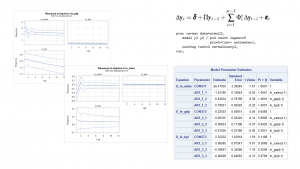
Macroeconometrics is not dead: (and I wish I had paid better attention in my time series course):
I wrote this on the way to see one of our manufacturing clients in Austin, Texas, anticipating a discussion how to use vector autoregressive models in process control. It is a typical use case, especially in the age of the “Internet of Things,” to use multiple sensors on a device to detect mechanical issues early. Industrial engineers often use rolling window principal component analysis or a dynamic factor model to detect trends. These are fairly common applications. In my customer engagements, these approaches had been the primary applications of multivariate time series methods. As an applied microeconomist, I believed that my encounters confirmed my decision during graduate school to shirk time series econometric courses and instead focus on cross-sectional and panel data econometrics. Until I visited a banking customer in Dallas this fall....
See, for the past several years, the banking industry has been busy hiring quants for a regulatory overhaul called CCAR/DFAST. Quantitative analysts are being employed to estimate various forms of loss models to meet CCAR/DFAST regulations, which require various economic scenarios to be factors in these loss models. Some of these models resemble cross-sectional models and some of these autoregressive time series models, but both of these models are univariate in nature. My colleague Christian Macaro and I wrote a paper, "Incorporating External Economic Scenarios into Your CCAR Stress Testing Routines," that provides an overview of both methods. Although some of these models “could” use multivariate vector autoregressive models, as my buddy Rajesh Selukar, developer of state space models at SAS (PROC SSM) says, ”If you don’t have to use multivariate time series methods, don’t use them,” conveying the complexity of modeling these interactions. Up to this point, my shirking had paid off. No PROC VARMAX needed.
Then came my trip to Dallas. At this bank I met a new type of CCAR/DFAST team, whose mission is to create macroeconomic forecasts of the US and international economies to be consumed by CCAR/DFAST modeling teams. Until now, most CCAR/DFAST economic scenarios have been generated by either the Federal Reserve or by one of several small consulting firms specializing in this analysis. These groups have long used complicated multivariate techniques such as dynamic stochastic general equilibrium (DSGE) or vector autoregressive models (VAR), but they tend to use smaller niche software tools in their consulting work. This new internal economic scenario team was charged with bringing economic scenario generation in house. I had heard that the Fed has become increasingly critical of relying on purchased forecasts. Tier 1 banks are now being required to generate these forecasts on their own, and the customer I met with is one of these banks.
Well darn. I guess I should have paid more attention to Dr. Ali’s ECO703 course! One of these days I will get back to the basics of multivariate time series analytics. After all, PROC SSM and VARMAX are two procedures in SAS/ETS® that work with multivariate time series models. In fact, when I get around to it, I will likely utilize a new book by Anders Milhøj, Multiple Time Series Modeling Using the SAS VARMAX Procedure, which provides both a theoretical and practical introduction to VAR models in SAS. If you already know a bit about multivariate time series and just wanted to get started with SAS, try the example programs with the VARMAX and SSM procedures and SAS Studio using free access to SAS OnDemand for Academics, whether you are a student, professor, or independent learner.