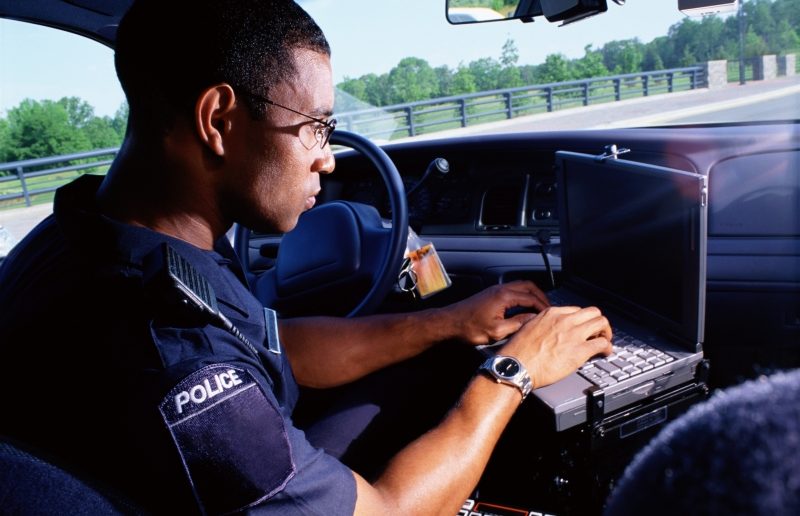
In my first post I looked at the role of analytics in policing and how analytics could and should be used to benefit modern policing. However, a key point that can be forgotten is analytics is only as good as the data it is based on. It’s vital to have access to a wide range of internal and external data assets that are carefully managed and ready for analytical processing.
Updated regularly
In my early days as a police analyst, we provided monthly reports to senior officers on crime figures, and within a couple of years these became daily dashboards. Modern-day analytical processes in banking and health care rely on real-time data that allows them to apply complex modelling to assess risk and make immediate decisions.
Modern manufacturing processes are using the Internet of Things to collect huge quantities of sensor data to inform decision making, preventing downtime and maintaining output. These decisions can be extremely important when tackling financial crime, saving lives and preventing lost revenue, saving organisations millions of pounds. What can policing learn from this? If policing is going to become more reliant on analytics and the insights it produces, then it must be able to do this quickly. Nightly refreshes of data warehouses and data stores may no longer be good enough. Data needs to be refreshed as frequently as is feasibly possible and reasonable for each data set. For example:
- Incident analysis should be in near-real time, allowing officers to make fast decisions regarding emerging trends.
- Analysis of body-worn video needs to be conducted in real time to assist officers – known as analytics at the edge.
- Call records need to be converted from voice to text in real time, allowing text analytics to immediately spot any common threads across emergency calls as they are happening.
- Social media data needs to be analysed in real time to spot emerging threats. It can be analysed more retrospectively when analysing a personal profile or tracking stolen goods.
- Analysis of crime data can afford to be slower, with crime data taking time to be manually entered and classified/reclassified.
Trusted
As we put more emphasis on the role of analytics in policing, we must ensure that decisions we make are based on trusted data. Data must be regularly assessed for completeness, uniqueness, validity, accuracy and consistency, then improved where necessary. Data quality checks and improvements can be carried out at multiple points during the data journey – e.g., as it’s entered into source systems or migrated to warehouses or data marts.
Data quality techniques such as standardisation and clustering should be used to ensure the data can be analysed easily and results maximised. These mechanisms can now be highly personalised by each organisation to develop bespoke processes that tackle their own specific quality issues and ensure data is processed for their needs. Police forces have been tackling data quality issues for many years, but they may have to revisit these processes to develop data that is a strong basis for analytics, rather than just meeting statutory requirements.
Police forces must develop data that is a strong basis for #analytics rather than just meeting statutory requirements. #CrimePrevention #DataQuality Click To TweetModern fraud analysis uses complex analytical processes to gather as much detail as possible from multiple sources, building a network of information about a person. Complex matching strings are created, allowing analysts to accurately identify and cluster duplicate records from across their data landscape. Police agencies can learn from these practices and expand their own deduplication routines to develop a true “golden nominal.”
Multiformat
No data is off limits – structured, unstructured, pictures or video – anything can now be used as the basis for analytical processing. For example:
- Enhanced text analytics processes are allowing us to extract entities, concepts, themes and sentiment from free text.
- Social media data is becoming increasingly available and should be used to drive decision making and combat crime.
- Images are increasingly being used as the basis for complex analytics using facial recognition and identifying cancerous tumours from scans.
- Video footage can be analysed in real time to monitor performance of football players. Can these techniques be used to analyse body-worn video footage and spot violence triggers as they are happening?
Appropriately accessible
As we expand data available at an exponential rate, careful consideration must be given to ensure that the data is presented and available in the correct format. This often means walking a tightrope between providing enough data for extensive analysis whilst maintaining performance. “Big data” will provide data scientists with the necessary ammunition to do their jobs, but larger data sets mean a greater overhead on resources. Some customers are now using concepts like in-database processing to leverage the extensive processing powers of source databases with parallel processing of complex analytical processes across multiple nodes.
I have lost count of the number of times I have been asked, “What is the best mechanism to present analytical data?” And I have seen customers adopt a variety of approaches – from small, well-defined data marts to huge Hadoop data lakes. Organisations must determine their own approach to this question, working within their own constraints and capabilities to develop a strategy that suits them.
Ready for analytics
Wherever appropriate, data should be prepared and made ready for analytics and modelling in advance to improve downstream performance. Preparation activities could include:
- Analytical partitioning – Splitting large data sets into smaller chunks that can be managed and accessed individually to optimise performance.
- Summarisation – Grouping and summarising large data sets to generate totals and subtotals of key variables for downstream processing. Using summary data sets where applicable, rather than granular data, will improve performance.
- Unique key addition – Adding unique keys to unstructured data to improve downstream performance of joins or modelling and enable processes like partitioning.
Analytics is nothing without data, and data scientists require access to a wide range of complex data to develop analytical processes and models that can inform decision making across policing. This means taking a pragmatic approach, with no data out of scope and using mechanisms for data quality and data preparation that generate data suited to analytics. These processes must also be easily adaptable to the changing requirements of the police force and the changing data landscape.