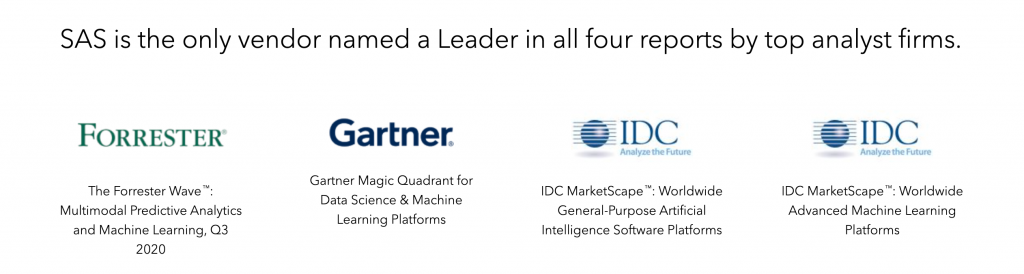
There are a plethora of commercially available data science platforms in the market, but guidance as to how to create a shortlist for consideration during the buying cycle is often lacking. That’s when it becomes time to turn to analyst firms for help in researching data science platforms - they do all the hard work for you!
An analyst report offers an unbiased, side-by-side, third-party evaluation of the technology in the market. These analysts know how to put the vendors through the paces and require proof of any claims that are made. Interacting with analysts is hard work on both sides as they require completion of an extensive RFI that makes my Ph.D. look like child’s play. They also require demos to validate what was said in the RFI and external points of view such as commentary from customers.
Below are summaries of 4 key analyst reports that I think should be on the reading list of data scientists and AI/Analytics leaders who are considering the adoption of a commercially available data science platform.
Let’s unpack each report.
1. 2020 Gartner Magic Quadrant for Data Science & Machine Learning Platforms
The key message in this report is that data science and machine learning platforms must support model operationalization in addition to model building. This is also known as ModelOps. Why is this so important? On average, only half the analytics models built ever make it into production. That’s right 50%! That can be disheartening to analytics teams who pour their time and energy into modeling only to never have those models see the light of day. In this report, SAS is acknowledged for its model operationalization and management platform which includes model performance monitoring, model governance, and lineage.
Furthermore, Gartner included vendors who could support a diverse analytics team such as expert data scientists who code their models (in SAS or open-source) in notebooks, citizen data scientists who prefer a visual drag and drop interface to construct pipelines, and others such as data engineers, developers, and machine learning engineers. SAS is also called out for ease of use for its automated capabilities such as AutoML and automated suggestions for data quality and data prep.
2. The Forrester Wave™: Multimodal Predictive Analytics and Machine Learning, Q3 2020
What stands out in this report is the inclusion of a scorecard comparing vendors on the current offering, strategy, and market presence. (I also like the reference to the mother lode of intelligence that models can infuse into processes and applications). Key messages in this report include augmenting data sciences via AutoML, ModelOps, and reviewing vendor roadmaps in terms of integration with other tools and technologies as well as the ability to serve ever-expanding analytics teams. SAS is praised for its AutoML, its guided analytics, and its support for open source programming languages such that they can take advantage of the powerful SAS engine.
3.IDC MarketScape™: Worldwide General-Purpose Artificial Intelligence Software Platforms
If you are just getting started with AI, then this would be a good report to read first. IDC offers advice for organizations just getting started with developing AI applications. This report includes a discussion of a platform’s ability to analyze both structured and unstructured data, which is important for AI capabilities such as NLP and computer vision. IDC also touches on knowledge representation and machine learning at a high level. IDC recognizes SAS for its ability to support the creation of models that use both structured data and NLP or computer vision. IDC also mentions SAS’ visual interface to better support non-data scientists with AI and machine learning.
4. IDC MarketScape™: Worldwide Advanced Machine Learning Platforms
This report gets into detail about the specific tasks that data scientists perform such as data access, data prep, feature engineering, building models, training models, tuning models, and deploying models. It also includes a thorough discussion of model management and model performance, topics that are getting more attention in recent years due to the need to deploy increasing more models into production (which is not an easy feat as mentioned previously). IDC recognizes SAS for supporting the end to end machine learning process with both a visual and programming interface which is appealing for those organizations who wish to develop an analytics team with differing skill sets, including data scientists, business analysts, and application developers who want to take advantage of REST APIs.
These four evaluations feature SAS Viya with SAS Visual Data Mining and Machine Learning and SAS Model Manager as the key products.
These reports are a great way to educate yourself about the trends, vendors in these spaces, and their product capabilities. So, sit back, get a cup of coffee, and start reading! Did I mention that these reports are free reading provided to you by SAS? See the links in the sidebar. No registration is required to get the reports. Happy reading!
1 Comment
Hey Susan - I don't actually see the links in the sidebar for these analytics reports.
https://blogs.sas.com/content/subconsciousmusings/2020/10/26/data-scientists-analyst-evaluations/
Is something missing? Looking forward to the articles!
Craig