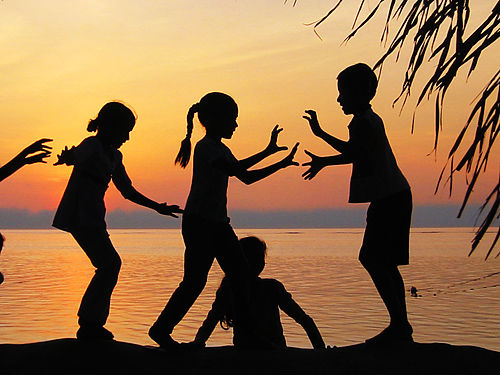
Flickr image by ann_jutatip
In my last blog post, I introduced common concerns I’ve heard about predictive analytics in child well-being efforts. In this post, I want to address those concerns and reassure leaders and advocates that predictive analytics can be a tremendous boon to our ability to help kids and ease the burden on caseworkers.
They concerns I hear most are about:
- False Positives
- Racial Bias/Disparity
- Equation vs. Comprehensive Process
- Current Tools are Sufficient
Concern: Child well-being predictive analytic solutions may result in a high number of false positives, giving workers added work.
This concern is misguided. Sophisticated analytics, like SAS for Child Safety, actually has identified less than 5% of overall cases as being at the highest level of risk of maltreatment and/or fatality. Current validated actuarial risk and needs assessment have routinely identified from 25-30% of cases as being highest risk. This increases the potential amount of false positives up to 500%.
Child safety models are built on a multitude of risk factors, many of which vary in intensity (not just Yes/No binary values). These risk factors are intuitive. The literature and common sense would agree on the peril a child is exposed to given extreme values of these risk factors, such as:
- Scores of prior maltreatment allegations by child’s primary caregivers
- Multiple victimization events in caretaker’s childhood
- Low maternal age
To be in the highest risk segments, a child needs to have extreme values in a multitude of risk factors (not just one). In such an environment, risk of general harm to a child is also elevated, not just risk of fatality.
Fatality modeling is more narrowly focused. For each fatality there may be dozens or more near misses (injuries but not fatalities). However, data for serious near misses is not readily available. As terrible as it may be, a child who ends up in the ICU due to abuse is considered a false positive for a fatality risk model.
We have to remember that risk to child well-being is ongoing. In our research at SAS, we have observed children in extreme risk segments at the end of a study period that eventually become fatalities. That said, risk can also decrease over time as, for instance, a child’s age increases or there are changes in household composition.
Concern: Predictive analytics may attribute to racial bias and disparity within communities.
Racial disparity is not apparent in fatality risk. Literature and SAS’s own modeling in multiple jurisdictions has found no significant difference in maltreatment and/or fatality risk for black versus white households. Of some interest was the fact that Hispanic households had a slightly lower fatality risk.
The fact is, race is vastly eclipsed by other risk factors like prior maltreatment allegations, making racial factors of little value in risk models.
Concern: Analytics is "just" a mathematical equation, no a comprehensive process
Analytics actually IS a comprehensive process that helps generate a “golden record” of an individual. The child well-being models that support these efforts are based on historical data multiple factors and outcomes, such as fatalities, maltreatment, permanency, homelessness, etc. More accurate and complete data is modeled, allowing for workers to be more confident and proactive.
Collections of various factors determine risk, not individual facts or flags. This eliminates the one-size-fits-all approach to case response (e.g. children under 5 are high risk and require a full investigation), which is not proven out by the risk model. A high risk score means there is a multitude of simultaneous risk factors present. The risk score brings this to the attention of the case worker to help inform decisions, coupled with their professional judgement.
Concern: Current actuarial tools are sufficient, so why use analytics?
Case workers are also analytic models (biological not mathematical) and in general, not very good ones. This is not because they aren’t dedicated, intelligent professionals, of course. However, judgement or consensus decision making is shown in the literature to underperform formal actuarial methods.
This is due to a number of factors, including high caseworker turnover rates and the associated variances in caseworker experience. Caseworkers can introduce bias in the scoring process when using actuarial methods like Structured Decision Making. In more extreme situations, there are examples of caseworkers manipulating actuarial tools to force certain actions like a home removal.
All of this could be helped by better information, but there’s a problem there, too. Caseworkers too often are provided with incorrect and/or overwhelming data. A combination of poor data quality and an inability to sift through what’s relevant and not amid an avalanche of data hinders decision-making.
Operational analytic models work across the risk spectrum and can also assist in screening and triaging cases so they can be routed based on available data. In addition, data from multiple agencies can better inform the model and resulting decisions. Additional data is especially important in creating more accurate risk assessments in situations with limited report histories for a child.
The debate over analytics, actuarial tools and basic human judgement will continue, and it should. We should all work towards finding the balance of approaches that gives us the best chance to help kids. That said, I hope I’ve alleviated some of the more common concerns and look forward to continuing the discussion in the comments section.