Remember Subconscious Musings?
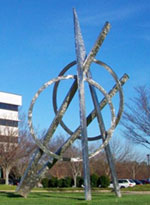
It was the name of the blog Radhika Kulkarni (now retired Vice President of SAS R&D) started in 2012. She wrote about trends that drove innovation and challenges that expanded the boundaries of what we thought was possible. It eventually evolved into what we now know as The SAS Data Science Blog.
Fast-forwarding to 2020, as the COVID-19 pandemic is paralyzing the world, many of the insights that once drove critical business decisions no longer apply. At the same time, organizations worldwide are moving to the cloud to innovate and to move faster toward their business goals.
The Analytics R&D team at SAS strives to empower and inspire using the most trusted analytics. Our customers see us as an integral, trusted, and innovative partner. We help them overcome complex data and analytics challenges on their digital transformation journeys.
Building on the original idea of Subconscious Musings, and as the new Vice President of Analytics R&D at SAS, I plan to regularly share stories, insights, and recent developments from the Analytics R&D team in this new blog series.
Meet Jan Chvosta
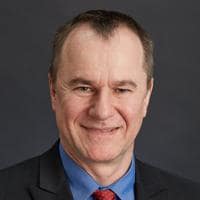
It is my great pleasure to kick off our new blog series with a discussion with Jan Chvosta. Today we will discuss the transformation of analytics. Jan is the head of Scientific Computing at SAS. He oversees Statistics, Econometrics, and Operations Research R&D.
Udo: In the last two decades, the area of analytics has undergone many changes. The transformation keeps accelerating, developing before our very eyes. New technology is a critical driver for advancements in methodology. Data are abundant in many areas. The cost of storing and analyzing data keeps going down. New technology and enhanced methodology enable us to compute and implement models that we could have only dreamed of two decades ago. How is your team coping with these challenges?
Jan: Actually, the transformation of analytics started a long time ago. While plenty of modeling and computational techniques are new to modern-day data analysis, some have been around for a very long time but have only recently become popular, or even possible.
One example is the Bayesian paradigm, named after the theorem discovered by Thomas Bayes some 250 years ago. The Bayesian paradigm offers clean solutions to a wide range of problems, but it stayed in the backwater of statistics for centuries. This was partially due to computational struggles in estimating the necessary distributions. However, with the arrival in the 1980s of affordable computing power, and also with the advent of Markov chain Monte Carlo (MCMC) methods, the Bayesian paradigm saw a renaissance and became a staple in countless application and research fields. With ever-increasing processing power, multicore CPUs, and distributed computing, new algorithms are improving our Bayesian modeling capabilities by leaps and bounds. A complex Bayesian model that took days to run 20 years ago can now be computed in a matter of seconds.
Scientific computing is not a thing of the past
Udo: Would you say that it is an exciting time to be working in the area of scientific computing?
Jan: Yes! We are presented daily with many great opportunities to push the frontier of data science forward and implement new methodology in our software. The landscape of data science is changing rapidly. It is hard to keep up with it all, but it is rewarding and can be a lot of fun. Many of us were trained as classical statisticians, econometricians, and operations research experts. In this new world driven by data and science, we have an opportunity to use our skills along with techniques coming from areas like computer science and machine and deep learning. Combining them all, we can create a powerful data science bundle that provides the right tools for an analyst in the 21st century.
Udo: Some people like to consider fields like statistics and econometrics as a thing of the past. But isn’t it true that a lot of the modern modeling techniques that have recently gained popularity stand on the shoulders of classical approaches?
Jan: A key starting point for much of modern data science is classical statistics. This includes descriptive methods such as clustering and principal components analysis as well as prediction methods such as linear, logistic, and generalized linear regression. These techniques were among the first that we worked on for SAS® Viya®, and they remain cornerstones of many applications. You combine them with different tools and techniques for other parts of the process, like handling missing values and scoring new data, or you use them many times in different ways, such as with model averaging. But still, in many ways, regressing a target variable on many potential predictors is what’s at the root of modern modeling.
Cloud computing accelerates transformation
Udo: SAS and Microsoft announced a deep technology partnership, stressing the importance of analytics moving to the cloud. What does cloud computing mean to you?
Jan: Moving to cloud computing presents a great opportunity to democratize analytics and introduce it to an even wider range of businesses and individual users. It used to be that data collection, processing, and modeling required a lot of dedicated and expensive resources. That is not necessarily the case today as technology and enhancements in methodology help us navigate through the process. Solid cloud analytics based on scientific research and principles makes a big difference. I believe that SAS provides a lot of value here.
Udo: Earlier, we mentioned that the cost of storing data keeps going down. At the same time, we have access to very powerful hardware and cloud infrastructure. Has this expanded the boundaries of what is possible?
Jan: Yes, in the initial stages of the transformation we were talking a lot about big data. Our efforts were concentrated on dealing with large volumes of data and processing it. Today our cloud infrastructure allows us to do that without any problems. Instead we can concentrate on what I call “big models.” Those are the models that we could not even have dreamed of a decade ago. They don’t necessarily have gigabytes of data associated with them, but they are very computationally demanding.
Big models ... don’t necessarily have gigabytes of data associated with them, but they are very computationally demanding.
Udo: There is no doubt that we are in the middle of a digital revolution that is impacting all areas of analytics. Researchers everywhere are trying to rethink the impossible and redefine their approaches. Can you lift the curtain a little bit and share some of the new exciting new developments your team is working on?
Jan: There is certainly no doubt that the digital revolution is happening, and it is interesting to note how quickly it has accelerated since the COVID-19 pandemic started. We have all had to adjust to a new normal that heavily relies on technology. The smooth transition to working from home wouldn’t have been possible without powerful cloud-based solutions for office productivity. Quick cloud transformation was also necessary to mitigate the impact of the virus as many businesses started to rely more heavily on digital channels to generate revenue. Powerful analytics also plays an important role in trying to control the pandemic.
We are proud to be part of efforts that helped develop solutions for our customers to understand the pandemic and predict its impact on their businesses. I also believe that all the investments to cloud infrastructure and solutions that our customers are making now will help them recover faster when the pandemic is over. We are doing all we can to support them on their cloud transformation journey by providing powerful analytics that they can rely on for their business needs.
Udo: Jan, many thanks for your time today and for sharing your insights.
Read more from Jan by ordering his book on econometricsThis is the first post in our series about statistics and analytics bringing peace of mind during the pandemic.
1 Comment
Pingback: Your recipe for unlimited capacity starts in the cloud - The SAS Data Science Blog