It’s been very hot here in Northern Italy: electricity provision has struggled to keep up and we’ve had frequent power outages in the area, even within our apartment building. A bit inconvenient? Don’t get me started. I feel like my home appliances have turned against me, taking me back to the Stone Age.
This begs the question – why do we continue to have this problem? What’s the cause? Is it the grid? Is it excessive appliance usage? Could this have been prevented? From my perspective as a data analyst, I would wholeheartedly say – “absolutely, a resounding yes! This can be prevented.”
Let me explain.
First: based on similar failures in the past, create predictive models that assess the likelihood of appliance malfunction (… and I hope this doesn’t jinx things!), such as dishwashers, electric curtains, washing machines, refrigerator, dryers, and – of course, air conditioning. What would these models do? Well, they would consider operating characteristics of the different machines, isolate key critical factors indicative of past failures. Then these factors can be monitored to predict the likelihood of future failures - ahead of time - and generate alerts for repairs, usage adjustments, and the like. But that means a lot of individual model, so an automated method to create such predictive models, like SAS® Factory Miner would be the ideal solution, building a “factory of models”.
For each appliance, or even for each critical part of every appliance, an accurate analytical model could be quickly built using modeling templates. All that's needed is the data that contains the historic readings of the various operating characteristics of my appliances and the recorded event of a past failure. What next? Well, I do know that my appliances have different risks and impacts associated with failures at different times of the year or due to changing factors.
For example, my dishwasher and TV might be more likely to fail in summer, because of the frequent power outages from my overloaded air conditioner. My dishwasher, on the other hand, cannot, I repeat … cannot break on me at Christmas time, when we host about 20 relatives in a one-bedroom apartment and eat ridiculous 20-course meals (yes, a hazard of being Italian).
And because different types and causes of failure happen at different times of the year, and under different conditions which impact me in different ways, I also need to include business rules to control for the different scenarios that determine when a specific predictive model is applicable.
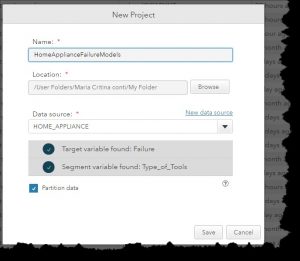
With these business rules defined, I’ve now gone from having a slew of predictive models to having a well-defined decision framework. I can now apply different models and different rules based on the modeling segments and the specific scenarios. For example, I know that the age of the appliance does influence the possibility of failure, so I’ll use more stringent rules when the appliance is older and have more flexible rules for the newer appliances I have. I will create this decisioning framework using a powerful tool such as SAS® Decision Manager.
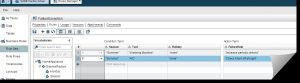
So now I have one system for real-time monitoring and the generation of early warning alerts. And I could even extend this to capture the sensor information generated from the appliance (with SAS® Event Stream Processing), applying the model and business rules in real-time continuously monitoring the health and well-being of my appliances.
At that point, I can finally rest easy and be assured that I’ll get a timely warning on my smartphone app, informing me about critical changes in the performance of one of my beloved home appliances, and even with guidance on steps needed to avoid pending malfunction. This means that the next time I go to work and schedule my washing machine, I will make sure I don't have to come home to unwashed, wet clothes that have been stuck in dirty water for the past five hours.
I wanted to share this application with you as a simple example that might help you envision an operational process within your organization where common, every-day decisions could benefit from automated intelligence. In the era of big data, analytics are the best and most effective way organizations can consistently drive repeated, operational decisions with accuracy and confidence.
A manufacturing company, for example, doesn’t have just one washing machine, and they most definitely don’t want to have to manually check their production line for every single piece of machinery they produce in every warehouse they have around the world. They want to make sure the likelihood of failure of a machine, or the probability of a malfunctioning component, are known at every point in time – prioritizing maintenance schedules and controlling processing so breakages and interruptions are minimized.
In my case the cost of the breakages was relatively small: I had to throw away one of my favorite t-shirts because it sat in the broken machine for too long, and I had to repair a few things around the house. But imagine what would happen to that manufacturing company if similar problems go undetected or are detected too late.
All organizations have operational considerations – whether it’s mechanical operations, decisions systems or front-line personnel, knowing in advance the likelihood of success or failure with the specific scenario rules provides the prescriptive instruction needed to act with confidence and consistency.
That's the power of analytics with decision management – scalable to even the broadest operations. The analytical knowledge it takes to build models and rules is applicable to any scale of operations when it’s supported by appropriate business knowledge.
If you’re curious to learn more about how you can use SAS® to take advantage of advanced analytics in your business to make repeatable and objective operational decisions for your enterprise, check out the new SAS® Factory Miner and the new release of SAS® Decision Manager.
And if in the meantime you’ve figured out how to teach my husband how to use the washing machine … please let me know.