Thanks to COVID-19, companies have experienced how challenging it can be to plan and maneuver their supply chains around an unforeseen disruption. While the pandemic was a once-in-a-lifetime event (we hope), the unfortunate truth is that less severe events have overwhelmed or undermined demand and supply planning in the past -- and will likely continue to do so.
The biggest learning from this disruption? Legacy ERP (enterprise resource planning) systems are no longer up to the task of getting supply where it's needed in a timely and cost-effective manner. To achieve accurate forecasting and effective demand planning in the year ahead, companies must overcome the following four challenges, which we can tackle with cloud-native solutions.
1.Extraneous information and data
Sudden fluctuations in demand have been causing havoc with supply chains long before COVID-19. Companies using static tools to generate forecasts, such as spreadsheets or legacy ERP systems, are struggling to adapt to the new digital economy, and even moreso for unforeseen disruptions. These decades-old systems rely primarily on historical shipment data, which is not the most relevant information when it comes to understanding the future -- particularly when unforeseen events interfere with historical trends and seasonality. When a shift in demand occurs, the forecast becomes useless and the company faces shortages or surpluses of inventory.
Forecasts and plans are never 100% accurate 100% of the time. But forecasts can be made more accurate if a company has the capability to capture and manage current data in real time. Historical data alone is no longer enough. Companies need to access all the factors that are influencing demand, including market changes, economic changes, shifts from brick-and-mortar to eCommerce, and other leading indicators to better predict the impact of disruptive events. Effective demand planning and forecasting technology using predictive analytics and real-time insights allows companies to react faster, allowing them to streamline sourcing and production planning.
2.Siloed vertical processes
Rapid and sudden socioeconomic shifts due to COVID-19 have disrupted global supply chains and markets, forcing businesses to shift their operating models and revise their sourcing strategies. Events like this emphasize the critical importance of timely, accurate forecasting and demand planning.
The COVID-19 pandemic has, without a doubt, highlighted the gaps in these processes. To make matters worse, the siloed planning approach makes it impossible to forecast demand accurately. Having demand planners reside in the supply chain organization focusing mainly on shipments (supply) does not allow them to react quickly to shifting consumer demand. Nor do they have access to those market, economic and commercial factors influencing demand, as they are too far removed from the consumer. They need to be downstream, closer to the market and the consumer programming that's used to influence consumers to purchase their products.
3.Traditional analytics no longer work
Short-term demand sensing requires the analysis of massive volumes of data. It's impossible if you’re using on-premise legacy ERP systems designed in the 1990s and early 2000s using classic server technology and desktop platforms to implement demand sensing. When data reaches a certain scale, all these systems and applications break down very quickly. The capabilities of the human brain, while impressive, are not built to analyze and make sense of huge data sets streaming in from multiple sources (devices embedded everywhere, IoT, online, mobile, etc.), necessitating modern tools to present this data in a way we can easily understand.
The availability of data has exploded over the past five years and needs to be analyzed to achieve meaningful insights for demand forecasting and planning. New technology solutions such as cloud-native demand planning have been gaining a lot of attention as they can handle big data. These modern cloud-native technologies and applications are not inhibited by the task of analyzing large amounts of data in real-time using predictive analytics and machine learning.
4.Poor execution of new product launches
Product life cycles have grown shorter and more difficult to predict, presenting new challenges to demand forecasting and planning processes. New product forecasting remains a challenge because they lack historical data, which can easily lead to mistakes in demand forecasting and planning. However, many new product introductions are variations of existing versions, such as packaging upgrades and new colors or flavors that can be easily automated and forecast using machine learning.
New technology can easily attach older product history to forecast new, similar products using a technique called ‘product chaining’, or phase in/out. An effective demand forecasting and planning system can also utilize machine learning and data mining to mine data across the entire product portfolio for existing product lines to find analogous, or “as like” products to forecast new products that are line extensions (new flavor, package size, and others). Once the initial forecast is developed, these advanced applications can apply machine learning to predict the launch volumes and automatically layer in the forecasts into the appropriate brand and product group.
Why should you opt for cloud native forecasting solutions?
Commercial, operational and financial data integration is essential for successful forecast modeling and relies on the use of ERP software. For businesses to create detailed, accurate demand forecasts they need to be based on current and fully integrated commercial and transactional data. The cloud can eliminate the need for on-premise software, thus decreasing the cost of ownership, while providing a consolidated platform to integrate all data and attain a single source of truth for the company. A cloud-native solution where the software draws from data that’s stored in a centrally maintained enterprise data repository (or data lake) allows the demand forecasting and planning organization to create models that use the most up-to-date information. The end result is improved demand planning.
While spreadsheets have been popular for modeling, many organizations are now turning away from them. In addition to spreadsheets being ill-suited to managing large quantities of information, spreadsheets are difficult to use and prone to inaccuracy. This makes it problematic for companies to have confidence in their statistical forecast model, resulting in a weakened ability to make informed business decisions. A cloud-native modeling platform is easier to organize and manage than spreadsheets and provides greater governance and control over the numerous modeling scenarios that a business creates. The cloud also makes it easy to integrate commercial, operational and financial data, which increases accuracy and ensures forecasts are aligned with company goals.
Cloud-native demand forecasting and planning solutions incorporate artificial intelligence and machine learning that allow system algorithms to be tweaked dynamically. They also allow users to get under the hood of the solution to tweak forecasting models and modify data inputs, all of which helps improve the accuracy of the forecasts. In many cases, the models are automatically adjusted when applying machine learning models that learn overtime.
Another advantage: The cloud can be accessed from anywhere. With a cloud-based data repository, every department in an organization can constantly stay up to date with new data trends and marketplace activities while sharing information across the organization. Cloud-native modeling software offers additional collaborative features allowing users to leave comments for other users, increasing communication between departments through integrated control towers, dashboards, and reports. The software also retains all previous scenario and plan versions, so employees can refer to prior actions and assumptions as needed.
Managing models in the cloud is easier and more efficient than managing numerous spreadsheets. With the enhanced collaboration capabilities of the cloud, departments can reduce the time spent in meetings and improve office workflow. The cloud also makes it easier to scale models by enabling multi-dimensional modeling. It can adapt to scaling needs by seamlessly accommodating an increased number of users without procedural errors or additional licenses. The cloud can handle large volumes of data easily so as your data needs grow, storage capacity grows with it.
Cloud-native solutions can help to improve efficiency and increase the accuracy of statistical forecasting and demand planning. With cloud-native applications, companies can enjoy advanced modeling capabilities, increased collaboration, lower cost of ownership and fast deployment. The SaaS (Software as a Service) platform is highly scalable, so companies can increase their usage requirements without the need to purchase new technology. See Figure 1.
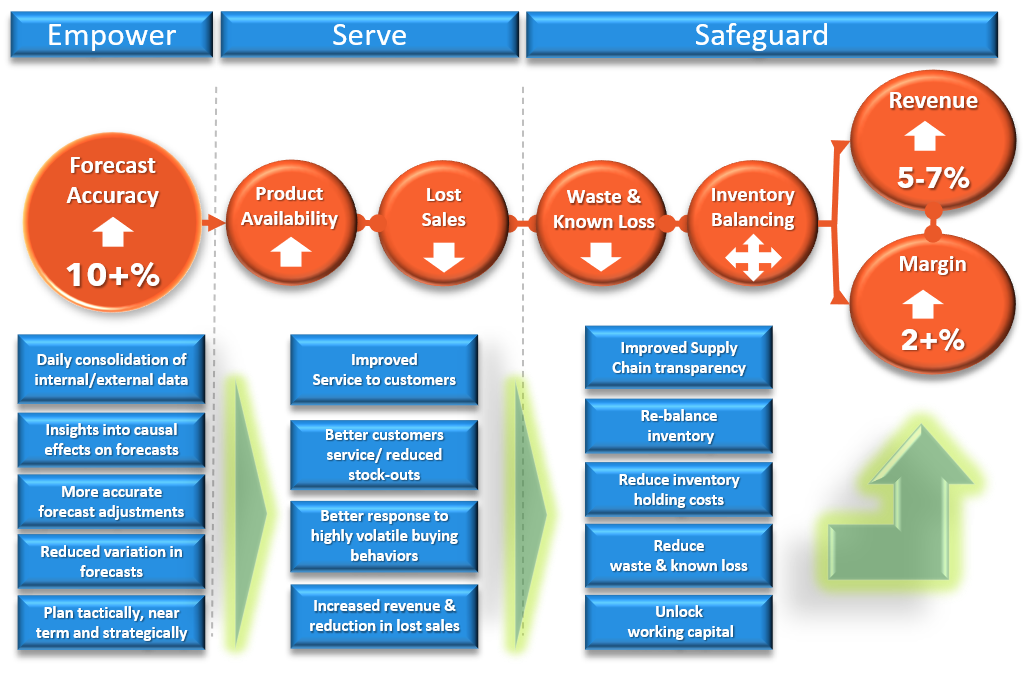
To learn more, download the free white paper: Using Advanced Analytics to Model, Predict, and Adapt to Changing Consumer Demand Patterns Affected by COVID-19.