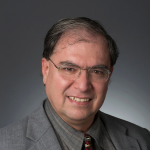
What’s new and what’s on the horizon for machine learning and analytics? We asked Kirk Borne, Principal Data Scientist and Executive Advisor at Booz Allen Hamilton, what machine learning technologies he’s watching. He focused his reply on applications, not algorithms.
“When I think about what’s new and coming up, I don’t expect that it’s the mathematics that will be changing but the types of things we can do with the math,” he says. With that in mind, consider these three applications of analytics that Borne is watching.
-
Graph analysis finds connections
Graph analysis is what we used to refer to as social network analysis, but then Facebook and Twitter came along and the term “social networks” took on a whole new meaning. Here's a broad explanation from Borne.
“Just about everything in the world has some relationship to something else,” he says. “You have a family, and you work at a business. That business has employees, and it has customers. Looking at your connections alone, you can tell there’s all kinds of connectivity going on in the world.”
Graph analytics is the process of discovering patterns in these networks. Some of these patterns can be helpful in running a business or improving a process, and some patterns can detect crimes or other preventable behaviors.
“Crimes like money laundering and insurance fraud are all perpetrated by networks of people who are laundering money or submitting insurance claims that might look legitimate on the surface, but aren’t,” explains Borne. “Understanding connections that are maybe two steps or three steps down the chain can help identify the bad actor or the person who is perpetrating the fraud.”
Graph analytics has positive and negatives applications. “Negative applications are the things we’re trying to prevent, and the positive applications are the things we’re trying to produce, like improved healthcare, improved education, improved transportation or improved energy use,” says Borne. “Understanding how things connect and relate to one another is all built around that sort of graph model, where you discover how A is linked to B, and B to C, and C to D in our world.”
Learn more about fighting fraud with graph analysis (also known as network analysis).
-
Geospatial analysis adds new dimension to analytics
The second application of machine learning to watch in the future, according to Borne, is geospatial analysis.
“Everything we do takes place in time and space. And so the tools we’re building, in terms of visualization and prediction, ought to take the contextual information of space and time into account,” says Borne.
For example, do patterns of behavior change based upon time and location? Are you more likely to purchase items on Fridays? And does your location influence your buying habits, your health decisions, your energy usage, your interests, your entertainment choices, your online preferences and so on?
“Building models that have that sort of spatial and temporal dimension to them is becoming more common. When you add those aspects of data and algorithms, it’s dealing with really complex data.”
For more on this topic, read, Location Analytics: Why Adding “Where” Makes BI Better.
-
Natural language generation can create and tell your data’s story
Natural language generation has become commonplace through virtual assistants on our phones and in our homes that can interpret our voice commands and reply in the same language.
Taking that capability a step further, Borne explains how NLG techniques can be used to help generate reports.
“In many cases, the report does not yet exist but the database exists. Natural language generation can automatically generate a written report for you from the database. Based on your voice prompt, the system finds the patterns, trends, anomalies, and gaps and generates a brand new report from the data,” explains Borne.
As data volumes continue to grow, and personal assistants become the norm, technologies that can help us interact naturally with data will become even more popular.
“If we can have a chat bot tell us the latest news, the stock market and all the financial numbers, the next step is for it to tell you the story behind the numbers in an interpretable way,” says Borne. “I think that’s really valuable.”
Learn more about natural language generation in the article, Your Personal Data Scientist.
What machine learning technologies are you watching, and how do you see machine learning changing the future? Tell us in the comments.
1 Comment
Excellent report Alison. For me in the region that we are catering to exactly these specific problems in the market. I would love to see if you have more content in this regard.
Always a pleasure to read this content.