How do we hire data scientists at SAS, since we are not unique in our search for a rare talent type that continues to be in high demand? This post is the last in a series on finding data scientists, based on best practices at SAS and illustrated with some of our own “unicorns.” You can read my first blog post on calling them unicorns and for tips 1 and 2 on finding them in an MS in Analytics Program or from a great program you may not have heard of. You can read tips 3 and 4 on how to find this kind of talent outside the traditional STEM academic disciplines. And tips 5, 6, and 7 detail the value we’ve found in intern programs, social networks, and sponsorship of foreign nationals.
This last post focuses on less tangible aspects, related to curiosity, clarity about what kind of data scientist you need, and having appropriate expectations when you hire.
8. Look for people with curiosity and a desire to solve problems
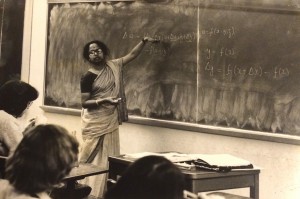
As I blogged previously, Greta Roberts of Talent Analytics will tell you that the top traits to look for when hiring analytical talent are curiosity, creativity, and discipline, based on a study her organization did of data scientists. It is important to discover if your candidates have these traits, because they are necessary elements to find a practical solution and separate candidates from those who may get lost in theory. My boss Radhika Kulkarni, the VP of Advanced Analytics R&D at SAS, self-identified this pattern when she arrived at Cornell to pursue a PhD in math. This realization prompted her to switch to operations research, which she felt would allow her to pursue investigating practical solutions to problems, which she preferred to more theoretical research.
That passion continues today, as you can hear Radhika describe in this video on moving the world with advanced analytics. She says “We are not creating algorithms in an ivory tower and throwing it over the fence and expecting that somebody will use it someday. We actually want to build these methods, these new procedures and functionality to solve our customers’ problems.” This kind of practicality is another key trait to evaluate in your job candidates, in order to avoid the pitfall of hires who are obsessed with finding the “perfect” solution. Often, as Voltaire observed, “Perfect is the enemy of good.” Many leaders of analytical teams struggle with data scientists who haven’t yet learned this lesson. Beating a good model to death for that last bit of lift leads to diminishing returns, something few organizations can afford in an ever-more competitive environment. As an executive customer recently commented during the SAS Analytics Customer Advisory Board meeting, there is an “ongoing imperative to speed up that leads to a bias toward action over analysis. 80% is good enough.”
9. Think about what kind of data scientist you need
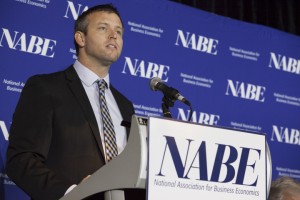
Ken Sanford describes himself as a talking geek, because he likes public speaking. And he's good at it. But not all data scientists share his passion and talent for communication. This preference may or may not matter, depending on the requirements of the role. As this Harvard Business Review blog post points out, the output of some data scientists will be to other data scientists or to machines. If that is the case, you may not care if the data scientist you hire can speak well or explain technical concepts to business people. In a large organization or one with a deep specialization, you may just need a machine learning geek and not a talking one! But many organizations don’t have that luxury. They need their data scientists to be able to communicate their results to broader audiences. If this latter scenario sounds like your world, then look for someone with at least the interest and aptitude, if not yet fully developed, to explain technical concepts to non-technical audiences. Training and experience can work wonders to polish the skills of someone with the raw talent to communicate, but don’t assume that all your hires must have this skill.
10. Don’t expect your unicorns to grow their horns overnight
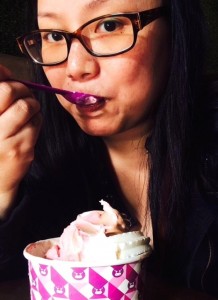
Annie Tjetjep relates development for data scientists to frozen yogurt, an analogy that illustrates how she shines as a quirky and creative thinker, in addition to working as an analytical consultant for SAS Australia. She regularly encounters customers looking for data scientists who have only chosen the title, without additional definition. She explains: “…potential employers who abide by the standard definitions of what a ‘data scientist’ is (basically equality on all dimensions) usually go into extended recruitment periods and almost always end up somewhat disappointed - whether immediately because they have to compromise on their vision or later on because they find the recruit to not be a good team player….We always talk in dimensions and checklists but has anyone thought of it as a cycle? Everyone enters the cycle at one dimension that they're innately strongest or trained for and further develop skills of the other dimensions as they progress through the cycle - like frozen yoghurt swirling and building in a cup.... Maybe this story sounds familiar... An educated statistician who picks up the programming then creativity (which I call confidence), which improves modelling, then business that then improves modelling and creativity, then communication that then improves modelling, creativity, business and programming, but then chooses to focus on communication, business, programming and/or modelling - none of which can be done credibly in Analytics without having the other dimensions. The strengths in the dimensions were never equally strong at any given time except when they knew nothing or a bit of everything - neither option being very effective - who would want one layer of froyo? People evolve unequally and it takes time to develop all skills and even once you develop them you may choose not to actively retain all of them.”
So perhaps you hire someone with their first layer of froyo in place and expect them to add layers over time. In other words, don't expect your data scientists to grow their unicorn horns overnight. You can build a great team if they have time to develop as Annie describes, but it is all about having appropriate expectations from the beginning.
To learn more, check out this series from SAS on data scientists, where you can read Patrick Hall's post on the importance of keeping the science in data science, interviews with data scientists, and more.
And if you want to check out what a talking geek sounds like, Ken will be speaking at a National Association of Business Economists event next week in Boston - Big Data Analytics at Work: New Tools for Corporate and Industry Economics. He'll share the stage with another talking geek, Patrick Hall, a SAS unicorn I wrote about it in my first post.
2 Comments
Hai,
The blog is very intersting,but one thing which is a big ice to break is that people only with experience are hired every where.Hoe does fresher start to gain a experience if he is not given an oppurtunity to work.Please do throw light in this issue.If there are any standardized training for freshers please do let us know.
Great tips Polly, can't wait to share with participants of my 21-day get "FIT" sas challenge to help job seekers find SAS work. https://www.linkedin.com/groups/SAS-Canada-Training-Career-Development-5095978/about