Companies have become obsessed with product quality – and for good reason. Customer dynamics, their willingness to expose product quality issues socially on the web, and the ease with which they can jump to competitive products make quality a more important differentiator than ever before.
Over the past 15 years, I have had the opportunity to work with many organizations, across industries, to help them leverage analytics to improve the quality of their products and services. With such high stakes, it is always a bit of a surprise to hear how exposed many companies are when it comes to detecting and correcting product defects in the field. For too many, they are entirely reliant on basic business intelligence reports and Excel. They know when a claim occurs and can slice and dice claim counts to, at best, infer the existence of a possible defect. The result is they spend more time chasing false signals, while real defects continue to plague their customers.
Time is money – and brand value. The longer issues linger, the more they cost the manufacturer, and the more negative pressure is piled on the brand. Advanced analytics can drive significant value by helping organizations greatly reduce the time and effort involved in identifying and resolving product defects that make it to the field. Here are three ways analytics can drive value in reducing the impact of product defects:
- Early Warning (issue detection): The sooner a company is alerted to an issue, the sooner they can resolve it. Analytics can look across millions of combinations of production periods, time in service, and time of claim/event and detect significant changes in behavior, beyond normal variation. These analytics are not fooled by changes in sales volume, or seasonality. Automating these analytics makes them even stronger, allowing the system to identify the issues and notify the right person.
- Prioritization: Analytics can help companies understand what the impact of an issue is today versus 30 – 60 – 90 days from now, to better allocate finite engineering resources on the right issues at the right time.
- Issue Definition: Data driven root cause analysis can take advantage of a wide range of techniques, from simple trends to exposure to decision trees and more. Univariate, multivariate, structured, textual – all can be leveraged by engineers, not just statisticians, to provide understanding into the combination of attributes that are driving a defect.
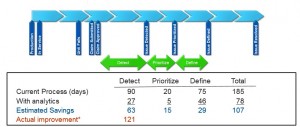
SAS conducted a study to measure individual company’s ability to detect, prioritize, and define product defects based on warranty data. To start, very few companies really try to measure these metrics. Through a standardized process, SAS was able to accumulate metrics from dozens of companies and the findings where striking. Through the study, companies determined that analytics could drive an average of 107 days out of their issue detection to correction cycle. What was most telling was that when SAS took their data and ran it through their early warning engine, it took 121 out of that process alone!
How well are you protecting your customer and managing your warranty costs? The cost of failure – each failure – is going up.