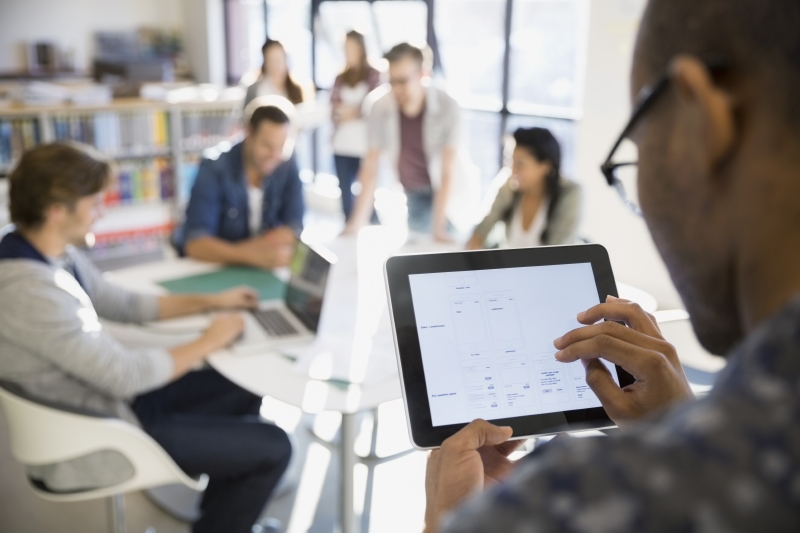
.@philsimon says don't treat data self-service as a binary.
.@philsimon says don't treat data self-service as a binary.
Welcome to the 1st practical step for tackling auto insurance fraud with analytics. It is obvious why our first stop relates with data, the idiom “the devil is in the details” can easily be applied in the insurance fraud sector as “the devil is in the data”. This article analyses
I'm a very fortunate woman. I have the privilege of working with some of the brightest people in the industry. But when it comes to data, everyone takes sides. Do you “govern” the use of all data, or do you let the analysts do what they want with the data to
I'm hard-pressed to think of a trendier yet more amorphous term today than analytics. It seems that every organization wants to take advantage of analytics, but few really are doing that – at least to the extent possible. This topic interests me quite a bit, and I hope to explore
Auditability and data quality are two of the most important demands on a data warehouse. Why? Because reliable data processes ensure the accuracy of your analytical applications and statistical reports. Using a standard data model enhances auditability and data quality of your data warehouse implementation for business analytics.
Trusted data is key to driving accurate reporting and analysis, and ultimately, making the right decision. SAS Data Quality and SAS Data Management are two offerings that help create a trusted, blended view of your data. Both contain DataFlux Data Management Studio, a key component in profiling, enriching monitoring, governing
In my first blog article I explained that many insurance companies have implemented a standard data model as base for their business analytics data warehouse (DWH) solutions. But why should a standard data model be more appropriate than an individual one designed especially for a certain insurance company?
A soccer fairy tale Imagine it's Soccer Saturday. You've got 10 kids and 10 loads of laundry – along with buried soccer jerseys – that you need to clean before the games begin. Oh, and you have two hours to do this. Fear not! You are a member of an advanced HOA
As I explained in Part 1 of this series, spelling my name wrong does bother me! However, life changes quickly at health insurance, healthcare and pharmaceutical companies. That said, taking unintegrated or cleansed data and propagating it to Hadoop may only help one issue. That would be the issue of getting the data
When you spend long enough writing and working in any industry, you inevitably see trends emerge and reach varying levels of maturity. Data governance is one such trend, as you can see from the following Google Trends chart:
I've been doing some investigation into Apache Spark, and I'm particularly intrigued by the concept of the resilient distributed dataset, or RDD. According to the Apache Spark website, an RDD is “a fault-tolerant collection of elements that can be operated on in parallel.” Two aspects of the RDD are particularly
In 2014, big data was on everyone’s mind. So in 2015, I expected to see data quality initiatives make a major shift toward big data. But I was surprised by a completely new requirement for data quality, which proves that the world is not all about big data – not
In a recent meeting, the CIO of a leading commercial automotive company’s shared his experience of high complexity in managing forecasting data. I was not surprised. Often demand planners complain about managing forecasting data. I can relate to where there are coming from. It’s due to the approach prescribed by their legacy
Integrating big data into existing data management processes and programs has become something of a siren call for organizations on the odyssey to become 21st century data-driven enterprises. To help save some lost time, this post offers a few tips for successful big data integration.
There is a time and a place for everything, but the time and place for data quality (DQ) in data integration (DI) efforts always seems like a thing everyone’s not quite sure about. I have previously blogged about the dangers of waiting until the middle of DI to consider, or become forced
The intersection of data governance and analytics doesn’t seem to get discussed as often as its intersection with data management, where data governance provides the guiding principles and context-specific policies that frame the processes and procedures of data management. The reason for this is not, as some may want to
We’ve been talking about data recently at the Analytic Hospitality Executive. I’ve advocated to use whatever data you have, big or small, to get started today on analytic initiatives that will help you avoid big data paralysis. In this blog, I’m going to get a bit more technical than usual
In recent years, we practitioners in the data management world have been pretty quick to conflate “data governance” with “data quality” and “metadata.” Many tools marketed under "data governance" have emerged – yet when you inspect their capabilities, you see that in many ways these tools largely encompass data validation and data standardization. Unfortunately, we
After acquiring personal IoT data in part 1 and cleaning it up in part 2 of this series, we are now ready to explore the data with SAS Visual Analytics. Let's see which answers we can find with the help of data visualization and analytics! I followed the general exploratory workflow
What data do you prepare to analysis? Where does that data come from in the enterprise? Hopefully, by answering these questions, we can understand what is required to supply data for an analytics process. Data preparation is the act of cleansing (or not) the data required to meet the business
As part of two of our client engagements, we have been tasked with providing guidance on an analytics environment platform strategy. More concretely, the goal is to assess the systems that currently compose the “data warehouse environment” and determine what the considerations are for determining the optimal platforms to support
Data Management has been the foundational building block supporting major business analytics initiatives from day one. Not only is it highly relevant, it is absolutely critical to the success of all business analytics projects. Emerging big data platforms such as Hadoop and in-memory databases are disrupting traditional data architecture in
In my previous post, I talked about how the Internet of Things promises new ways to use sensor and machine data by creating a highly efficient world that demands constant analysis and evaluation of the state of events across everything that surrounds us. I have also explained why it is
As this is the week of Christmas, many, myself included, have Christmas songs stuck in their head. One of these jolly jingles is Santa Claus Is Coming To Town, which includes the line: “He knows if you’ve been bad or good, so be good for goodness sake!” The lyric is a
The healthcare big data revolution has only just begun. Current efforts percolating around the country primarily surround aggregation of clinical electronic health records (EHRs) & administrative healthcare claims. These healthcare big data initiatives are gaining traction and could produce exciting enhancements to the effectiveness and efficiency of the US healthcare
Sometimes you have to get small to win big. SAS Data Management breaks solution capabilities into smaller chunks – and deploys services as needed – to help customers reduce their total cost of ownership. SAS Master Data Management (MDM) is also a pioneer in "phased MDM." It's built on top of a data
In my previous post, I outlined the main components needed for a phased approach to MDM. Now, let's talk about some of the other issues around approaching MDM: data governance and the move to enterprise MDM. Where does governance come in? Throughout your MDM program, it's important that deep expertise
For decades, data quality experts have been telling us poor quality is bad for our data, bad for our decisions, bad for our business and just plain all around bad, bad, bad – did I already mention it’s bad? So why does poor data quality continue to exist and persist?
Last time we explored consumption and usability as an alternative approach to data governance. In that framework, data stewards can measure the quality of the data and alert users about potential risks of using the results, but are prevented from changing the data. In this post we can look at
Data is everywhere,and getting to and managing that information is vital for accurate reporting, analysis and proactive decision making. This brings us to Best Practice # 3: Identify and Integrate Authoritative, Trusted Data Sources. As you might remember, these tips all come from my interviews with SAS education customers. From Best