Machine learning is not new. SAS has been doing it for over 20 years and some early machine learning papers date back to the 50’s. So why is it one of the hottest topics at the Strata Hadoop World conference later this week? Clearly, Hadoop is playing a major role in the increased focus on machine learning.
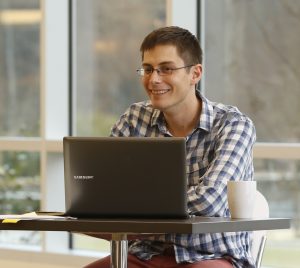
owerful, low-cost distributive computing environments coupled with Hadoop give data scientists the ability to run iterative models (like neural networks) they may not have been able to in the past. In addition, organizations are collecting more data than ever before. The Internet of Things is just the latest source of data now being collected to analyze. The addition of all this data allows models to learn more and make better predictions.
Over the past two weeks, we’ve shared a few of the key technologies SAS will be demonstrating and talking about at Strata Hadoop World in San Jose this week. We first discussed the hot topic of streaming analytics, moved to support for Spark, visited visualization on Hadoop and even had a special guest blog from Jill Dyché sharing her thoughts on Big Data how it can be used for good. Now it’s time to wrap up our pre-conference coverage by diving deeper into machine learning and with Patrick Hall.
Patrick is a Senior Machine Learning Scientist at SAS and adjunct professor at George Washington University. If you're attending Strata this week, stop by the SAS booth (#1022) to ask Patrick anything about machine learning. I had a chance to meet with him recently, and asked him a few of my own questions:
What are some emerging business opportunities in using machine learning?
Patrick Hall: I see emerging opportunities in three areas:
- Replacing traditional approaches. Machine learning approaches can potentially be more accurate than traditional BI or statistical approaches for solving the same problems.
- Augmenting traditional processes. In some regulated industries, machine learning techniques can be a hard sell to leadership or regulators. Also, many organizations have been getting great results from their traditional models for years and don't want to reinvent the wheel. If that's the case, organizations can consider augmenting the processes around traditional models with machine learning techniques. For example, they could use a machine learning model to predict when a traditional deployed model will go stale, and replace or retrain that deployed model before its performance starts to degrade.
- New types of data and analyses. Machine learning techniques excel at analyzing the kind of data used to represent text and images. Information extracted from unstructured data can be used to augment existing models -- or lead to totally new insights.
Can traditional industries increase revenue with machine learning?
Hall: Yes. In certain types of portfolios, every one percent increase in model accuracy can lead to millions of dollars of increased revenue. Also, creativity isn't restricted to startups. I see highly skilled machine learning practitioners in traditional industries doing things I could never have anticipated. Of course, not every bright idea leads to increased revenue, but some certainly do.
What challenges do organizations face when beginning to use machine learning?
Hall: Challenges can be broken into two basic categories: Those that involve people and those that involve technology.
- People challenges. Successful incorporation of machine learning into an organization's analytics strategy can require new types of business processes, new types of practitioners and new types of management and leadership. Since machine learning is often used for automatic decision making, using it successfully also requires increased levels of trust between technical groups and leadership.
- Technology challenges. Successful use of machine learning can also require new technological infrastructure. Traditional RDBMS are often not the best way to store unstructured data. Training machine learning models can require GPUs or clusters of computers, and standing a model up for 24/7/365 automated decision making in an operational server is a lot more involved than calling 'predict()' on someone's personal laptop.
What does machine learning mean to you?
Hall: It's important to understand that real science almost never moves at the pace of Internet hype. Machine learning probably isn't going to change the world the way running water, telephones or electricity did (and still are in some places). It's really just another tool in the data science toolkit, well-suited for automated decision making, but with its own set of strengths and weaknesses. That said, I'm a big believer in the capacity of data science to make life a little easier and more efficient for everyone. So, the more tools we have in the data science toolkit, the better.
What excites you the most about your role as a data scientist?
Hall: I’ve always liked solving problems with and about data. For instance, when I was in chemistry graduate school, I found myself more interested in how to visualize the data from my results than in the actual scientific significance of the results. Now, as I've become more involved with data science, I'm learning how to apply the scientific method to experiments with data itself. Applications of data science have enjoyed great commercial success for about 50 years now, and at SAS it’s exciting and rewarding to play a central role in many commercial data science success stories. I'm also really hopeful about the societal advances that can be made by the academic study of data science and data from across scientific and humanistic disciplines.
If you’re looking to learn more about machine learning read the article, Introduction to machine learning: Five things the quants wish we knew or watch the on-demand webinar, Machine Learning: Principles and Practice.
Stop by the SAS booth (#1022) to chat with Patrick, pick up a “Data Dude” or “Data Diva” t-shirt and meet the rest of the SAS team. You won’t want to miss Patrick’s presentation with Paul Kent on March 30th at 4:20: A survival guide to machine learning: Top 10 tips from a battle-tested solution.
It’s not too late to register for Strata Hadoop World. Use the discount code SAS20 to receive 20% off of your registration.