As organizations embrace AI, they often handle large volumes of data that power AI systems. Handling data appropriately includes implementing adequate privacy policies and security measures to protect it. Doing so prevents accidental exposure and ensures ethical data use.
AI technology often uses sensitive data for creating, training and utilizing models. This data may contain private information about people, sensitive business use cases, or health care data. Unauthorized access to or inappropriate disclosure of these types of data can cause harm to individuals or organizations. In response, governments have expanded privacy and security laws. These laws protect individual data subjects' privacy and set up guardrails to minimize sensitive data exposures and data breaches.
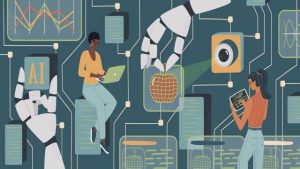
While privacy and security were previously associated with intellectual property (IP) and cybersecurity, the definition and scope have expanded in recent years.to encompass data access management, data localization and the rights of data subjects. It’s important that organizations, especially those using or building AI solutions, be aware of privacy and security best practices and regulations.
Interestingly, existing privacy regulations overlap with upcoming AI regulations, as both emphasize principles such as explainability, fairness and security. In fact, a recent study found that more than 50% of organizations develop their AI governance on top of existing privacy frameworks. These organizations see parallels between keeping data secure and keeping AI models well-governed. Responsible innovators understand the need to meet regulatory requirements and respect the privacy and security of training data subjects.
Let‘s discuss three things organizations can do to make sure their data is more private and secure.
1. Data masking: Respecting the privacy of subjects
A global study recently found that 68% of consumers are concerned with their online privacy. While the study focused on data collection while browsing online, the message is clear – consumers care about their data and how it is collected, processed and used. For organizations processing data containing personal or sensitive information, it is imperative to effectively protect data subjects’ privacy and data masking can help.
Data masking is a technique used to protect sensitive information by randomizing, substituting or reshuffling original data with similar values. In other words, you’re “hiding” the data's values. This method ensures that the data remains usable for testing, development or training purposes while minimizing the risk of exposing sensitive information. Data masking can protect addresses, names, social security numbers, intellectual property and financial information, among other sensitive information.
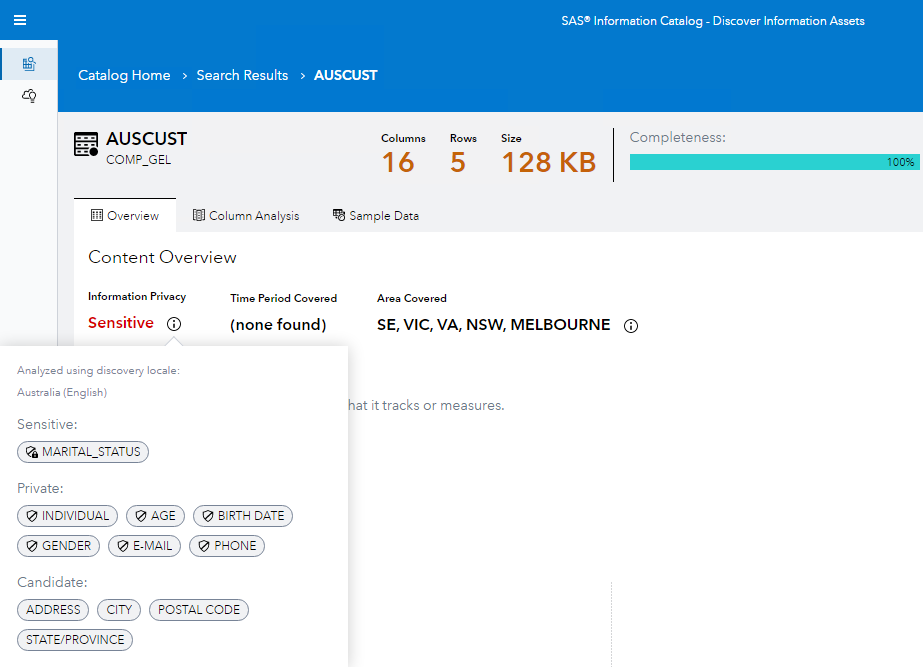
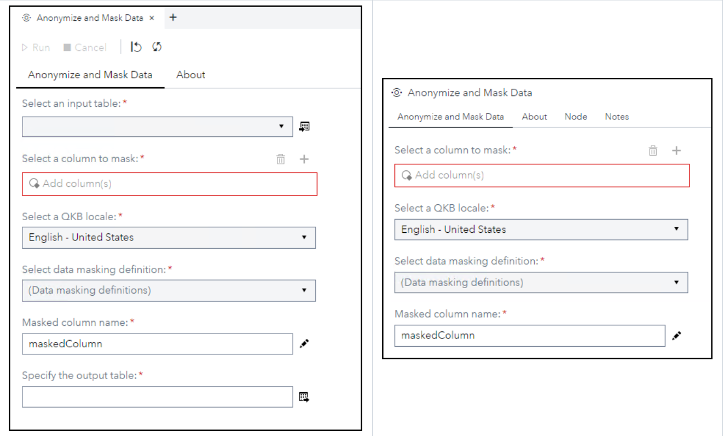
2. Authorization management: Who needs to know?
Authorization management is integral to data hygiene and data management. In a physical office, an organization may have spaces open to guests and visitors; employees only; or special access required. If you have a business visitor, you will likely hand out day visitor passes and invite them to the conference rooms but not give them the building security codes. Authorization management is a similar concept but in the digital domain.
Not everyone needs the same level of access when engaging with data and seeking data-driven insights. In many cases, only select data scientists in your organization need access to the full data set to find the most relevant and enlightening insights. These insights can then be shared with the appropriate employees without disclosing the underlying sensitive data. Authorization management asks, “Does this person need to see the data, or do they just need the insights?”
A good authorization management practice is to apply a least-privileges model that grants each role the minimum access required to complete the tasks they have on hand successfully. Similarly, setting up user groups to manage appropriate rights can help restrict data usage on a need-to-know basis. This approach ensures that those with a legitimate need only access sensitive information. By limiting access in this way, organizations can improve their data hygiene and protect sensitive information from those with unauthorized access or disclosure.
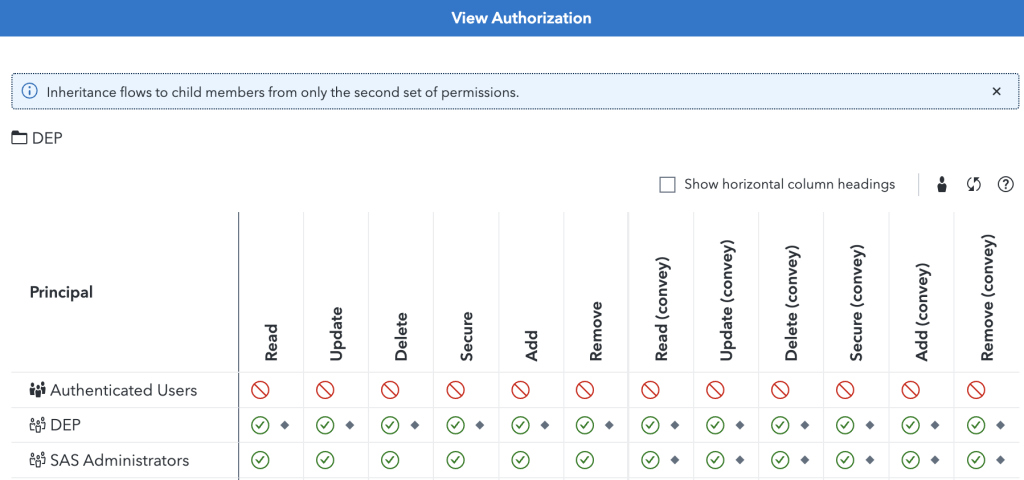
3. Safeguarding against external attacks
Data masking and authorization management provide privacy to the data subjects when their data is used within the organization. However, it is important not to overlook the possibility of adverse external influences. Organizations are exposed to increasing malicious attacks, which increases the importance of having a private and secure environment.
One type of attack that organizations should be particularly wary of is adversarial attacks. These attacks can muddy the data and introduce incorrect variables in the machine learning models by fooling the models with deceptive data and labeling. This can lead to incomplete or inaccurate data points, which may harm not only the business but also the individual employees and users of the system.
For these reasons and more, organizations should take active steps to mitigate these external attacks by using adversarial training, detecting and cleaning adversarial inputs, and implementing differential privacy in training data. Additionally, monitoring input variables that exhibit substantial distribution shifts could help defend against data poisoning attacks.
Upholding ethical standards
At SAS, we consider privacy and security principles essential to responsible innovation. As a global company with operations in over 50 countries, we collect personal data from our customers, prospects, partners, suppliers, applicants and employees. To protect the data as a data processor and controller, we work with multiple third-party and regulatory frameworks to stay on top of industry standards and regulations.
With data masking, authorization management and proactive defense against external attacks, organizations can create a private and secure environment to protect data. These measures protect individuals and organizations and uphold ethical standards and regulatory compliance in the AI landscape.
Stay informed about where your data is located, how it is used and how we protect and defend your data.
Kristi Boyd and Vrushali Sawant made contributions to this article
2 Comments
Thanks for the article Kristi! Great info
It's such a simple question to ask, "where's your data?" I wonder how many organizations cannot, fully answer that question.