There is no doubt about it – over the past few years there has been a monumental shift in how we think about “enterprise” data management. I believe this shift has been motivated by four factors:
- Open data. What may have been triggered by demands for governmental transparency and the need to make government data sets available to the public has blossomed into a more general acceptance and willingness of all types of organizations to provide access to some of their data sets. One might say the lion’s share of open data remains sourced by government agencies, but integration of open data with internal data presents interesting opportunities for broadening the way reporting and analytics are done.
- Streaming data. In many cases, commercial accessibility to open data is obtained through streaming. Some established examples include news, weather and financial feeds. Social media channels are increasingly becoming the sources of streaming data. There are also a plethora of sensors and controllers that are increasingly networked together, not to mention the millions of mobile devices that are constantly streaming data to centralized servers. In other words, many data sets that are candidates for inclusion in the enterprise rubric originate in other places – different administrative domains.
- The API community. Application development approaches are shifting in reaction to data access patterns, with organizations providing application programming interfaces (APIs) and microservices that enable rapid app development, standardized accessibility to data streams, and incremental upgrades to functionality and features.
- Cloud computing. Hoarding the complete data management infrastructure within your own (often poorly constructed) firewalls is becoming a thing of the past. Virtualized systems running on cloud-based server farms are increasingly hardened with security and data protection, and cost structures make cloud computing an attractive alternative to the conventional data center.
The combination of these factors has created cracks in the traditional firewall that contains what we have referred to as the enterprise. Now, a growing slice of data used by the enterprise either originates, flows around or is stored outside of the enterprise environment. This is what I consider “extra-enterprise data.” To accommodate this type of data, information management must expand beyond the organization's traditional boundaries.
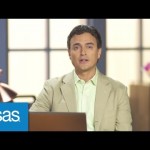
Here are three key questions that need to be considered:
- Management – how does the expansion of data beyond the enterprise change the way corporate data is managed?
- Accessibility – with data sets that are not under your administrative control, what are the best ways to ensure data is accessible and available for your data consumers’ needs?
- Governance – the absence of administrative control means that you also have no control over the conventional dimensions of data quality, such as completeness, accuracy or compliance with business expectations. What types of stewardship and governance can be imposed over extra-enterprise data?
In my next post, we’ll look at these questions in more detail by examining how the emergence of extra-enterprise data creates opportunities to adapt.
2 Comments
Hi David,
Very interesting your post.
I would like to know a bit about SAS and Hadoop integration.
I know that you can connect SAS to Haddop via libname using SAS/ACCESS to Hadoop. As far as I know in that case you interact with Hadoop using HiveQL. You can insert SAS tables in HDFS using hiveQL and you can read Hive tables from SAS.
Other way is using data loader product. I think it also connect with HiveQL
I would like to know how you can do analytics procedures in Hadoop. For example High performance analytics procedures. I have read that this HP procedures can work with Hadoop, but how does it worsks??, is also a connector using HiveQL?, can you execute the procedures in the haddop clusters using map-reduce??, I wnat to know more about thin interaction....if you only gest data from Hadoop or if you can take advantage of hadoop clusters performance executing in a parallel architecture
Other question... SAS Visual Analytics and Hadoop,...I supopose that you can get information from Hadoop (vía HiveQL) and upload to LASR server..is it right??, all the calculations and aggregations are made in LASR not in Hadoop cluster..is it right??
Thank in advance
Many thanks, Juan V., for your comment and great questions. As one of the SAS Support Communities managers, I’m happy to point you to some resources that may help.
Check out a series of articles focused on how SAS Data Management works with Hadoop. Quite a few go into detail on the topics you mention including How to persist native SAS data sets to Hadoop (Hive), How to leverage the Hadoop Distributed File System using the SAS Scalable Performance Data Engine, Server and How to create SAS Scalable Performance Data Engine tables on the Hadoop Distributed File System.
Visit the SAS Data Mining Community for info on high-performance analytics (HPA) as well, and articles focusing on HPA are coming soon as part of the Tip of the Week series.
There’s also a community devoted to SAS Visual Analytics where Hadoop discussions are addressed. We’ll be working on posting tips on how VA works with Hadoop soon. Feel free to subscribe to the SAS Communities Library for these kinds of articles, and to each of the discussion forums noted above.
I hope this is useful, and that I “see” you on the community.
Anna Brown