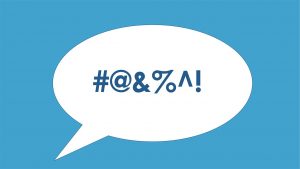
When a person feels sufficiently wronged to lodge a complaint with the Consumer Financial Protection Bureau (CFPB), there’s likely to be some negative sentiment involved. But is there a connection between the language they use and the likelihood they will be compensated by the offending company?
At the upcoming Sentiment Analysis Symposium, I will discuss how machine learning and rule-based sentiment analysis can support each other in a complementary analysis, and produce actionable information from large amounts of free form text. In this case, machine learning and sentiment analysis could improve and evolve the CFPB’s ability to assess consumer complaints.
This is accomplished by identifying patterns between degrees of negative sentiment expressed in free-form consumer complaints. A model which generates rules based on this free-form text, where the related companies ended up paying out compensation as a result of the complaint. These machine-generated rules indicate patterns in the free-form text which tend to only be present in the cases of monetary compensation.
Examples include types of lending and retail companies associated with the lending but not present in the structured data. For example, if someone lodges a complaint about bank fees, and uses a derivative of the term “steal”, it is more likely to be associated with some kind of financial recompense. This goes beyond traditional sentiment analysis, identifying key negative terms, in a particular context, to highlight patterns associated with a result.
Visual analytics provides these newfound insights with illustrative structure – a previously hidden, yet incredibly valuable, map of areas of concern, including predatory lenders or credit card companies with substandard customer service.
Being able to rapidly identify and visualize key information – to anticipate something like consumer sentiment – has huge implications for the entire global economy. But the speed at which the analysis can be set up and operationalized against the data also makes it a game changer for predicting, preparing for and responding to population and infrastructure threats, such as natural disasters and public health crises.
I asked Sentiment Analysis Symposium organizer Seth Grimes, a thought leader in the text analytics sphere, for comment. Reflecting a perspective that aligns with my own, Seth says, "It's cool that SAS is able to show that detecting signals in consumer, health and behavioral and correlative big data can help agencies (and corporations) meet mission and public needs while saving enormously on information processing costs. I've worked with SAS for decades and recognize SAS as a leader in text analytics and sentiment analysis, so it's great to see it applied, per Tom's talk, for public benefit."
.@sethgrimes: I've worked w/ SAS for decades & recognize SAS as a leader in text analytics & sentiment analysis. Click To TweetWith a natural disaster, officials could use machine learning and sentiment analysis to visualize patterns between mood-state indicators (social media posts geo-tagged near the affected area) and existing field data (why and when certain people visited a particular clinic on a given day) to better understand how to allocate resources and sharpen future preparedness efforts. For example, the analysis may indicate that there were a number of cases where individuals required oxygen, or access to certain prescription drugs such as warfarin. Knowing this, and providing access to these resources during a crisis will help to preserve lives.
Similar analysis could similarly improve the ability of epidemiologists to catch and fight infectious disease outbreaks early on, and of public health researchers to identify prescription drug users at-risk of overdose.
Interested in learning more? Check out the SAS White Paper Combining Knowledge and Data Mining to Understand Sentiment – A Practical Assessment of Approaches and attend the 2016 Sentiment Analysis Symposium, being held July 12 in New York City.
4 Comments
We found that when trying to identify issues or areas of concerns, we wrote queries to identify the Top 25 Negative Noun Tokens in Sentences and include the related sentences after Natural Language Processing. We then grouped those sentences for tagging in an interactive tree (tree of sentences). We were able to identify the top issues affecting consumers, very quickly; because of the refined sample size (Top 25 Tokens). We would repeat this effort with each week of new data...slowly becoming the knowledge experts in the source domain. As the unique issues started to dry up we instituted a dynamic filtering system where every keyword in a sentence became a filter. We could shuffle the results with each click, spinning the results. We also implemented the ability to combine those keywords and flip them for even more complex dynamic filters. And then we also started an automatic favourite keyword identification system so that on subsequent weeks of results, I knew which keywords/favs were able to pull back the targeted results we were after. So for those looking to find the top negative issues, this may be a plan of attack in the identification of issues, something you could include in your own system or check out my site to see this in action. Hope this helps someone when the uniqueness in the results seems to dry up.
A video of the presentation this past July is now available - go see at https://vimeo.com/179984693. It'll be worth your time.
I will be presenting an internationalized version of this at LT-Accelerate (http://www.lt-accelerate.com/) in Brussels, Belgium. LT-Accelerate is Europe’s premier event for social, text & speech analytics technologies and their business applications.The conference runs November 21-22. I believe all presentations will be available after the conference and will post a link.
You can find all presentations from lt-accelerate here, enjoy! http://www.lt-accelerate.com/programme