When it comes to fraud detection and risk mitigation, predictive modeling has earned a reputation as the “heavy hitter” in the realm of data analytics. As our celebration of International Fraud Awareness Week continues, I would challenge our readers to ask themselves this question, “Is the reliance upon predictive analytics misguiding our ability to detect fraud? “
I did not come here to bury predictive modeling, of course. It’s superior to a rules-based approach since it moves away from mere intuition to data-driven decision making. Predictive modeling is highly effective in assessing risk since a predicted probability score helps scale risk from “somewhat likely” to “highly likely”. With that information, investigators can focus valuable resources on those events “most likely” to be truly fraudulent or non-compliant. And as seen by various Federal and State agencies, predictive modeling has had great impact when it comes to identifying and preventing fraud.
For instance, Los Angeles County Department of Public Social Services used predictive models to combat child care benefits fraud. LA County was able to identify organized conspiracy groups much earlier, significantly reducing the duration of fraudulent activities. LA County mapped out a network of participants and providers that visually displayed their relationships. They looked at whether any given small network fit into a larger scheme of networks, in which participants are in collusion with other child care providers. They identified strong central nodes and, in one case, found a child care provider serving many nodes of participants colluding in fraudulent activities.
That is all great, but with that said, since predictive models are built on known targeted historical events (i.e., known fraud), they can only predict well when scoring new data with similar relationships in place. As patterns of behavior change, predictive models become outdated very quickly and are not able to pick up on those new behaviors unless they are retrained with examples of what the “new behavior” looks like.
With this limitation in mind, I challenge readers to ask to what extent do we become reliant on outdated predictive models? Do we have the data to retrain those models? If not, does it make more sense to focus more effort on finding the “unknown unknowns?”
Imagine instead the ability to identify relationships without knowing which rule to write (rules-based) or relying upon known historical representations of what is bad (predictive modeling). These relationships would instead surface through contextual linkages and network patterns within the data. These type of analytics (network analytics or “entity link analysis”) would provide the contextual clues for detecting fraud by revealing changes in baseline behaviors, peer groups and other monitored entities and not rely upon known historical evidence of something bad.
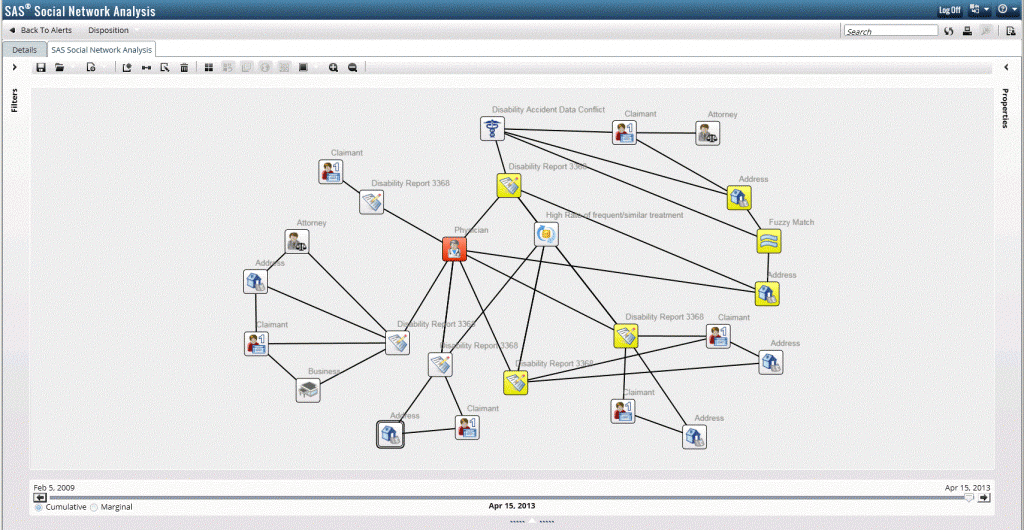
Outputs from the contextual linkages such as measures of centrality (for example, closeness and proximity), and changes in these measures over time, can then be used as inputs to help an existing model do a better job in detecting anomalies. They can also help a new model establish behavioral trends, and spot anomalous behaviors as compared to the behaviors of one’s peers. In other words, instead of using known historical evidence of fraud to train models, “membership” in a network becomes the target that is being modeled and someone is scored based on the extent they are moving “away” or “closer” to a type of behavior like collusion.
So in terms of fraud awareness, to what extent have you become reliant on predictive models while fraud behavior inevitably changes over time? And to what extent can you explore the territory of the “unknown unknowns”? How can you further enable your predictive models to detect new trends and open up new ways to predict fraud?