Editor's note: This blog post is part of a series of posts, originally published here by our partner News Literacy Project, exploring the role of data in understanding our world.
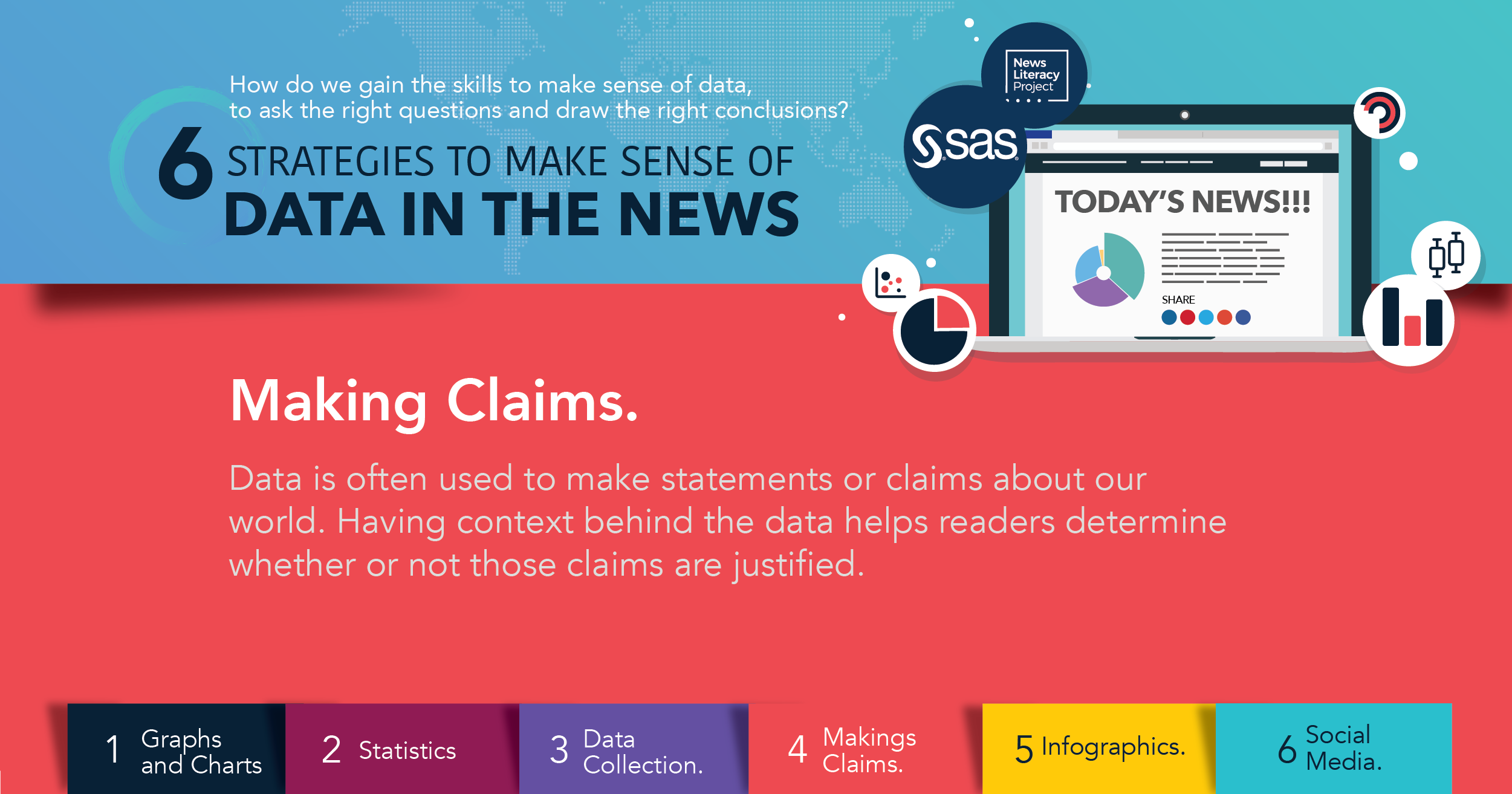
Every day people use data to better understand the world. This helps them make decisions and measure impacts. But how do we take raw numbers and turn them into information that we can easily understand?
We make claims, or statements, about what we think the data tells us. And we often get our information from what the media report about data -- and authors use data to support arguments or inform the public. However, the claims may depend heavily on how and what type of data was collected. We discussed some issues with data collection in the last post, but let’s go into more detail about issues that may arise when authors use data to build arguments or make claims.
Control or comparison groups
People often use data to draw comparisons between different types of groups or behaviors. It’s important to pay attention to the questions asked and who is being asked. And, it’s equally important to ask what researchers are comparing their claims against.
Imagine we poll people and find that 60% say green is their favorite color and they eat vegetables every day. We could claim that liking the color green means a person is more likely to eat vegetables. While the survey indicates that most people who like the color green eat vegetables daily, it’s hard to draw further conclusions without a comparison group.
If only 20% of people who don’t like the color green eat their vegetables, this might suggest an interesting relationship. However, it might also be true that 60% of people who don’t like green eat their vegetables – the exact same proportion. In this case, liking the color green has absolutely nothing to do with vegetable consumption. You might also find that 90% of people who don’t like the color green eat vegetables daily. This shows a negative relationship between liking the color green and eating vegetables daily.
As you can see, if an author presents only one number without comparison, interpreting the true value of the statement can be difficult. We need to compare that number against another group to get a better picture of its significance.
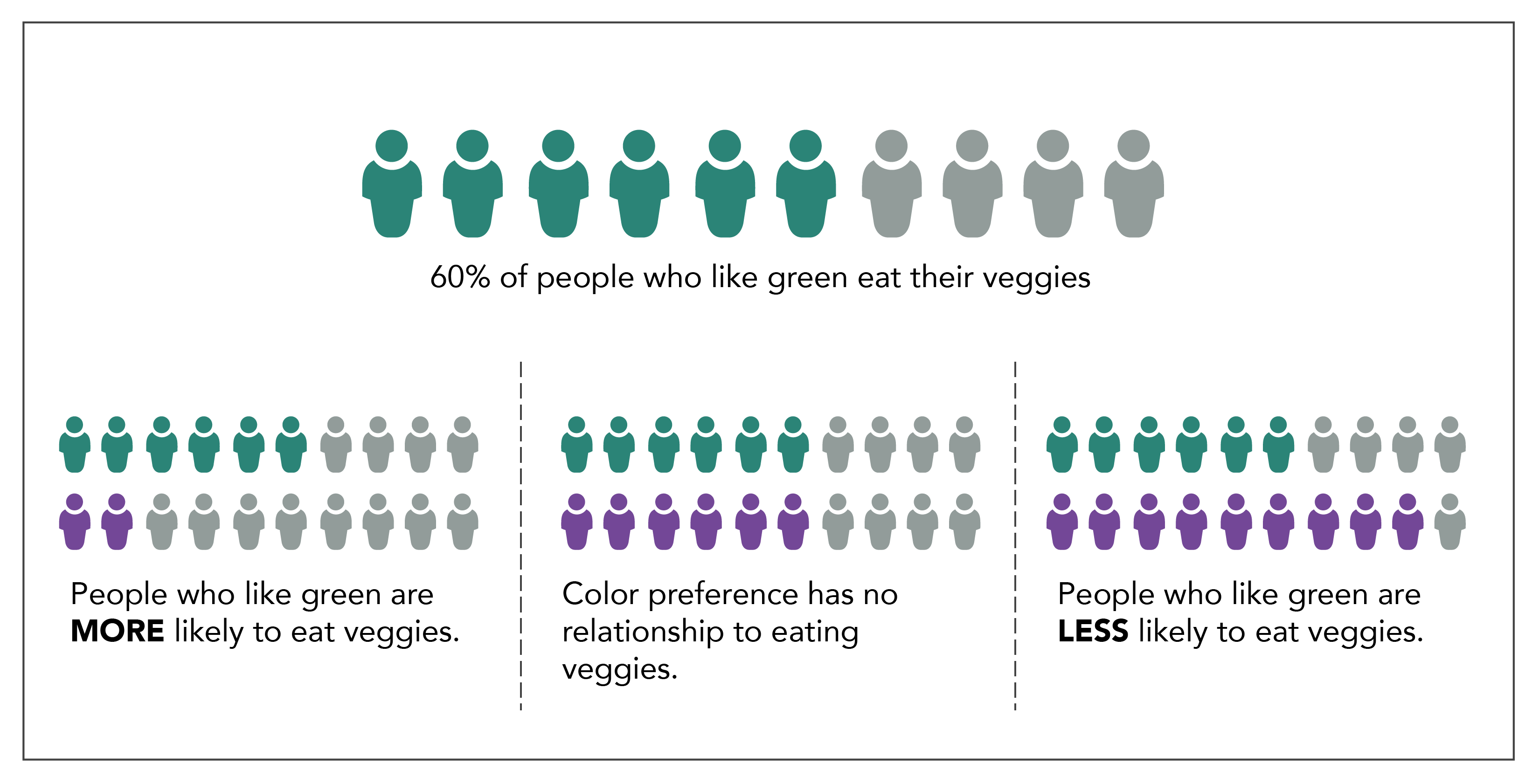
Interventional studies
This is especially important when researchers conduct interventional studies in areas such as medicine, fitness, food and other areas to investigate a particular outcome. In these cases, researchers use a control group. This is a special population that serves as a comparison and does not receive whatever is being tested. Designing an appropriate control group can be tricky because things can change simply because the group is aware it's being observed.
Imagine we conduct a trial for a new weight loss drug. Participants are split in half. One group receives the new drug and the other group receives nothing. Our goal is to determine which group loses the most weight. It may be that the people who receive nothing realize that the situation remains the same and they're not receiving treatment. It’s unclear how this might affect their behavior. Similarly, the people taking the drug assume something might happen, and perhaps this affects their attitude and behavior. We can’t tell if the drug had the impact or if the act of taking a pill changed their behavior. For this reason, many studies include a placebo, a pill that doesn’t contain any chemicals at all, as part of the comparison. This works well especially if researcher and participants do not know who gets the placebo and who gets the real drug.
More challenging studies
This can be much harder in studies that don’t involve medication. Perhaps my intervention is an exercise program. How can I design a fair control group? Do I give my control group advice to not exercise? Do I ask them to do exercise that is similar to my program but not exactly the same? Each of these decisions directly impacts the comparisons I might be able to make and how I will communicate results.
When reading such study data, it’s important to look at the actions of both the experimental group and the control group. Ask yourself if the comparison seems fair, or if it seems as though one group had an advantage or disadvantage. What other factors might explain the results you see?
Bias in research
Finally, why the studies are being conducted and who is interested in the research are of particular concern in data and research studies. Specifically, organizations collecting data on their own products may be motivated to prove those products work or that people like them. They may unintentionally make decisions in designing their research questions or comparison groups that increase the chances they get the results they’d like to see. Researchers who receive money from a specific organization may also make decisions that favor the group that funded their research. As we’ve already learned, there are many complicated decisions that go into collecting data that will inevitably impact the quality of the final result, so it’s important to critically analyze these choices.
While data allows us to measure and better understand our world, it's not a perfect representation of reality. There are many opportunities for bias or flaws when collecting data that can impact the quality of the results. Critical thinking is the best way to find these biases or flaws when reading about data. Ask how the data was collected and from whom. Look for who was included and who was left out. If there is something that seems impressive, ask “compared to what?” To gather those answers, you may have to do some digging of your own, but it will help you determine what data you can trust and recognize data that may be too biased or flawed and should be disregarded.