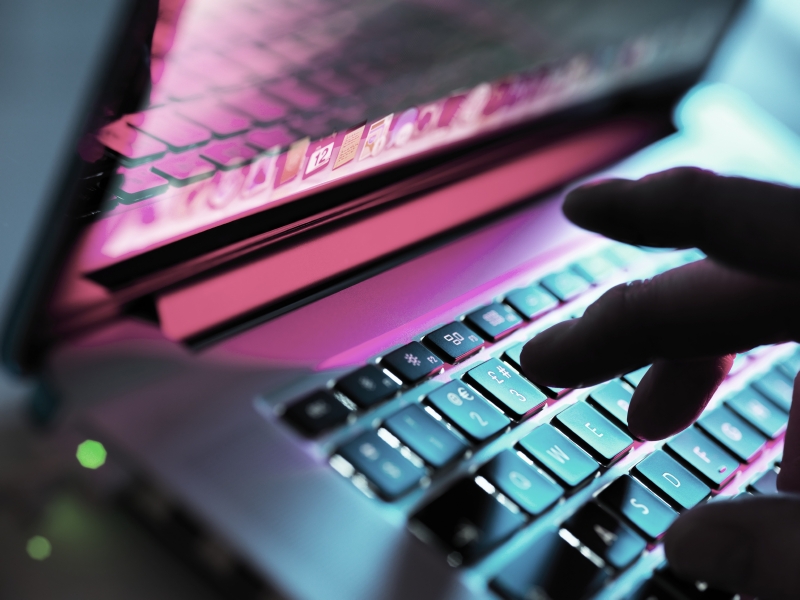
Modernization is a term used to describe the necessary evolution of information technologies that organizations rely on to remain competitive in today’s constantly changing business world. New technologies – many designed to better leverage big data – challenge existing data infrastructures and business models. This forces enterprises to modernize their approach to data