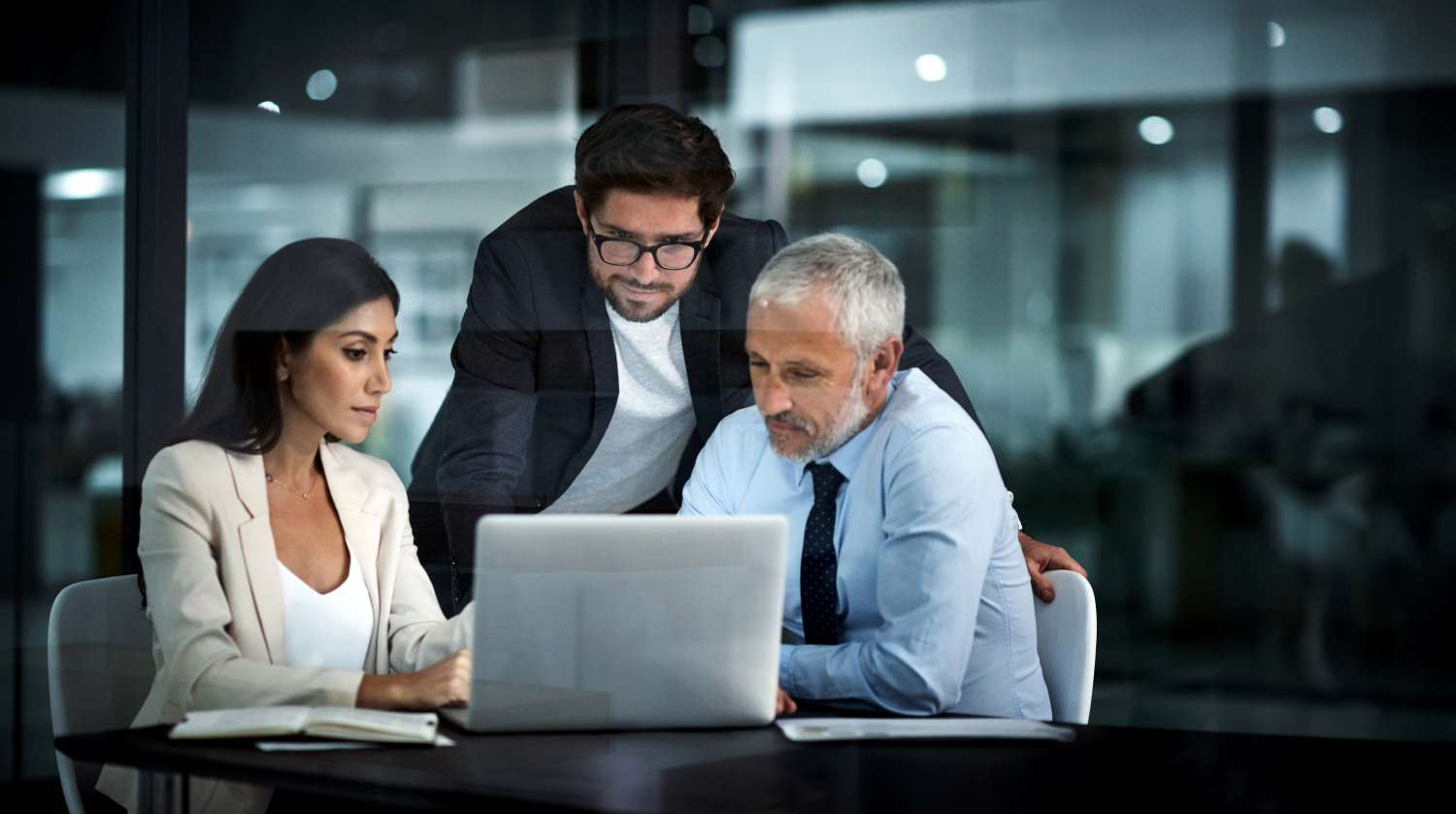
Jim Harris says the question of who owns big data is no longer an esoteric concept – it's a frequently debated topic.
Jim Harris says the question of who owns big data is no longer an esoteric concept – it's a frequently debated topic.
In the movie Big, a 12-year-old boy, after being embarrassed in front of an older girl he was trying to impress by being told he was too short for a carnival ride, puts a coin into an antique arcade fortune teller machine called Zoltar Speaks, makes a wish to be big,
In this blog series, I am exploring if it’s wise to crowdsource data improvement, and if the power of the crowd can enable organizations to incorporate better enterprise data quality practices. In Part 1, I provided a high-level definition of crowdsourcing and explained that while it can be applied to a wide range of projects
In this blog series, I am exploring if it’s wise to crowdsource data improvement, and if the power of the crowd can enable organizations to incorporate better enterprise data quality practices. In Part 1, I provided a high-level definition of crowdsourcing and explained that while it can be applied to a wide range of projects
James Surowiecki wrote a book about The Wisdom of Crowds. Jeff Howe, who co-coined the term crowdsourcing, wrote a book about Why the Power of the Crowd Is Driving the Future of Business. In this blog series, I explore if it’s wise to crowdsource data improvement, and if the power of the crowd can
Since now is the time when we reflect on the past year and make resolutions for next year, in this post I reflect on my Data Roundtable posts from the past year and use them to offer a few New Year’s data resolutions for you and your organization to consider in
As this is the week of Christmas, many, myself included, have Christmas songs stuck in their head. One of these jolly jingles is Santa Claus Is Coming To Town, which includes the line: “He knows if you’ve been bad or good, so be good for goodness sake!” The lyric is a
‘Tis the season. While the season means different things to different people, its most common theme is people buying things for people. Things that become presents when they are covered in wrapping paper. Two retailers have been running television commercial campaigns this season about how presents are wrapped. One campaign
In my previous post I explained that even if your organization does not have anyone with data steward as their official job title, data stewardship plays a crucial role in data governance and data quality. Let’s assume that this has inspired you to formally make data steward an official job title. How
Data. Our industry really loves that word, making it seem like the whole world revolves around it. We certainly enjoy revolving a lot of words around it. We put words like master, big, and meta before it, and words like management, quality, and governance after it. This spins out disciplines
“Correlation does not imply causation” is a saying commonly heard in science and statistics emphasizing that a correlation between two variables does not necessarily imply that one variable causes the other. One example of this is the relationship between rain and umbrellas. People buy more umbrellas when it rains. This
Yesterday was one of the two times a year that an equinox occurs. From its Latin roots, the term equinox translates as equal night since, on the day of an equinox, daytime and night are of approximately equal duration. This occurs because during an equinox the Sun is aligned with the center of the
My previous post pondered the term disestimation, coined by Charles Seife in his book Proofiness: How You’re Being Fooled by the Numbers to warn us about understating or ignoring the uncertainties surrounding a number, mistaking it for a fact instead of the error-prone estimate that it really is. Sometimes this fact appears to
In my previous post Sisyphus didn’t need a fitness tracker, I recommended that you only collect, measure and analyze big data if it helps you make a better decision or change your actions. Unfortunately, it’s difficult to know ahead of time which data will meet that criteria. We often, therefore, collect, measure and analyze
In his pithy style, Seth Godin’s recent blog post Analytics without action said more in 32 words than most posts say in 320 words or most white papers say in 3200 words. (For those counting along, my opening sentence alone used 32 words). Godin’s blog post, in its entirety, stated: “Don’t measure
My previous post explained how confirmation bias can prevent you from behaving like the natural data scientist you like to imagine you are by driving your decision making toward data that confirms your existing beliefs. This post tells the story of another cognitive bias that works against data science. Consider the following scenario: Company-wide
Nowadays we hear a lot about how important it is that we are data-driven in our decision-making. We also hear a lot of criticism aimed at those that are driven more by intuition than data. Like most things in life, however, there’s a big difference between theory and practice. It’s
Many people, myself included, occasionally complain about how noisy big data has made our world. While it is true that big data does broadcast more signal, not just more noise, we are not always able to tell the difference. Sometimes what sounds like meaningless background static is actually a big insight. Other times
In my last three posts on data ethics, I explored a few of the ethical dilemmas in our data-driven world. From examining the ethical practices of free internet service providers to the problem of high-frequency trading, I’ve come to realize the depth and complexity of these issues. Anyone who's aware of these
Imagine if your ability to feed your family depended upon how fast you could run. Imagine the aisles of your grocery store as lanes on a running track. If you can outrun your fellow shoppers, grab food off the shelves and race through the checkout at the finish line, then
In my previous post, I examined ethics in a data-driven world with an example of how Facebook experiments on its users. Acknowledging the conundrum facing users of free services like Facebook, Phil Simon commented that “users and customers aren’t the same thing. Maybe users are there to be, you know... used.” What about when a
I have previously blogged about how the dark side of our mood skews the sentiment analysis of customer feedback negatively since we usually only provide feedback when we have a negative experience with a product or service. Reading only negative reviews from its customers could make a company sad, but could reading only
Data science, as Deepinder Dhingra recently blogged, “is essentially an intersection of math and technology skills.” Individuals with these skills have been labeled data scientists and organizations are competing to hire them. “But what organizations need,” Dhingra explained, “are individuals who, in addition to math and technology, can bring in
Data-driven journalism has driven some of my recent posts. I blogged about turning anecdote into data and how being data-driven means being question-driven. The latter noted the similarity between interviewing people and interviewing data. In this post I want to examine interviewing people about data, especially the data used by people to drive
At the Journalism Interactive 2014 conference, Derek Willis spoke about interviewing data, his advice for becoming a data-driven journalist. “The bulk of the skills involved in interviewing people and interviewing data are actually pretty similar,” Willis explained. “We want to get to know it a little bit. We want to figure
In physics, antimatter has the same mass, but opposite charge, of matter. Collisions between matter and antimatter lead to the annihilation of both, the end result of which is a release of energy available to do work. In this blog series, I will use antimatter as a metaphor for a factor
My previous post made the point that it’s not a matter of whether it is good for you to use samples, but how good the sample you are using is. The comments on that post raised two different, and valid, perspectives about sampling. These viewpoints reflected two different use cases for data,
In my previous post, I discussed sampling error (i.e., when a randomly chosen sample doesn’t reflect the underlying population, aka margin of error) and sampling bias (i.e., when the sample isn’t randomly chosen at all), both of which big data advocates often claim can, and should, be overcome by using all the data. In this
In his recent Financial Times article, Tim Harford explained the big data that interests many companies is what we might call found data – the digital exhaust from our web searches, our status updates on social networks, our credit card purchases and our mobile devices pinging the nearest cellular or WiFi network.
As an unabashed lover of data, I am thrilled to be living and working in our increasingly data-constructed world. One new type of data analysis eliciting strong emotional reactions these days is the sentiment analysis of the directly digitized feedback from customers provided via their online reviews, emails, voicemails, text messages and social networking