provider to build, deploy, and maintain a scalable end-to-end document processing solution to enhance, optimize, and modernize the customer's existing medical record review process. The existing process required skilled nurses and trained medical coding specialists to manually review thousands of pages of patient documentation to determine coverage eligibility.
The solution we deployed helped automate the processing of these lengthy service claims and surface the relevant information for the reviewer to then assess and confirm. The solution was operationalized in less than a year and currently processes upwards of ten million pages of documents per day. The resulting analysis has found that this solution enabled the customer to redirect the efforts of more than 350 full-time equivalent (FTE) employees while maintaining or exceeding the quality audit score of human reviewers.
LLM providers and users will also benefit as massive amounts of data, previously trapped in paper, PDFs, and dusty file cabinets are released to be incorporated into training data. With richer data sets, the resulting models should be more accurate and less prone to bias, which will benefit government end users.
When will document intelligence arrive?
IDP is here now but requires additional groundwork to be widely operationalized to generate value. Models such as ChatGPT, Claude and Llava are already capable of multimodal processing and reasoning, and more open-source models featuring similar capabilities are expected to be released soon.
However, these models are not silver bullets that can be deployed with a press of a button to solve an organization's existing workflow challenges. The challenging work needed to implement such models in a production setting still needs to be done.
This includes the less sexy (but just as vital) dependencies, such as understanding existing workflow processes, translating business logic into technical requirements, setting up data processing pipelines, ensuring data integrity, and building appropriate guardrails, feedback loops, and monitoring practices to meet the newly released governmental regulations surrounding the usage of AI models.
This is the necessary groundwork that must be addressed before an organization can realize any concrete ROI from such data science and AI efforts. At SAS, we are creating modular and industry-focused document analysis models.
The first release will focus on granting data scientists, analysts, and business users the ability to deploy end-to-end cloud-based OCR processing pipelines in an intuitive and easy-to-use manner. Additional, domain-focused functionality will follow.
Unlocking the past in the present to empower the future
It is difficult to overstate the need for this technology and its potential benefits. We've already shown the immense jumps in efficiency document vision approaches can yield. It can resolve information backlogs going back decades and empty massive warehouses of old files. New data can then be analyzed for decision-making and fed into data-hungry LLMs where appropriate. The technology is sophisticated enough to decipher poor-quality documents, copies of copies, and even handwritten doctor's notes from World War II medical records.
The technology exists and is only getting better. It is now up to businesses and government agencies to understand how such technology can augment their existing business processes. While document processing might not capture headlines nearly as much as other AI-related developments, document intelligence is poised for its own “LLM moment.” It promises much greater ROI than the first wave of generative AI delivered.