Getting ahead of an equipment failure can be the difference between millions of dollars saved, and disastrous losses. Predictive maintenance, which uses data and analytics to determine the health of an asset to predict the best time to perform maintenance, is now easier than ever if implemented with a smart, deliberate approach.
Predictive maintenance isn’t new – for decades, we have assessed the integrity of machines and systems through measurements taken during periodic inspections. What has changed is that with increased connectivity in our devices and more accurate sensors, applying advanced analytics to larger sets of more accurate data vastly improves predictions about the interventions that will be the most effective.
At the recent IoT Slam, which SAS had the honor of hosting, I moderated a panel that included representatives from Lockheed Martin and Georgia-Pacific, as well as IoT and analytics experts from SAS.
The panelists shared powerful examples of value delivered by predictive maintenance programs and lessons learned from real experiences implementing these efforts. We discussed how scheduled downtime in manufacturing and supply chain logistics is much less costly than halting production or distribution while waiting for the right components to repair a critical asset. When these companies relied on predictive maintenance programs, they spent less money on labor and supplies for repairs, had less unplanned downtime, and understood their operational processes better overall.
View the IoT Empowered Predictive Maintenance panel from IoT SlamHere are four key takeaways from the panel conversation that will benefit anyone considering predictive asset maintenance:
Know your goals
Every predictive maintenance program should be specific to the company or organization's needs. For an airplane manufacturer, the value of predictive maintenance might be a reduction in aircraft damage or vulnerability. At the same time, a telecommunications provider might aim to reduce the maintenance cost of replacing aging cables. Leading with specific and measurable goals based on your organization's needs will enable you to craft the most valuable program.
Start small then scale up and scale out
The potential impact of a predictive maintenance program is promising, but it would be difficult to launch a program at full scale immediately. The initial scale of your implementation should align with your organization’s experience with data science and the level of data collection and asset connectivity. By focusing on training models to anticipate specific, known maintenance issues, you can develop a proven program as a bedrock for more advanced insights as you scale up to a larger number of more complex assets being tracked and assessed or scale out with more models. The value of a program that has been trained to accurately predict two or three points of failure in an asset becomes much more apparent when you can reliably scale it up to entire fleets of trucks or forklifts.
Learn how Lockheed Martin reduces downtime with advanced analyticsKnow your data
Suppose your organization’s IoT implementation is currently sparse. In that case, you might think you won’t be able to collect enough useful data from your machines, vehicles, or processes to make real improvements to your maintenance program. But newer and more advanced solutions allow you to run models that can start small and factor in new equipment as your IoT capabilities grow.
On the other hand, with more granular data collected from advanced systems at high volumes, predictive maintenance programs ingest substantial amounts of data. If each of your assets is generating gigabytes of data by the hour, you might benefit from prioritizing data preparation and implementing machine learning algorithms that can clean data that might otherwise need to be reviewed manually before it’s usable.
Data science is for everyone
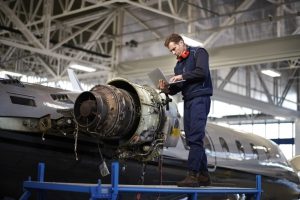
Data scientists are instrumental in handling these kinds of predictive models — but they’re not the only people on your team who can draw useful information from them. Thanks to powerful and user-friendly interfaces on new analytics tools, any team member has the potential to generate valuable insights from predictive maintenance programs. SAS Asset Performance Analytics has a powerful yet streamlined point-and-click interface with rich, interactive analyses that anyone can use. With tools like SAS Event Stream Processing, the people on your team can take on data science themselves, drawing on trends and insights from the program as well as their own knowledge with the equipment.
The value of predictive asset maintenance will differ depending on your organization’s specific needs and level of automation and IoT advancement — but it only takes a few insights at the start of the process to realize substantial returns on investment. Advanced asset performance analytics solutions reveal hidden patterns in your data, identify potential issues and enable corrective actions before disaster strikes.
Bryan Saunders, Head of Industry Consulting, SAS IoT has worked closely with Volvo Truck, which has had great results from its predictive maintenance efforts.
“Volvo was looking at the individual operating profiles of vehicles, and by assessing that they were able to better plan and predict maintenance activities across the repair network. That led to a 70% reduction in diagnostic time, and a 25% reduction in repair time,” said Saunders in his panel remarks. “So that certainly drove quite a bit of benefit to Volvo in terms of their resource efficiency and utilization, but also tremendous value to their customers.”
Learn how SAS powers remote diagnostics for Volvo TrucksPredictive maintenance ensures high-capital assets can run at maximum efficiency and achieve greater production targets, reduce operating costs, and minimize environmental, health and safety risks. The benefits range from preventing days of costly unplanned manufacturing downtime to saving lives by addressing vulnerabilities in vehicles or aircraft that could cause them to fail.
Now is the time to embark on a predictive maintenance journey. As Saunders explained, "There are so many new sensing packages and the analytics have caught up to really take advantage of those. It's just an exciting time. […] Start with value, but be creative in how you solve those problems because there's a wealth of technology at your disposal."