AI – just like humans – can carry biases. Unchecked bias can perpetuate power imbalances and marginalize vulnerable communities. Recognizing the potential for bias is one of the first steps toward responsible innovation. Doing so allows users to include diverse needs and perspectives in building inclusive and robust products. Through the lens of inclusivity, we can shape AI's future.
A lack of inclusivity in a solution can cause unintended consequences. Facial recognition algorithms, for instance, sometimes misidentify people of color, placing them at a disadvantage. Similarly, language models tend to associate negative words with women more frequently than with men. These embedded and unmitigated biases reinforce harmful stereotypes and deepen disparities.
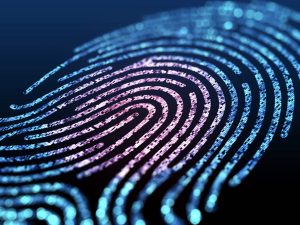
AI algorithms designed to detect cancer often fall short in underserved populations, and models predicting job performance are more likely to assume that women and people belonging to minority groups are less qualified, exacerbating discriminatory practices and further hindering equal opportunities.
Inclusivity also extends to users of a solution or platform. The importance of inclusivity in the design, development and deployment of data-driven systems can’t be overstated. Inclusivity entails ensuring the accessibility and integration of diverse perspectives throughout the AI and analytics life cycle. Organizations must assemble diverse, multidisciplinary teams to participate in problem definition and solution design to achieve this outcome. By actively involving diverse perspectives and experiences, we can address the needs and concerns of all community members.
Here are three ways we can effectively put inclusivity into practice:
1. Ensure comprehensive data representation
At the heart of responsible innovation lies comprehensive data representation. Data exploration is crucial to understanding the representation of diverse populations. It’s important to explore the distributions of variables in training data and analyze the relationships between input and target variables before training models. This approach offers valuable insights into whether people with similar characteristics experience similar outcomes. For example, when training an AI model to predict whether someone will be approved for a loan, you would want to make sure your data set includes people from all diverse backgrounds and income levels.
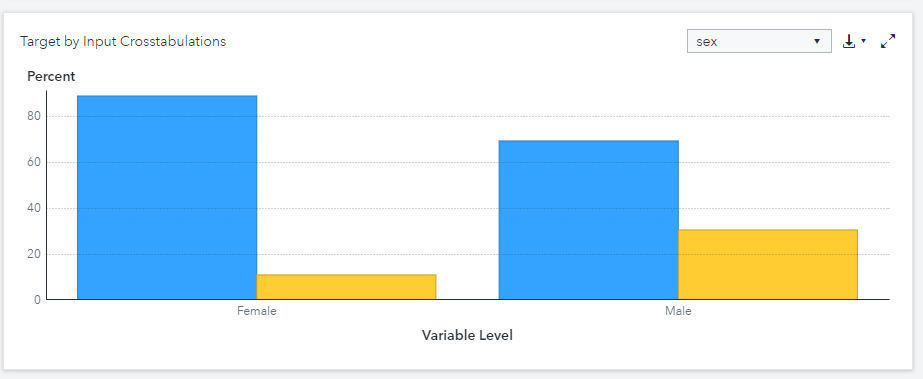
2. Prioritize all communities equally
Once the model is developed, it should be reviewed for potential differences in model performance for different groups, particularly within specified sensitive variables. Bias must be assessed and reported regarding model performance, accuracy and predictions. For instance, if an AI model predicts whether someone will default on a loan, it should maintain the same probability of predicting a default regardless of race, gender or ethnicity.
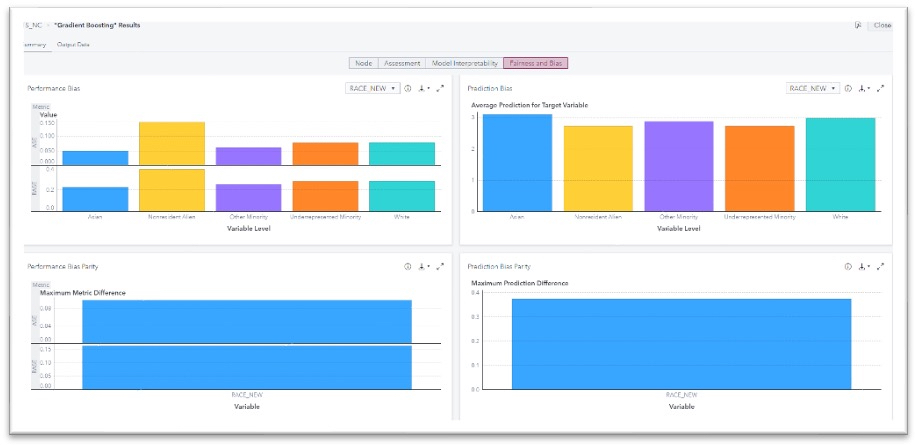
However, detecting biases is just the beginning. We must mitigate them as well. Bias mitigation techniques can be classified into three main categories: pre-process methods, which transform the data before model training; in-process methods, which consider fairness constraints during the model training process; and post-process methods, which adjust predictive outputs to compensate for bias without altering the model or data input. Data scientists must pick the appropriate bias mitigation methods that suit their project needs.
3. Design products with everyone in mind
Inclusive innovation extends beyond data representation, bias mitigation and equal prioritization. Inclusive innovation necessitates participatory and accessible solution design. By involving end users from diverse backgrounds throughout the development process, we can create AI systems that empower and serve the needs of all community members.
This includes considerations of intuitive user interfaces and compatibility support for multiple languages and technologies. For SAS, a dedicated accessibility team reviews and tests our software during design to ensure that products are not only compliant with accessibility requirements but also provide good experiences for users of assistive technology. Notably, several team members use screen readers in their daily lives. “I’ve worked closely with folks on the accessibility team and attended a few seminars they’ve hosted,” says Sierra Shell, a UX Designer. “I’ve seen how effective the team is and how much ground they can cover, given the expertise of contributors who intimately understand assistive tech usage.”
Creating an inclusive future for technology
Inclusivity is not just a buzzword; it is a guiding principle for the future of responsible innovation. Recognizing and mitigating bias are important steps toward shaping a future where technology will be inclusive and fair. We have seen how unchecked biases in AI algorithms can perpetuate power imbalances and deepen disparities, hindering equal opportunities for marginalized communities. To ensure inclusivity, we must consider comprehensive data representation, take into account all communities equally and design products with everyone in mind. This requires diverse, multidisciplinary teams working together to address those needs.
But inclusivity is not limited to the development process. It also extends to the users by focusing on intuitive interfaces and accessibility for diverse populations. By actively embracing inclusivity in responsible innovation, we can create a future where technology serves and empowers all, regardless of backgrounds and circumstances. Through this collective commitment, we can unleash the true potential of AI and help create a more equitable and just society.
1 Comment
With EU legislation maturing, love we have a DEP here to ground our work and influence the global AI conversation! https://www.cnbc.com/2023/06/14/eu-lawmakers-pass-landmark-artificial-intelligence-regulation.html