Today in manufacturing there has been a lot of investment in automation, supervisory controls, quality, and execution systems. The amount of data produced and now being captured is staggering. The data captured in industry will re-define what is “big” in big data.
Yet, for all this investment:
- Equipment still fails.
- Scrap is still produced.
- Safety incidents still occur.
Therefore, there are things about manufacturing processes we still don’t know.
The machines have been computerized and safety systems installed. The data from those computers are captured by SCADA systems, graphically represented, trended, alarmed, and stored into a data repository (typically a data historian). Quality control systems like SPC have been applied to these processes yet bad product makes its way into customers’ hands. Through Manufacturing Execution and Intelligence Systems the manufacturing process has been digitized. Operations have been digitally represented from raw materials to work in process to finished goods. MES takes the orders from the business system, applies finite scheduling algorithms, dispatches the work orders, tracks material to product and process specification, manages genealogy and yet we produce bad product. Manufacturing Intelligence tells us how we are doing at executing the process, how our equipment operates (OEE), the quality produced (yield), and how products were made and still we produce bad product.
Through all this investment we have “digitized” our manufacturing process with many variations of context from graphical to quality. We have modeled all we “know” about these manufacturing processes. How do we find out what we don’t know?
Industrial analytics
Analytics, or to be more precise, industrial analytics is the next phase for productivity improvement in manufacturing. We have all the data, in fact, more data than humanly possible to analyze. Industrial data has a lot of information locked within it, unknown correlations that lead to quality, safety, and equipment issues. Analytics will allow us to discover these correlations to not only understand what happened, but why it happened. Once we ask the question of why (diagnostics) something happened we can start to work on how to manage it and mitigate its affect going forward.
Analytics will buy time for the manufacturers. Once failure mode has been diagnosed, predictive analytics can build a model which is then wired up to live streaming sensor data. These models have the ability to recognize patterns in the data that will warn well in advance giving manufacturer’s critical time to “proactively” deal with the situation and mitigate if not eliminate the affects all together. This can have a huge effect on:
Quality:
- In process.
- Finished goods.
- Shipped product.
Asset Utilization:
- Uptime.
- OEE.
Safety:
- Controlled shutdowns.
- Eliminate unplanned downtime.
So what is holding up manufacturer’s from exploiting advanced analytics?
How many data scientist does it take to predict a failure?
Manufacturers are starting to leverage analytics to improve their operations but there have been obstacles in doing so. Let’s look at some of these:
Industrial big data
Throughout the history of manufacturing, data has always been available. Investments in controls, sensors and software have exploded the amount of data produced. Today, the problem isn’t getting the data, it’s having too much data. The next step many manufacturers have to do is put together a strong data management structure. Connect to all these data sources or “islands of data” much in the same way they connected their “islands of automation” with control networks. This data needs to be integrated, cleansed and made ready for analytics.
If you look at the example to the right, the first challenge is managing an industrial big data problem. Industrial data can take many forms but the one that dominates is the streaming sensor data coming off all the machines in industry, just a small slice of the Internet of Things. So in addition to the need to connect, integrate, and cleanse the data, manufacturers have to be able to store it, analyze it, and get meaningful answers in a reasonable time.
I have seen companies start to address streaming data on the manufacturing side of their business and attempt to throw all this data into a Hadoop cluster. However, they haven’t thought through what they would do once it got in there. Their desktop analytics tools were not designed to handle such volumes of data. Therefore, when industrial companies look at solving their big data problem they need to consider the end game and how they will derive the value from the data they are attempting to store.
Approachable Analytics
The second issue is the ability to use advanced analytics. Manufacturing organizations have outsourced much of their engineering capabilities from the plants to service providers. So where will they get the expertise to use theses analytics? Manufacturers need to be able to take advantage of advanced technology like analytics but don’t have the resources to learn it.
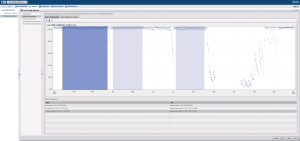
Fortunately, today the field of analytics is progressing and eliminating the need to have a PhD in math. Visual front ends allow engineers to graphically build analytic models and deploy them. These models can give them alerts to deviations that will lead to quality issues or machine disruptions. This simplified method greatly widens the pool of talent that is capable of deploying analytics into manufacturing. Complex models can be operationalized and leveraged by the rest of the organization realizing the value analytics can give them.
This will allow a manufacturer to progress in their maturity of use of analytics. As adoption grows they can gain competitive advantage in several ways. They can increase profit margins by minimizing waste and rework. Capacity can be reclaimed by eliminating unplanned downtime leading to a more predictable manufacturing environment. Finally, manufacturers can use analytics to protect their brand and turn their customers into advocates in addition to repeat buyers.
Conclusion
Manufacturing has evolved over the decades since Ford invented the assembly line. Computers have revolutionized how we automated tasks and software allowed us to capture and add context to the data produced from operations. Today’s manufacturer is pressed to make significant gains as their mix of products explode due to consumer demand. Advanced analytics is that next level of productivity. They need to carefully consider the analytics platform they choose. This platform needs to be able to handle Industrial Big Data and be usable by the manufacturing practitioner without the need of data modelers or scientist.