If you think machine learning will replace demand planners, then don’t read this post. If you think machine learning will automate and unleash the power of insights allowing demand planners to drive more value and growth, then this article is a must read.
The impressive advances in artificial intelligence (AI) and machine learning (ML) over the past decade are supported by supervised deep learning: training ML algorithms to perform narrow, single-domain tasks. The learning is supervised because you're telling the algorithm the correct answer (the label) as it is exposed to many examples using big data. We're now seeing unsupervised learning systems that learn faster, require less data, and achieve impressive performance. These supervised and unsupervised “intelligent automation” techniques can help experts achieve automation while enriching their domain expertise to do their work more effectively, not eliminate them.
Intelligent automation techniques can be applied to all kinds of activities across your organization to reduce the everyday repetitive work while uncovering key insights to improve the effectiveness of your processes, as well as your workforce.
How can “Intelligent Automation” enhance existing processes?
Forecast value added (FVA) has become a standard metric for most companies to improve not only forecast accuracy, but reduce non-value add touch points in the demand planning process, thus improving process efficiency. Consider the size of managing all those touch points using Excel. So, what if we boost FVA with machine learning? With the goal of reducing the complexity of managing all the FVA information through automation, while providing demand planners with targeted intelligence to pinpoint where, when and by how much to make manual overrides to the statistical forecast.
Why is this important?
We've learned through experience that not all manual overrides, whether fact-based or experience-based, add value. In fact, many add very little value (Fildes, Goodwin 2007). The challenge is not only to identify overrides that are non-value add, but also those that are value add. FVA introduced by Mike Gililand (John Wiley & Son, 2010) is a way to identify non-value add overrides, and value add overrides.
This situation is made more difficult when you have thousands of SKU’s (stock keeping units) across multiple channels including brick-and-mortar, mobile, online, Amazon.com, and other related ecommerce channels in multiple countries requiring millions of forecasts. Using Excel makes it impossible for demand planners to review and manually adjust all those forecasts. So, they rely on aggregate level adjustments that are disaggregated down their business hierarchies. In many cases, mass aggregation of overrides is not an accurate way of manually adjusting statistical forecasts even when based on additional sales and marketing activities not considered, or available during the origination of the statistical forecast.
A practical application of “intelligent automation” techniques
Recently, SAS tested a new patent pending intelligent automation technique that uses machine learning to boost FVA with a large global consumer packaged goods (CPG) company using ML to learn from past demand planners’ manual overrides with a focus on two main objectives:
1) Identify forecast entities that need overrides.
2) Provide demand planners with the direction and range of overrides (as to the need to raise or lower statistical forecasts) at various levels of the business hierarchy.
Machine learning analyzes the past statistical forecast and consensus forecast overrides learning from successful and unsuccessful forecast adjustments to detect the best periods to review candidates for overrides. Then, providing guidance to demand planners where and by how much -- suggested upper/lower volume range -- to adjust the business hierarchy forecasts by either raising or lowering the statistical forecast.
Process approach
A minimum of two and half years of historical overrides based on an 18-month rolling forecast were collected for five product categories in two geographic areas for over 700 items. A 60-day future forecast was used for FVA purposes. In-sample/out-of-sample training and validation periods were used in comparison to the FVA analysis to choose the appropriate ML model. A three-step approach was implemented: 1) Enrich 2) Model, and 3) Assess (see Figure 1).
Step 1: Enrich the process by identifying value add and non-value add overrides made by several demand planners, and add any available attributes that were available.
Step 2: Build machine learning models using neural networks, gradient boosting, and ensemble random forest training models in a competition to determine the champion model.
Step 3: Assess models using the out-of-sample validation data and report the accuracies.
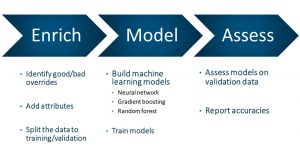
These steps can be enhanced by adding additional causal factors like sales promotions, pricing strategies, and others. Unfortunately, for this case there were no causal factors to enhance the ML models for this POV (proof-of-value). Also, smart rules can be added. For example, don’t make overrides if the MAPE (mean absolute percentage error) is less than 10 percent, and/or only consider a period for FVA analysis, if the three-month historical FVA average is greater than 30 percent.
Results
The machine learning boosted forecast value added process reduced the number of manual overrides by 47 percent, allowing the demand planners to focus only on those products in the business hierarchy and periods that would add the most value from their overrides. As a result, it improved the value added to the forecast by 6.3 percent.
Overall, machine learning helped to improve the statistical model accuracy on average across all the product groups, items, and geographies with the best champion ML model (neural networks) as follows:
- Best ML model average accuracy across all product groups, items, and geographies was 75 percent.
- Accuracy if ML recommends no overrides: 86 percent.
- Accuracy if ML recommends overrides: 65 percent
Given these results, using ML to boost the FVA process has demonstrated that it can automate demand planners mundane work of managing the enormous amounts of data required to support the FVA process while providing targeted intelligence to pinpoint where, when and by how much to manually adjust the statistical forecast. The real benefits are the ML recommendations where not to make manual adjustments to the statistical forecast, as it is much more accurate than their prior overrides.
Conclusions
Intelligent automation will help demand planners to sense and synthesize vast amounts of information boosting the FVA process guiding demand planners with surgical precision to work smarter. Demand planners will be able to ingest and analyze massive amounts of forecast information, respond quickly to complex inquiries, and make overrides with laser precision across the entire business hierarchy.
Similarly, as automobile’s onboard computers can now be connected to diagnostic machines allowing an auto mechanic to assess the problem within minutes (versus hours manually) pinpointing the exact failed part helps facilitate his/her ability to work smarter, not be replaced by the machine.
The rapid deployment of intelligent automation is ushering in the second machine age where computers and other digital advances are boosting our mental power—the ability to use our brains to understand and shape our environments more effectively, and set new standards of quality, efficiency, speed, and functionality. Instead of being replaced, demand planners will be elevated to a strategic role.
“The conversation we should have is how machines and algorithms can make us smarter, not how smart we can make the machines…” Tom Gruber, Co-creator of Siri (TED Talk, 2017)
Learn more about how AI/ML can deliver "intelligent automation"