Data scarcity, privacy and bias are just a few reasons why synthetic data is becoming increasingly important.
In this Q&A, Brett Wujek, Senior Manager of Product Strategy at SAS, explains why synthetic data will redefine data management and speed up the production of AI and machine learning models while cutting costs and driving innovation.
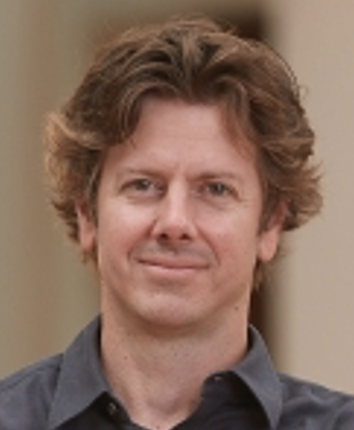
Q: How would you define synthetic data?
Brett Wujek: Synthetic data is data that is artificially generated. There are several different mechanisms for generating the data. You can provide statistical distribution information to sample from. You can define rules that encode business logic that describe how the value should be generated and how the attributes of your data should relate to each other. There are AI-based techniques that use algorithms to generate data based on patterns that are found in real data. So, there are several ways you can generate data to either supplement existing data or, in some cases, just completely fill gaps of missing data. For example, to ensure that there’s proper representation across all groups.
Q: What is driving the market need for synthetic data?
Wujek: Analysts predict that by 2026, 75 percent of businesses will use generative AI to create synthetic customer data. We certainly see synthetic data being used, or planning to be used, in several different industries. You can think about health care where patient confidentiality is of utmost concern. Health care organizations and research organizations within the health care and life sciences industries can really take advantage of data to find new discoveries and treatments for conditions that they perhaps haven’t had an opportunity to in the past simply because they didn’t have access to the data due to privacy concerns. Synthetic data can fill that role.
Related: SAS acquires Hazy synthetic data software to boost generative AI portfolio.
Within the government sector it is all about people and processes and defining policies to provide services to the right people at the right time. And a big issue there, of course, is ensuring that we have proper representation that those services, and the decisions being made to apply those services, are fair across the entire population. Ensuring that the data being used and fed into the models in those policies is very representative of the full population. So synthetic data can help balance the data that’s available to feed the AI that’s driving those decisions.
Q: Does synthetic data solve data management issues?
Wujek: Synthetic data can solve data management issues that have challenged organizations for years. Organizations spend a lot of time acquiring data, preparing data and cleaning data for their AI development efforts. It’s not a one-time process. It happens repeatedly. With a reliable synthetic data generation process, organizations can avoid costs associated with data acquisition and preparation and essentially “turn the crank” on the data they need at any given time.
Q: How is synthetic data currently being regulated?
Wujek: Regulatory bodies and policymakers are taking note of synthetic data and the role it’s playing in AI in helping drive innovation. Synthetic data is not getting a free pass. There are standards being put in place, whether it’s ISO, GDPR or the EU AI Act. There is some level of control over the use of synthetic data as part of this process to ensure that it is being used in a proper manner and following essentially the same sort of standards and guidelines that regulatory bodies impose on “real data”.
Q: What about synthetic data and trustworthy AI?
Wujek: Throughout the data and AI life cycle, organizations need to be mindful that there isn't any sort of leakage of private or sensitive data and fair representation across all groups. This is exactly what synthetic data is trying to achieve. Once you get AI applications in place, the next step is validating decisions and making sure decisions don’t start to deviate from what is expected. At that point, you need to revisit the data and AI life cycle to regenerate synthetic data that is more representative of the current conditions. Synthetic data should be used in conjunction with the full data and AI life cycle to ensure trustworthy usage and application within the decision-making process.
Q: What are real-world use cases?
Wujek: In the banking industry, fraud is a huge concern. Fraudulent transactions cost organizations a lot of money. Fraud, by nature, is considered a rare event. Therefore, we don’t have a lot of data on fraudulent transactions. Synthetic data can help provide information about those cases that we don’t have a lot of data on and that we want to be able to model to capture and identify when fraudulent transactions are occurring.
In marketing and retail, it’s all about matching up people and products. To do that typically, we are relying on historical transactional data and the behavior of customers, perhaps website traffic information. And all this, again, is very personal information. Where we are relying on access to that data and the availability of that data to be able to develop models that can help drive decisions about how to best serve customers. And so, this is where synthetic data can really come into play and provide some of that data that maybe customers don’t want to be shared. And that can help create models and drive decisions to help position products better.
In government, it’s largely about people and processes and defining policies to ensure that we’re delivering services to the right people at the right time in the right manner. This allows us to validate those decisions upfront before we implement them, potentially having an adverse impact on real people.
Q: Do you predict a faster data and AI life cycle because of synthetic data?
Wujek: Yes, synthetic data will drive significant advancements and innovation. It solves many data acquisition and management problems so data scientists can train models faster with complete, representative and trusted data. Synthetic data will fill holes where real data is hard to come by or when rare-case scenarios need to be detected. We will see remarkable innovation, from finding therapies for rare diseases to preventing fraud in financial services.