We live in a complex world that overflows with information. As human beings, we are very good at navigating this maze, where different types of input hit us from every possible direction. Without really thinking about it, we take in the inputs, evaluate the new information, combine it with our experience and previous knowledge, and then make decisions (hopefully, good informed decisions). If you think about the process and types of information (data) we use, you quickly realize that most of information we are exposed to contains a spatial component (a geographical location), and our decisions often include neighborhood effects. Are you shopping for a new house? In the process of choosing the right one, you will certainly consider its location, neighboring locations, schools, road infrastructure, distance from work, store accessibility, and many other inputs (Figure 1). Going on a vacation abroad? Visiting a small country with a low population will probably be very different from visiting a popular destination surrounded by densely populated larger countries. All these are examples that illustrate the value of econometric and statistical methods for spatial data analysis.
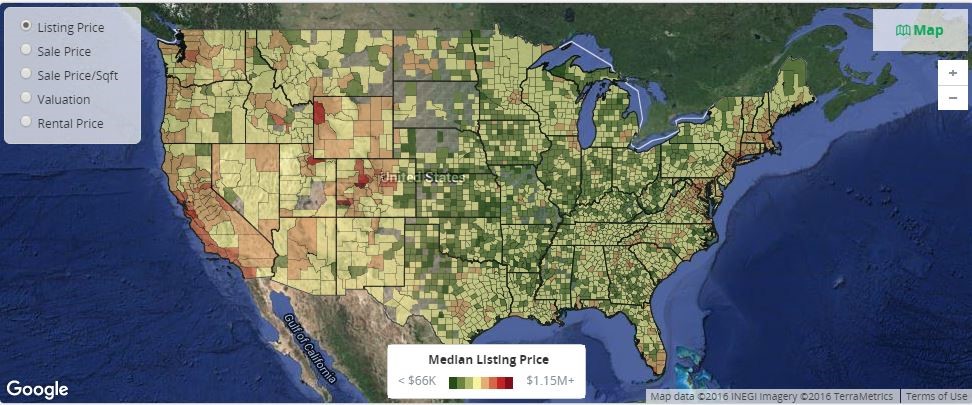
We are all exposed to spatial data, which we use in our daily lives almost without thinking about it. Not until recently have spatial data become popular in formal econometric and statistical analysis. Geographical information systems (GIS) have been around since the early 1960s, but they were expensive and not readily available until recently. Today every smart phone has a GPS, cars have tracking devices showing their locations, and positioning devices are used in many areas including aviation, transportation, and science. Great progress has also been made in surveying, mapping, and recording geographical information in recent years. Do you want to know the latitude and longitude of your house? Today that information might not be much further away than typing your address into a search engine.
Thanks to technological advancement, spatial data are now only a mouse-click away. Though their variety and volume might vary, data of interest for econometric and statistical methods for spatial data analysis can be divided into three categories: spatial point-referenced data, spatial point-pattern data, and spatial areal data. The widespread use of spatial data has put spatial methodology and analysis front and center. Currently, SAS enables you to analyze spatial point-referenced data and spatial point-pattern data with the KRIGE2D and VARIOGRAM procedures and spatial point-pattern data with the SPP procedure, all of which are in SAS/STAT. The next release of SAS/ETS (version 14.2) will include a new SPATIALREG procedure that has been developed for analyzing spatial areal data. This type of data is the focus of spatial econometrics.
Spatial econometrics was developed in the 1970’s in response to the need for a new methodological foundation for regional and urban econometric models. At the core of this new methodology are the principles on which modern spatial econometrics is based. These principles essentially deal with two main spatial aspects of the data: spatial dependence and spatial heterogeneity. Simply put, spatial econometrics concentrates on accounting for spatial dependence and heterogeneity in the data under the regression setting. This is important because the ignorance of spatial dependence and heterogeneity could lead to biased/inefficient parameter estimates or flawed inference. Unlike standard econometric models, spatial econometric models do not assume that observations are independent. In addition, the quantification of spatial dependence and heterogeneity is often characterized by the proximity of two regions, which is represented by a spatial weights matrix in spatial econometrics. The idea behind such quantification resonates with the first law of geography—“Everything is related to everything else, but near things are more related than distant things.”
In spatial econometric modeling, the key challenge often centers on how to choose a model that well describes the data at hand. As a general guideline, model specification often starts with understanding where spatial dependence and heterogeneity come from, which is often problem-specific. Some examples of such problems are pricing policies in marketing research, land use in agricultural economics, and housing prices in real estate economics. As an example, it is likely that car sales from one auto dealership might depend on sales from a nearby dealership either because the two dealerships compete for the same customers or because of some form of unobserved heterogeneity common to both dealerships. Based on this understanding, you proceed with a particular model that is capable of addressing the spatial dependence and heterogeneity that the data exhibit. Following that, you revise the model until you identify one that meets certain criteria, such as Akaike’s information criterion (AIC) or the Schwarz-Bayes criterion (SBC). Three types of interaction contribute to spatial dependence and heterogeneity: exogenous interaction, endogenous interaction, and interaction among the error terms. Among a wide range of spatial econometric models, some are known to be good for one type of interaction effect, whereas others are good for other alternatives. If you don’t choose your model properly, your analysis will provide false assurance and flawed inference about the underlying data.
In the next blog post, we’ll talk more about econometric and statistical methods for spatial data analysis by discussing spatial econometric analysis that uses the SPATIALREG procedure. In particular, we’ll discuss some useful features in the SPATIALREG procedure (such as parameter estimation, hypothesis testing, and model selection), and we’ll demonstrate these features by analyzing a real-world example. In the meantime, you can also read more in our 2016 SAS Global Forum paper, How Do My Neighbors Affect Me? SAS/ETS® Methods for Spatial Econometric Modeling.