The DO Loop
Statistical programming in SAS with an emphasis on SAS/IML programs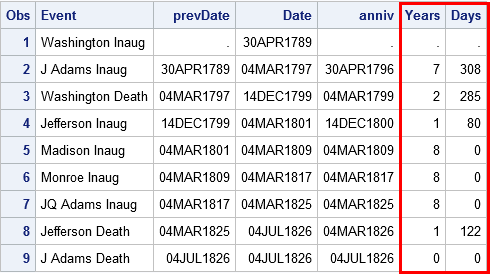
Last week I showed a timeline of living US presidents. The number of living presidents is constant during the time interval between inaugurations and deaths of presidents. The data was taken from a Wikipedia table (shown below) that shows the number of years and days between events. This article shows
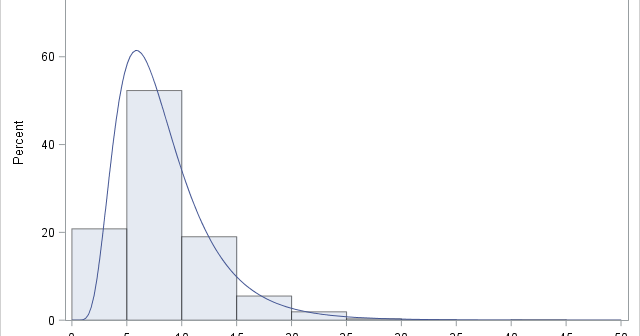
A SAS customer asked how to simulate data from a three-parameter lognormal distribution as specified in the PROC UNIVARIATE documentation. In particular, he wanted to incorporate a threshold parameter into the simulation. Simulating lognormal data is easy if you remember an important fact: if X is lognormally distributed, then Y=log(X)
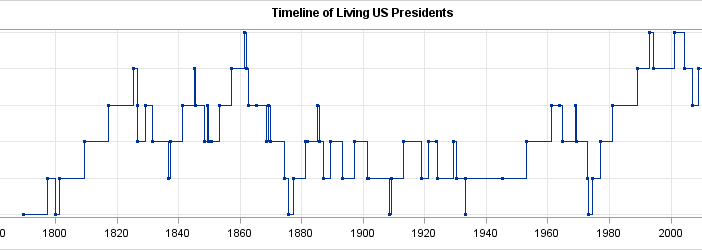
Quick! What is the next term in the numerical sequence 1, 2, 1, 2, 3, 4, 5, 4, 3, 4, ...? If you said '3', then you must be an American history expert, because that sequence represents the number of living US presidents beginning with Washington's inauguration on 30APR1789 and
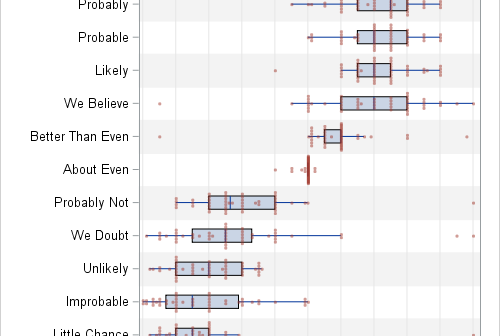
If a financial analyst says it is "likely" that a company will be profitable next year, what probability would you ascribe to that statement? If an intelligence report claims that there is "little chance" of a terrorist attack against an embassy, should the ambassador interpret this as a one-in-a-hundred chance,
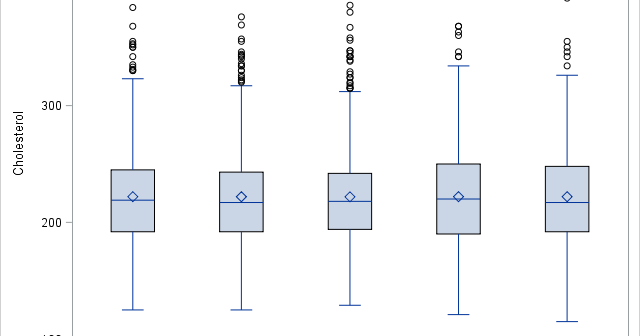
A frequently asked question on SAS discussion forums concerns randomly assigning units (often patients in a study) to various experimental groups so that each group has approximately the same number of units. This basic problem is easily solved in SAS by using PROC SURVEYSELECT or a DATA step program. A
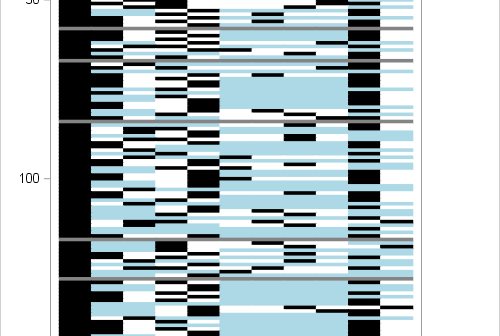
Most SAS regression procedures support a CLASS statement which internally generates dummy variables for categorical variables. I have previously described what dummy variables are and how are they used. I have also written about how to create design matrices that contain dummy variables in SAS, and in particular how to