Over the last year, generative AI has captivated the public imagination.
Many of us have become newly acquainted with the concept of an approaching Singularity coined by John von Neumann or Nick Bostrom’s Paper Clip thought experiment. Fortunately, Microsoft’s office assistant, Clippy, has yet to dutifully transform our planet into a heaping pile of paper clips. Nor has generative AI managed to improve itself to a point of surpassing human intelligence.
Apocalyptic predictions aside, generative AI does hold the potential to cause harm, and these types of danger fall into different categories. Well-intentioned people can create processes that accidentally harm individuals by failing to be transparent, invading privacy or introducing implicit bias.
In a previous article, we discussed how generative AI in the wrong hands can be used to create supporting medical documentation for services that never occurred. This can happen in clinical notes, medical imaging, beneficiary verification of service letters and more.
The good news is that none of these capabilities represents entirely new behaviors unseen by health care payers. Rather, bad actors can utilize the strengths of generative AI to increase the efficiency and sophistication of previously known schemes. In other words, this new technology can accelerate fraud, allowing for increased volumes of fraudulent claims submissions with fewer human resources. Health care payers will need to respond in kind.
Special investigation units (SIU) and payment integrity (PI) professionals are crucial in countering these challenges. SIU investigates and uncovers fraudulent claims, while PI ensures payment accuracy and compliance.
Instead of reinventing the wheel, using generative AI can enhance the efficiency of SIU and PI teams. Here are three examples of how these teams can use generative AI to fight back.
1. What if you had a digital assistant for claims?
One of the unspoken “joys” of health care fraud fighting is the iterative dance of requesting and using claims data. A familiar scenario for many goes like this: An investigator requests claims data for a provider from an analyst. The analyst asks questions about the parameters of the data pull. The investigator provides a narrow set of procedure codes, diagnosis codes, timeframes, etc. The analyst pulls the claims, and upon the investigator’s review, a new insight requires the data to be pulled again but with one more column. Rinse and repeat ad infinitum.
Imagine having a digital assistant plugged into the claims data, allowing non-technical users to pull their data. An investigator could type in plain English, “Pull claims data for Dr. Greenthumb with these procedures and diagnoses between these dates of service.” The digital assistant would even alert the user of multiple provider identifiers and check definitions of procedures to ask, “Would you like to include all of these codes?”. A few rounds of this may take minutes rather than days or weeks of data exchanges between multiple people.
A non-technical user could also run a fundamental descriptive analysis of returned claims. One could ask for the most prevalent combination of diagnoses assigned by the provider anytime two specific procedure codes were billed on the same service date. Or the user can ask for the average number of days between dates of service if the frequency of visits is a concern. Extremely complex parameters and analysis would still require a technical expert, but many tasks could be handled without one.
2. Using generative AI for synthetic data generation
To put it mildly, training health care fraud models is complex. Among many other reasons, a major roadblock is that while you have thousands or millions of non-fraud claims, you may only have a few examples of fraudulent claims for your algorithms to learn from. This is known in the machine learning community as the class imbalance problem. One way to attempt to get around this is to create synthetic data.
Unfortunately, creating synthetic medical data is arguably even more difficult than building the models that would use it. Several factors contribute to this complexity, primarily the intricacy of medical data. Various sampling techniques exist to overcome this, including reducing non-fraud examples (under-sampling), duplicating existing fraud examples (oversampling), and creating new fraud examples similar to existing ones (synthetic data generation). The diagram below puts this into perspective.
Generative AI excels in the realm of synthetic data generation and stands out because rather than performing tasks like classifying and predicting, its output generates more data. That is why it can create text strings, images, audio, etc. The potential of generative AI lies in its ability to find nuanced patterns and generate more data to look similar to those fraud examples. It is hoped that new techniques will surpass previous methods for synthetic data generation for the class imbalance problem.
3. What about a case management assistant?
Analyzing behavior patterns in data and carrying out investigations is only one piece of the fraud-fighting puzzle. Case management is another critical element. It involves documenting investigative actions and task assignments, drafting communications, tracking ROI and creating additional intel by linking common actors and behaviors across multiple investigations. Most of these actions are repeatable and hold the potential for partial automation.
Medical fraud investigations come in various forms, each with unique nuances and requirements. The questions about nerve conduction study administration will vastly differ from questions about durable medical equipment. A digital assistant trained for investigations can recommend questions for specific scenarios. Similar to integrating a digital assistant or chatbot with claims data, incorporating one into a case management system can be valuable to support investigators and investigative managers in their day-to-day tasks. When an investigator initiates a case, the first step often involves reviewing notes and determining case status. A digital case management assistant can swiftly compile a case summary or answer a specific question about the case. Also, it could recommend the next steps based on the provided information.
Furthermore, this assistant could mine investigative case notes for additional intel. In most case management systems, there are forms to enter demographic identifiers about subjects of interest, including names, addresses, phone numbers, license information, etc. There is the possibility that an investigator calls a phone number to reach a provider. Still, this number is only entered into the investigative narrative rather than a form, meaning it would not be used as structured data to inform analytics. An assistant could pull that number from the note and add it as usable structured data.
The accelerating role of generative AI in fraud prevention
It shouldn’t surprise anyone that generative AI is quickly becoming a potent tool. There are numerous ways existing generative AI capabilities can help payers beat the rising tide of fraud; only some are described above.
Over time, we can count on introducing more generative AI in the fraud space. Technology will continue to enhance the ability to commit fraud and fight it. Payers will need to continue to evolve strategies for detection and improve the efficiency of conducting investigations. Now is the time to use generative AI in the fight against fraud.
Explore more topics related to International Fraud Awareness Week
Jason DiNovi and Tom Wriggins contributed to this article
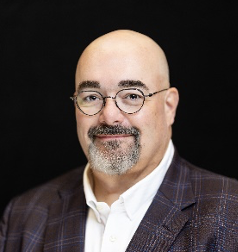
With over 30 years of health care experience, Tom Wriggins brings practitioner-level expertise to his role as a Senior Manager with SAS. Wriggins combines extensive clinical experience with data and analytics knowledge to help government and commercial health care entities crack down on fraud and improper payments. He has led multidisciplinary teams that have delivered large and complex data solutions for government health agencies, and has created fraud and abuse investigative training programs.