By now, we have all heard about the Internet of Things (IoT), or the Industrial Internet. Across industries, organizations are attempting to instrument and measure all critical business systems and assets in an effort to drive improved production and service delivery. It is estimated that by 2020, companies will spend $500 billion on the Internet of Things. That growth in connected devices and increased data volumes will certainly create new value opportunities … won’t it?
The answer is yes, but the real value will only be realized when organizations make the connections within these new data streams to drive improved decision making and actions. It is surprising to me how many organizations are investing in improved sensing technology, without clear line of sight to how they plan to use it within their organization … or those that have visions of what this data could do for them, but struggle with how to get started.
During a recent customer engagement, we were discussing how to transform the customer's business model by optimizing their overall maintenance and reliability strategies, which would drive immediate impacts to bottom line profitability and give them a competitive advantage in the market. While the customer saw the value, they weren't sure how they could implement such a system in a greenfield facility. “We are just finishing the build out of the new facility, so we don’t have enough production machine data to get started," explained the customer. "In addition, since we haven’t been running in this location, we don’t have any failure events to leverage while building predictive models.”
In reality, I don’t think this concern is isolated, but it does represent a misconception about what is required to get started with sensor data. Why wait for equipment to fail before you start thinking about how to prevent it? As companies re-shore their operations or upgrade their existing operations, lack of historical machine data should not compromise their ability to develop a predictive maintenance strategy. For manufacturing and supply chain customers interested in protecting their critical business equipment, there are approaches and techniques available to harness value from sensor data starting with the first reading.
A common approach in many condition monitoring systems is threshold based analytics. Threshold rules can provide preliminary equipment coverage and serve as the foundation for an early warning identification system. In the past, I have seen this approach used in a variety of applications where extensive operating or failure histories were not available. This type of rule can be developed independent of operating data using first principles physics models, OEM specifications, industry standards, or be based on engineering knowledge/expectations of how the equipment should operate. Regardless of the approach utilized, threshold rules can be implemented to provide insight when a parameter falls outside of expected limits. Rule flows can be as simple as “If X > Y, raise an exception,” all the way up to advanced multi-variable ensembles.
Another approach that can be implemented for new equipment monitoring leverages pattern matching techniques. A classical approach from process manufacturing would be based on standard statistical process control rules (e.g. Western Electric). Equipment sensors can be continuously evaluated to flag conditions where the process or equipment is drifting out of control. For example, increasing trends, abnormal shift in the mean, oscillating values, etc.
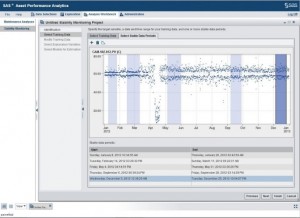
Generalized statistical models (e.g. Regression, ARIMA) can also be very powerful for providing initial equipment failure coverage. Although this approach does require data for model training, the amount can be extremely limited compared to traditional techniques. As new data is obtained, models can be retrained to continuously improve accuracy as new operating states, seasonal effects, etc. are observed. In some situations, pre-production data may be available before a facility transitions to commercial operations, which can also provide the baseline information required to leverage this approach.
Stability Monitoring, introduced in Asset Performance Analytics version 6.1, simplifies the model building process by providing an intuitive visual modeling environment, where “stable” periods of operation are defined for an asset.
Defined models compare the predicted behavior of a sensor to its actual behavior, with the goal of identifying equipment problems before they become a major issue. These deviations may relate back to mechanical failure, performance loss or any other process disturbance that isn't within expected operating envelopes.
As companies invest in new sensing technologies, there is value that can be extracted from day one. Waiting to act on that information is lost opportunity and negatively impacts business ROI. Obviously, more sophisticated models can be developed with richer input data, but a perceived gap in this area shouldn't dissuade organizations from starting the journey toward a functional predictive maintenance program. Start small … learn … and adapt as the data and system become more mature!