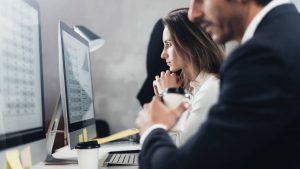
In my last post, I discussed the growing pool of regulations (both in the US and globally) that mandate protection of personal or private data. In the post, I raised a question about the degree to which we need to manage data asset metadata. Yes, I know I didn’t call it that last time, but if you're talking about characteristics associated with the data sensitivity attributes of a data asset – and the corresponding requirements for observing defined data policies (and consequently regulatory compliance) – you're referring to “data about the data.”
Note that this is different from the typical “structural” metadata that describes the names, lengths and types of structured data assets. In this case, I'm referring to qualitative information about the data asset, such as:
- The data owner and the data creator.
- The date of creation or acquisition.
- The size of the data asset.
- The number of records (if it's a structured object).
- And, importantly, characterization of the classification in terms of data sensitivity.
What is data sensitivity?
Since we're talking about regulatory compliance (and data privacy regulations like GDPR or CCPA), the obvious class of sensitivity is private individual or personal data. And as I pointed out last time, even though the pool of regulations is intended to prevent exposure of “personal information,” the definitions of personal information (and consequently, the policies for protection and management) may differ from one regulation to the next. That means you need to specify a classification scheme indicating the type of sensitive data according to the terms of each regulation.
For example, according to CCPA an individual’s social security number is considered personal data. But it's referred to as one of the 18 HIPAA-defined identifiers as “protected health information” (PHI). On the other hand, “education information” is listed as a type of personal information by CCPA – but it's not listed as one of the 18 HIPAA PHI identifiers. This means that a data asset containing individual social security numbers would be classified as CCPA-personal and HIPAA-PHI. But a data asset containing an individual’s college degree information would be CCPA-personal but not HIPAA-PHI. Compliance is then based on the defined policies associated with the different classifications.
Chief data officers: Consider all types of data that need protection
Once you decide to institute methods to protect against unauthorized access to personal data, why not consider other types of data that should be protected from exposure? Examples include trade secrets, intellectual property, employment information and government classified documents. These high-level classes of data are ripe for assignments to taxonomies that define the level of sensitivity to the business and in relation to corporate data protection policies. That's aside from those that are directed by regulatory compliance. Alternatively, there may be other aspects of different types of regulations with implied data policies for data handling, data retention or data disposition that also need to be folded into the classification taxonomy.
The implication is four-fold and falls under the realm of the chief data officer:
- First, the CDO is responsible for motivating specification of a data classification taxonomy that lists all classes of data sensitivity and shows how those classifications are associated with defined data protection policies.
- Second, the CDO should institute appropriate processes and technologies for data assessment and classification. This most likely consists of a process for scanning the contents of each data asset and inferring the data policy dependencies inherent in each.
- Third, the CDO must ensure that there's a data catalog designed to capture all of this information and enable search and delivery of the information to both people and automated agents.
- Fourth, it's up to the CDO to make sure there's a process for using technology to automatically validate compliance with different data policies in relation to each data asset – as well as documentation for auditing and reporting.
In essence, regulatory compliance and data protection are great motivators for some critical aspects of data governance. It's beneficial when organizations and their CDOs take on responsibility for instituting the right types of procedures for managing the growing pool of data policies.
Download The SAS Data Governance Framework: A Blueprint for Success