Computer vision is a field of artificial intelligence that teaches computers to understand visuals. Using digital images from cameras and videos and deep learning models, machines can learn to recognize and categorize objects and respond to their surroundings based on what they “see.”
Computer vision's accuracy has skyrocketed in the last ten years — far surpassing human capabilities. This, combined with hardware advances, made computer vision a viable option for industrial applications. For instance, manufacturers can see a hefty financial boost from minor improvements to manual inspections, processes and automation.
Consider Georgia-Pacific, a leading manufacturer of tissue, pulp, packaging and building products. Georgia-Pacific produces many popular name brands, including Quilted Northern, Brawny, Dixie and Vanity Fair. We recently talked to Sam Coyne, Georgia-Pacific’s Senior Director of AI, to understand how his team uses computer vision, why he and his team chose SAS and the other technologies they use for artificial intelligence.
Q: Sam, can you tell us what computer vision is to you and your team?
Sam Coyne: Computer vision is essentially another set of eyes. It mimics what our brains do: process images and recognize patterns. Computer vision can reliably detect issues that are anomalous or predictive of future behavior.
Q: What are some benefits of computer vision for manufacturers?
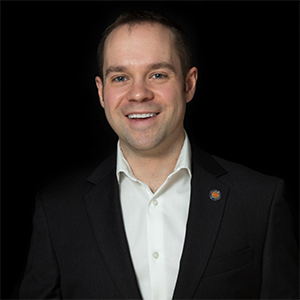
Coyne: Computer vision is a great solution for monitoring various parts of a process. Whereas an individual may not want to monitor the same mundane task for eight hours, a computer equipped with a camera and embedded models can look for abnormalities around the clock without fatigue. Then, our human resources can focus on higher-value tasks.
For example, let’s say the human operator is alerted that this step in the process is stopped due to wet materials. Machine learning models can then recalculate the next-best step on the fly to save the products from being downgraded or scrapped.
Computer vision is about augmenting your workforce with tools they can use to be more efficient and effective.
Q: What are some use cases that computer vision is helping Georgia-Pacific solve?
Coyne: Computer vision has helped us solve problems with quality and safety — the two most important things you can keep an eye on in manufacturing. For example:
- Computer vision alerts us when parts show signs of wear so we can repair them during planned maintenance, making our techs safer and more efficient.
- We use computer vision models, deep learning models, and various neural networks to detect if someone is in a “no-fly zone,” which is a restricted area.
- Computer vision models are used to monitor off-quality products as they come down conveyor systems, alert an operator, and auto-reject the materials.
Q: How are you integrating computer vision models into your current business processes?
Coyne: As with all the other analytics we use, computer vision is embedded in the day-to-day operations of our facilities. On the support side, which is our group, we plan to deploy these models into production to run 24/7, 365 days a year, to highlight and capture anomalous behavior.
Q: Can you speak to the time-to-value aspects of a computer vision use case?
Coyne: We put a premium on time-to-value at Georgia-Pacific’s Collaboration and Support Center. Having said that, our approach is iterative. Rather than driving immediately toward perfection, we deploy smaller iterations of a project so stakeholders can interact and provide feedback. We focus on experimental discovery and maturing the product over time to become part of day-to-day operations.
Q: Computer vision is often associated with edge computing. How important is it to process computer vision workloads at the edge?
Coyne: Computer vision at the edge is critically important to our facilities. When you think about our two primary use cases — quality and safety — we need real-time information so that we can take immediate action. Another benefit of computer vision at the edge is that when there are connectivity issues (many manufacturing facilities are in remote locations where high-speed connectivity isn’t reliable or cost-effective), the model can still execute and provide the 24/7 coverage we need in a closed-loop system.
Q: What technology considerations should be top-of-mind for manufacturers considering adding computer vision to their operations?
Coyne: There are a couple of considerations; the first is that it’s not a decision of open source or SAS. We are using the best of both worlds. SAS is very open, especially in its streaming capabilities. You can use open-source Python and the resiliency of SAS® Event Stream Processing to ensure your models are always running. Regardless of the technology you choose, the most important consideration is to have the infrastructure and architecture set up to ensure an end-to-end solution (not just writing Python).
When thinking about what efforts to take on, think outside the box. We’re all familiar with PPE detection and facial recognition, but there’s so much more. Also, not all computer vision use cases are created equal, so focus on high-value projects first.
Q: Why did you choose SAS?
What really differentiated SAS for us was its ability to integrate two worlds. There’s the Python world with new libraries coming out daily, if not hourly. And then there’s the SAS world, which gives you the structure you need to be successful with deployment and keeping this running in production. And with that structure comes resiliency, sound architecture, and confidence in a stable platform, which is critically important for us as manufacturers.
SAS also makes it easy to manage workloads from a central location, improving uptime regardless of connectivity and making it easier to manage the full analytics lifecycle. Finally, using SAS and ESP has helped us keep an eye on the health of our models, which drives a ton of value in the end.