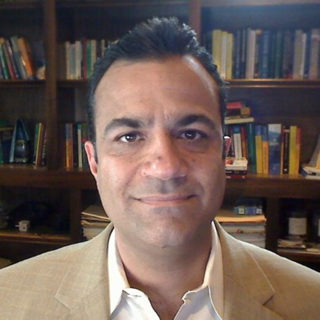
This guest post was written by Zubin Dowlaty. He has 20+ years’ experience in the business intelligence and analytics space. At Mu Sigma, he works closely with Fortune 500 companies counseling them on how to institutionalize data-driven decision-making. Zubin is focusing his efforts managing an agenda of rapidly implementing innovative analytics technology and statistical techniques into the Mu Sigma ecosystem.
There is an old adage that goes, “don’t put all your eggs in one basket” and for those that like financial advice, the only free lunch is diversification. The spirit of these ideas emphasizes risk reduction with a behavior change. The idea of minimizing risk to interpret and generalize analytical models is not new. Reducing the risk of over-fitting and methods such as bootstrapping are utilized to reduce risk. However, the idea of diversification tends to be underutilized in analytics workflow.
In the big data space, we are witnessing a trend towards NoSQL technologies, where we have multiple tools and frameworks to access data at our disposal. For the data analyst, prepping data for modeling consumes a tremendous amount of time. Anecdotal estimates are usually between 60%-80% of the data scientist’s time allocated to preparing the ‘model' ready data set.
Why then, when we complete the data prep, most analysts will estimate OLS regression models and stop? If that’s not the case, then only one modeling technique will be selected. The analyst will then interpret, refine the model and present results. At least in corporate America there is a clear bias towards running only one technique. This one model bias clearly goes against the spirit of minimizing risk by utilizing a portfolio approach. Ensembles, the technical term for running multiple models, should be the default method not the former.
Design thinking and design principles are beginning to be taught in major graduate business schools. One of the major principles of design thinking is prototyping and ideation. Furthermore, the ensemble approach leads to the champion model of a natural ‘po’ concept, which translates to a provocation. Design concepts are also in alignment with the ensemble approach, especially for measurement and forecasting use cases. One should explore and provoke the ‘champion’ model.
Let’s say you have selected an OLS model to be your champion. One should challenge this model by running a portfolio, or ensemble of methods to improve insight and generalization. Given the various assumptions around robustness, functional form, structural change within our data, it can be very risky to estimate one model. The idea is not to run various models like in the traditional ensemble sense, but rather to aggregate the various models in some form to get a better predictor. Again, it would be ideal to run an ensemble, leveraging the advantages of robustness, variable importance, and functional form in other techniques, in order to improve your dominant champion model, not replace it.
With today’s computational resources, the marginal time and cost of running many models are near zero – there is no longer any excuse. The bottom line, it’s a mindset change.
Join me in a discussion of these ideas at the Analytics 2014 conference. We will review over a dozen models used in a business use case, in order to harden the champion model. We will demonstrate how the ensemble approach to improving your champion model can significantly improve interpretability as well as trust in your model outcomes.