AI tools should, ideally, prioritize human well-being, agency and equity, steering clear of harmful consequences.
Across various industries, AI is instrumental in solving many challenging problems, such as enhancing tumor assessments in cancer treatment or utilizing natural language processing in banking for customer-centric transformation. The application of AI is also gaining traction in oncologic care by using computer vision and predictive analytics to better identify cancer patients who are candidates for lifesaving surgery.
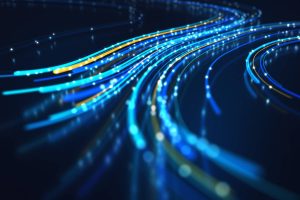
Despite good intentions, the lack of internality during AI development and use can lead to outcomes that don’t prioritize people.
To address this, let’s discuss three approaches to AI systems that can help prioritize the people: human agency as a priority, being proactive in finding potential for inequities, and promoting data literacy for all.
1. Human agency as a priority
As the use of AI systems becomes ubiquitous and starts to impact our lives significantly, it is important that we keep humans at the center. At SAS, we define human agency in AI as the crucial ability of individuals to maintain control over the design, development, deployment, and use of AI systems that have an impact on their lives.
Ensuring human agency in AI involves adopting various strategies. One notable approach is to require human participation and approval of certain tasks within the AI and analytics workflow. This is commonly referred to as the human-in-the-loop approach. This method promotes human oversight and agency in the analysis workflow, thus creating collaboration between humans and AI.
-
Fig. 1: Fostering human agency through SAS Workflow Manager’s capabilities of creating the AI & analytics workflow.
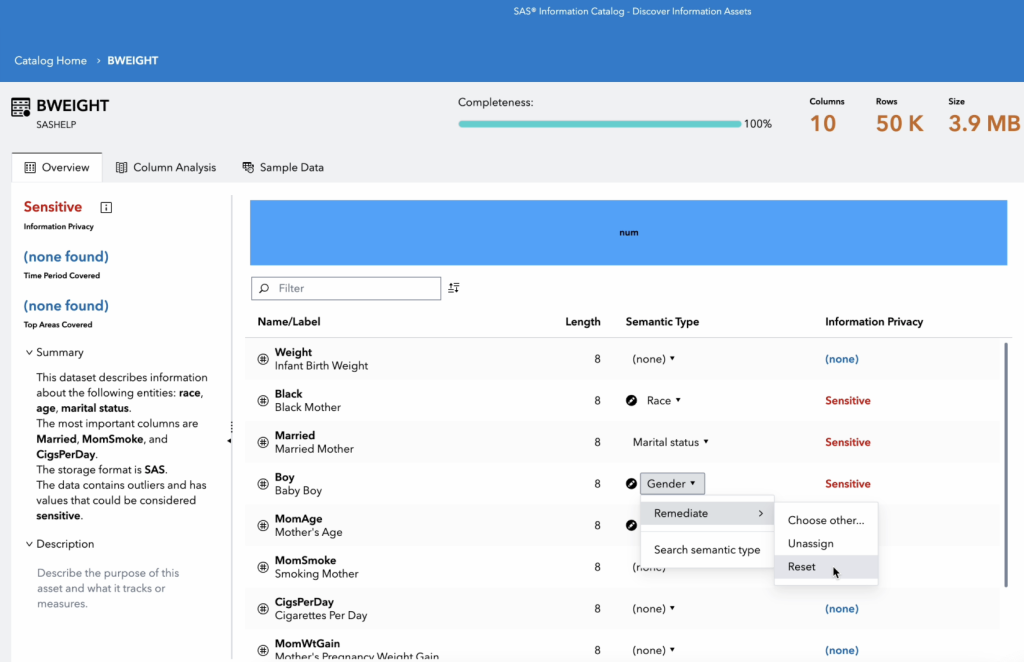
2. Proactiveness in finding potential for inequities
When striving for trustworthy AI, a human-centric approach should extend its focus to encompass a diverse and inclusive population. At SAS we advocate for a concentration on vulnerable populations, recognizing that safeguarding the most vulnerable benefits society as a whole. This human-centric philosophy prompts a crucial set of questions: “For what purpose?” and “For whom might this fail?” These questions help developers, deployers and users of AI systems evaluate who may be harmed using AI solutions.
Understanding the AI system's purpose can also clarify the target populations. This clarity can help decrease some of the bias by making sure that the AI systems are built on data representative of the population. Automated data exploration becomes a critical tool in this context, as it assesses the distributions of variables in training data and analyzes the relationships between input and target variables before training models. This proactive data exploration guarantees that insights derived from the data reflect diverse populations and address the specific needs of vulnerable groups. Once the model is developed, it should be reviewed for potential differences in model performance for different groups, particularly within specified sensitive variables. Bias must be assessed and reported regarding model performance, accuracy and predictions. Once groups at risk are identified, AI developers can take targeted steps to foster fairness.
Fig 3: Proactively understand potential differences in model performance across different populations using SAS Model Studio’s Fairness and Bias assessment tab.
Fig 4: SAS® Model Studio’s automated data exploration gives a representative snapshot of your data.
3. Data literacy for all
Finally, human centricity in AI also underscores individuals' agency in understanding, influencing or even controlling how AI systems impact their lives. This level of agency relies heavily on the concept of data literacy, as defined by Gartner. Data literacy is the ability to read, write and communicate data in context, including understanding data sources and constructs, analytical methods and techniques applied, and the ability to describe the use case, application and resulting value. As AI is being increasingly used to make decisions that affect our lives, it becomes essential that individuals develop data literacy. Being data literate not only at an organizational level but an individual level helps us understand how data is used to make decisions for us, and how can one protect themselves from data privacy risks and hold AI systems accountable,
Learn more about SAS' free data literacy course
In prioritizing human agency, proactive equity measures and data literacy, a human-centric approach ensures responsible AI integration. This alignment with human values enhances lives, minimizing risks and biases in AI implementation. This will ultimately create a future where technology serves humanity.
Read more stories about data ethics and trustworthy AI in this series
Vrushali Sawant and Kristi Boyd contributed to this article.