The real reason companies cleanse the historical demand is that traditional forecasting solutions were unable to predict sales promotions or correct the data automatically for shortages, or outliers. To address the short comings of traditional technology, companies embedded a cleansing process of adjusting the demand history for shortages, outliers, and promoted (incremental) volumes by seperating them from the baseline volume. The theoretical rationale for manually adjusting (cleansing) demand history by removing promotional spikes and outliers supposedly improves the forecast results. Furthermore, it is believed that cleansing the actual history will produce a true historical baseline. In practice cleansing data does not improve forecast accuracy. On the contrary, it actually makes the forecast less accurate. This is primarily a result of the statistical methods being deployed in the technology--mainly exponential smoothing methods--which are not capable of measuring and predicting sales promotions or automatically correct for shortages and outliers.
In my experience any manual adjustments to the historical demand data or to the future demand forecast tends to make the forecast less accurate due to personal bias whether intended or not. Yet, close to 70% of companies continue to adjust historical sales data for trend and seasonality according to a 2014 Industry Week Research Report (see Figure 1).
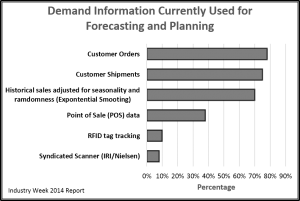
Exponential smoothing methods can only measure trend and seasonality. As a result, the related sales promotional data had to be eliminated from the history, as well as adjusting for shortages (outliers). After cleansing the demand history the baseline is modeled using a moving averaging or an exponential smoothing method — which was essentially forecasting smoothed trend and seasonality simulating a moving average — the sales promotion volumes are then layered back using judgment.
Exponential smoothing methods are traditionally deployed in over 90% of demand forecasting and planning solutions. So, a cleansing process needed to be adopted to seperate baseline demand (primarily trend and seasonality) from promotional spikes and outliers. Those anomilies were then either eliminated or adjusted accordingly using judgment, and then, layered back on top of the baseline volume.
Today, new demand forecasting and planning solutions can holistically model trend, seasonality, sales promotions, price, and other related factors that influence demand using predicative analytics (see Figure 2).
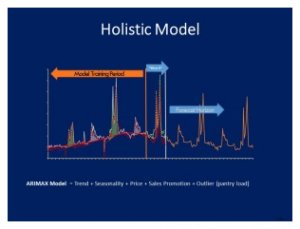
In addition, these same models can automatically correct for shortages and outliers without cleansing the actual demand history. Methods such as ARIMA, ARIMAX, dynamic regression, and Unobserved Component Models (UCM) can be deployed up and down the business hierarchy to holistically model trend, seasonality, sales promotion lifts, price, in-store merchandizing, economic factors and more. Intervention variables (dummy variables) can be used to automatically adjust the demand history for shortages and outliers. There is no longer a need to cleanse the demand history for shortages, outliers, and sales promotional spikes. In fact, these same predictive methods can measure the impact of sales promotions, calculate the lift volumes and predict the future lifts in different time intervals based on marketing event calendars. In addition, the commercial teams (sales and marketing) can spend more time running "What If" simulations with precision, rather than judgmentally layering back the promotion volumes to the baseline volume.
Manually cleansing historical demand? Let’s make that history.