For nearly five years, Anupam Trivedi, Senior Director of Credit Control Services at HSBC, has been on a journey to modernize the bank's decision ecosystem for retail credit lending.
Supporting lending decisions for the largest bank in Europe is no simple feat. Overall, the HSBC system processes lending decisions for $12 billion in financial transactions per year. It also supports more than 300 credit strategies, 200 production changes a month, 400 users and runs across more than three global data centers. Trivedi shared his experiences at SAS Innovate in Orlando.
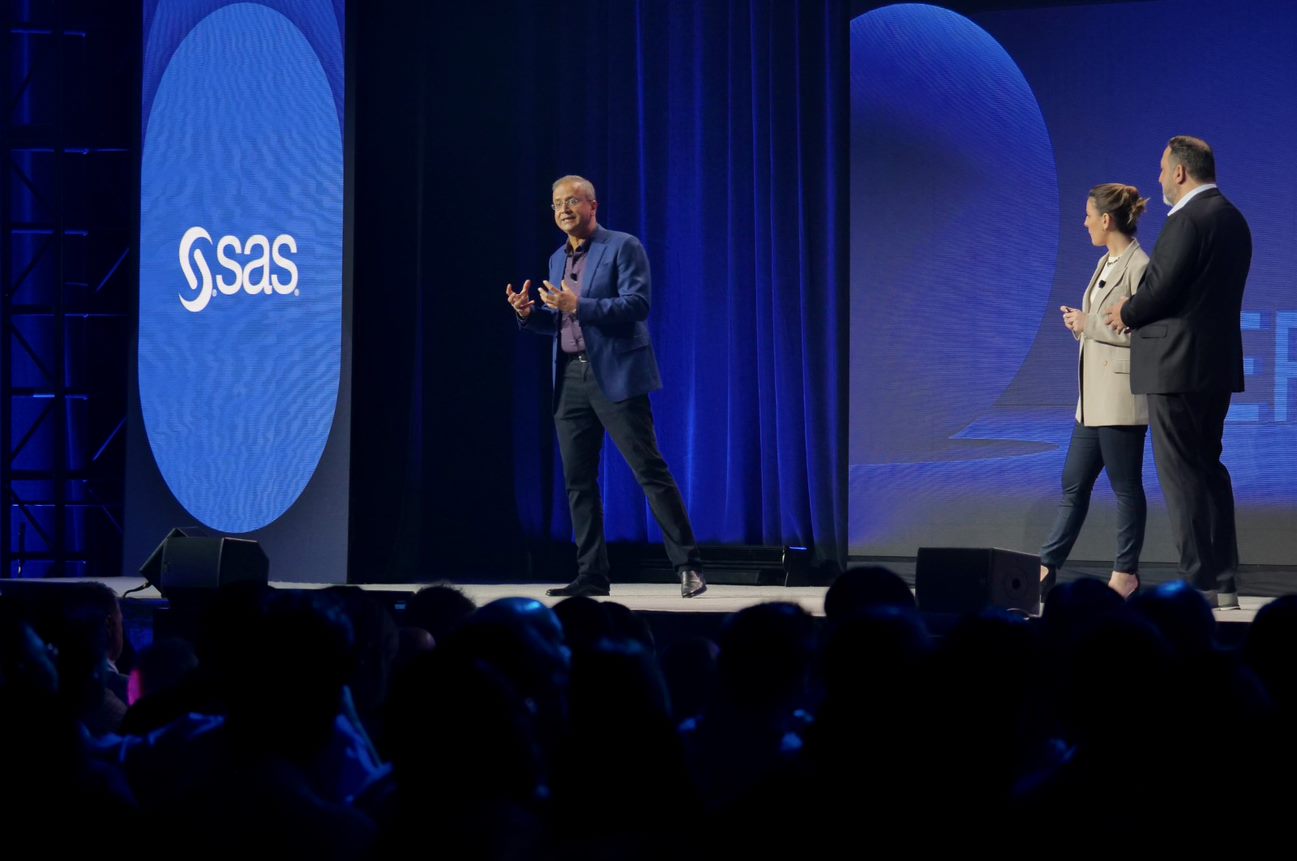
Five years ago, Trivedi’s team set out to rebuild the HSBC credit decision ecosystem to overcome four major challenges:
- Limited data: We very quickly saw that the data we were using for a particular customer was very limited, said Trivedi. They looked at transactional and application data, and information for the credit bureau.
- Rudimentary analytics: “We were using basic regression models, traditional hypothesis-driven modeling, and a large number of manually created rules that overruled the models.”
- Diverse users: Multiple teams with varied skills and fragmented platforms all relied on the system.
- Outdated technology: Many systems within the ecosystem were at end-of-life states, said Trivedi.
“Nobody had touched this ecosystem for a long time,” said Trivedi, “Because it was so critical to the organization and it was used at such a large scale.” But, he said, the aging system components triggered the start of the journey to something new.
To get further buy-in on the upgrades, Trivedi’s team ran several proofs of concept to test whether they could improve outcomes by adding new data and more advanced models.
“We showed we could improve the return on our lending decisions by more than 20 percent,” said Trivedi. “If you multiply that across the amount of transactions completed, that’s a lot of potential untapped value.”
Partnering with SAS for modernization
When determining whether to upgrade or replace the system, the team looked at:
- Existing partners: The types of changes weren’t on their roadmaps, said Trivedi.
- Fintechs: “They do great work but could not scale to our ecosystem,” according to Trivedi.
- Big tech: These companies had many of the capabilities, but they weren’t fully commercialized, explained Trivedi, and “We weren’t sure they would commit for long term.”
- Open source: Trivedi found some affordable options here, but he said it would have been development intensive and did not provide the guardrails needed for the scale of decisions at HSBC.
Trivedi knew he still needed the new system to support native open-source analytics for coding but not execution. The new system also had to consume large data volumes and support advanced models and model deployment.
“We were working with SAS already but didn’t know they had a product in place that had these capabilities,” said Trivedi. When he met with SAS, he found out the SAS products met most of the capabilities he needed, and he felt certain that SAS R&D could develop the rest.
“We gained a high degree of confidence in SAS R&D capabilities and a confidence in our ability to get what we wanted, even though some of it was not what the product had at that time.”
It's important to realize that “An RFP is a point in time,” said Trivedi. “The most important thing was finding the partner we wanted to be with for a long time.”
Reaching ROI even before finishing implementation
Today, HSBC has been successfully running the new environment for four years with impressive results and no production challenges. “One-third of the way through implementing the program, we succeeded with reaching business case value,” said Trivedi.
Since the risk-adjusted return of 20 percent calculated during the proof of concept only occurred with new models, Trivedi’s team prioritized rewriting models that would provide the most impact. “We rewrite for value and lift and shift for speed,” he said.
Another crucial piece of the modernization is a “get decision API” that Trivedi said insulated the ecosystem from change. “All calling systems call one API, so we can change the back-end without the calling systems knowing anything was changing. That was the primary approach that caused little disruption.”
Today, the data and decision system can perform at scale across markets. It offers customer-level decision management across all credit journeys. Additional positive results include:
- A higher rate of positive transaction authorizations.
- New probabilistic models instead of deterministic application logic.
- Faster adoption and replacement of technologies as they evolve.
- Driving convergence between credit and fraud spaces.
Many of the new opportunities the team sees today would not have been visible to them four years ago, adds Trivedi. And he expects the successes to continue, especially as the team begins to collaborate more between fraud and credit teams.