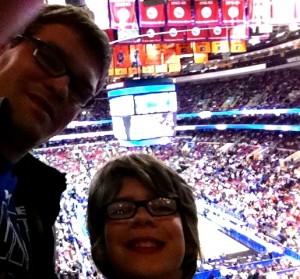
In my region of North Carolina (Raleigh, Durham, and Chapel Hill) one of the most anticipated times of the year has arrived— the NCAA basketball tournament. This is a great time of year for me, because I get to combine several of my passions.
For those who don’t live among crazed college basketball fans, the NCAA (National Collegiate Athletic Association) holds an annual tournament that seeds the regional conference winners and the best non-conference winning teams in a single elimination tournament of 68 teams to determine the national champion in collegiate basketball. The teams are ranked and seeded so that the perceived best teams don’t face each other until the later rounds.
In the tournament history stretching back more than 75 years, only 14 universities have won more than one championship, and three schools local to SAS world headquarters are on that list (the University of North Carolina, Duke University, and North Carolina State University). That concentration, combined with the fact that this area is a well-known cluster for statistics, means that I am not alone amongst my neighbors in combining my passions.
The NCAA tournament carries with it a tradition of office betting pools, where coworkers, families, and friends predict the outcome of the 67 games to earn money, pride, or both. Unbeknownst to many of them they are building predictive models, something near and dear to my heart. As a data miner, I analyze data and build predictive models about human behavior, machine failures, credit worthiness, and so on. But predictive modeling in the NCAA tournament can be as simple as choosing the winner by favorite color, most fierce mascot, or alphabetizing. Others rely on their observation of the teams throughout the regular season and conference championships to inform their decisions, and then they use their “gut” to pick a winner when they have little or no information about one of both of the teams.
I’m sure some readers have used these kinds of strategies and lost or maybe even won the “kitty” in these betting pools, but the best results will come using historical information to identify patterns in the data. For example, did you know that since 2008 the 12th seed has won 50% of the time against the 5th seed? Or that the 12th seed has beat the 5th seed more often than the 11th seed has beat the 6th seed?
Upon analyzing tournament data, patterns like these emerge about the tournament, specific teams (e.g. NC State University struggles to make free throws in the clutch), or certain conferences. To make the best predictions, use this quantitative information in conjunction with your own domain expertise, in this case about basketball.
Predictive modeling methodology generally comes from two groups: statisticians and computer scientists (who may take a more machine learning approach). The field of data mining encompasses both groups with the same aim - to make correct predictions of a future event. Common data mining techniques include logistic regression, decision trees, generalized linear models, support vector machines (SVM), neural networks, and many many more (all available in SAS).
While these techniques are applied to a broad range of problems, professors Jay Coleman and Mike DuMond have successfully used those from SAS to create their NCAA“ dance card,” a prediction of the winners that has had a 98% success rate over the last three years.
If you think you have superior basketball knowledge and analytical skills, then hopefully you entered the ultimate payday competition from Warren Buffett. He will pay you $1 billion if you can produce a perfect bracket. Before you go out and start ordering extravagant gift, it is worth considering that the odds of winning at random are 1 in 148 pentillion (148,000,000,000,000,000,000), but with some skill your odds could improve to 1 in 1 billion. In this new world of crowdsourcing, about 8,000 people have united to try and win the billion dollar prize. I don’t know how many picked Dayton to beat Ohio State last night, but that one game appears to have eliminated about 80% of participants.
If you’re looking for even more opportunities to combine basketball and predictive analytics, then check out this Kaggle contest with a smaller payday but better odds.
Statistician George Box is famous for saying, “essentially, all models are wrong but some are useful”. I wish you luck in your office pool, and if you beat the odds remember the bloggers in your life 🙂