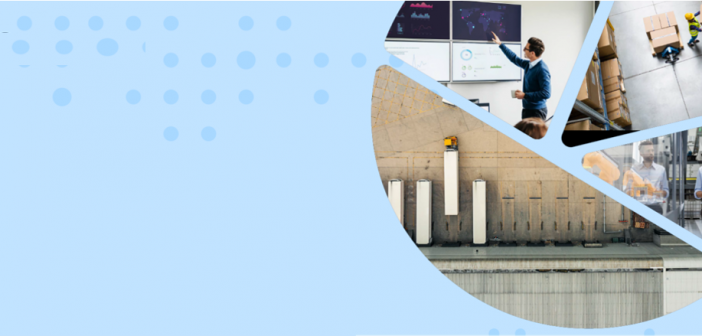
The social and economic impact of COVID-19 has dramatically affected supply chains and demand planning across all industries. Then there’s the Amazon effect, which has led to sky-high consumer expectations of the ordering and delivery process. Demand planners for retailers and consumer goods companies have quickly realized they have no