To some people, electricity is like air: There for the taking. For others, circumventing paying a utility bill is a just cause, sticking it to “Big Energy” for their perceived transgressions against customers.
In either case, not paying for energy is considered fraud and a crime. In some states, energy fraud is a felony worthy of hard time and steep penalties.
So why the big deal over a few customers not paying their “fair share?” The numbers tell the story. A recent study by Deloitte pegged the utility fraud problem at $6 billion in North America. One large investor-owned utility estimates their lost annual revenue due to fraud at $100 million, conservatively. Those few customer sticking it to "The Man" add up.Utilities leaving millions, tens of millions, or even hundreds of dollars off their income statement due to energy theft and fraud creates a difficult business environment. That’s money that can’t be invested in the utility’s core business of keeping the lights on, maintaining customer satisfaction, and doing so in a safe, sustainable, affordable way.
The good news is that there is a light at the end of the tunnel (and it's not the flashlight of someone rigging their meter to reduce or eliminate their bill). Low-tech solutions include consumer education, tamper-proof meters and a legal environment that supports stiff penalties for energy theft and fraud. High-tech solutions involve the use of smart meters that generate data that tells a more complete story about energy consumption at a specific customer site.
The availability of rich data sets for customer premises creates opportunities to learn more about customer patterns overall. In working with customer data, systems used to analyze the data must be able to distinguish between normal and abnormal usage patterns, and distinguish between different types of abnormal. For instance, is the abnormal energy usage pattern due to theft or is the meter faulty?
To help utility companies fight this battle, different technologies and methodologies have emerged in recent years: Energy balancing, rule-based, signal processing and machine learning. Table 1 below summarizes the pros and cons of these four methodologies.
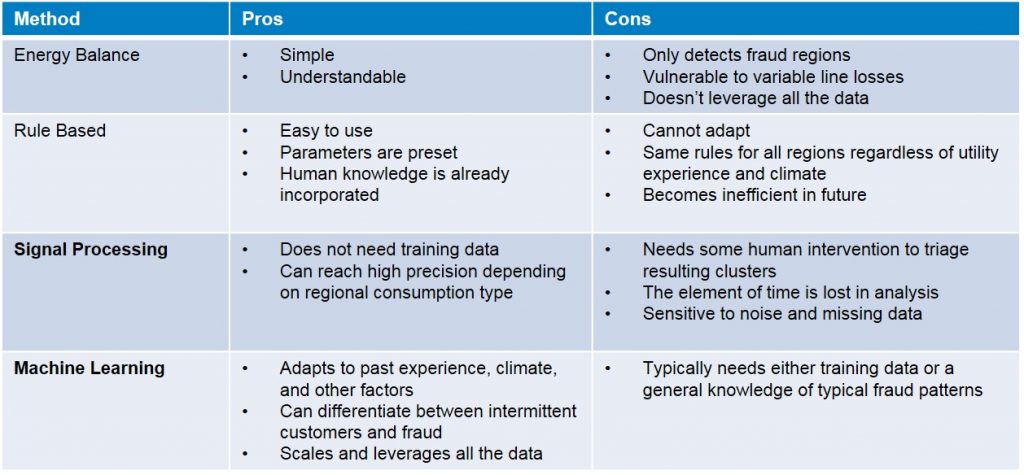
Of note, the first two methodologies, energy balance and rule-based, are easier to implement but are limited in their effectiveness. Conversely, the second two methodologies, signal processing and machine learning, are more complicated in terms of staff training requirements and implementation, but yield a better ROI in the long term. Essentially, the energy balance and rule-based methodologies will perform to a certain level from the start and stay that way. But the signal processing and machine learning methodologies continue to improve in their ability to identify fraud as more data is analyzed over time.
Figure 1 below is a screen shot of the presentment from a signal processing and machine learning based fraud detection solution. It includes location information, a graphic presentation of the anomaly in the daily load at a customer site, and red flags for customer accounts that are suspected of fraud, making this a powerful tool for identifying fraud and taking action on fraudulent accounts.
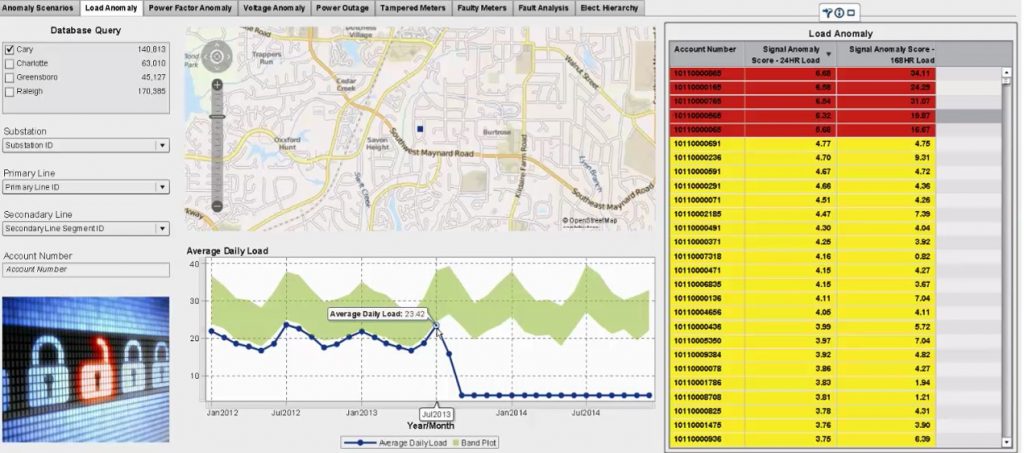
Fraud is a multi-million dollar problem for nearly every utility in North America, and a $6 billion problem for the entire industry. Fortunately, analytics solutions exist today to mitigate this issue and bring some of those missing dollars back onto the utility’s income statement.
The SAS Fraud Framework leverages signal processing and machine learning to provide a powerful long-term fraud solution. It includes configurable scoring, decisioning and a routing engine which provides a framework for operationalizing the scoring models; a prebuilt investigative user interface to surface and disposition alerts; and advanced link analysis, allowing analytics to be used to determine hidden links and associations that could be indicative of suspect activities, including possible internal collusion by employees or contractors.
Download this solution brief to learn how utilities can detect and prevent fraud with greater speed and accuracy.