The dramatic drop in crude oil prices from the highs above $100USD per barrel down to levels around $50 per barrel is one of the drivers behind the industry’s march towards improved operating efficiency. Along the corridors of Independent Oil Companies (IOCs) and National Oil Companies (NOCs), machine learning and deep learning methodologies are the buzz, as analysts seek new approaches to enhance the traditional interpretation of upstream data.
New analytical approaches alone will not accelerate the rebound from low oil prices. Complex global and local influences combined with too much supply and weak global demand play into a dynamic scenario. Therefore, oil and gas companies are also investing in new resource development strategies to balance market volatility. It is imperative for the upstream players to walk gingerly. As the markets react to daily influences on the price of oil, gliding up and then falling, executives will piecemeal realign to more aggressive and competitive price structures to enhance profit margins. These activities require a robust analytics platform to manage and optimize the transition to more efficient operations.
When I visit SAS oil and gas customers all around the globe, senior executives note that is it harder and harder to retain talented geoscientists. Once the lifeblood of this fascinating industry, geoscientists are becoming a rare breed. As a result, organizational systems and processes must be redesigned to incorporate more digital capabilities that augment a flexible staffing environment and take advantage of new data sources coming from digital oilfield technologies. The Industrial Internet of Things (IIoT) and the connectivity of data streaming from sensors, via historians to dynamic soft computing models, can predict and prescribe solutions for a multitude of business problems: these are the life jackets to survive current and future industry downturns.
How do we derive actionable knowledge from raw data sets? Figure 1 illustrates a road map for moving from raw data to distributed intelligence for upstream oil companies.
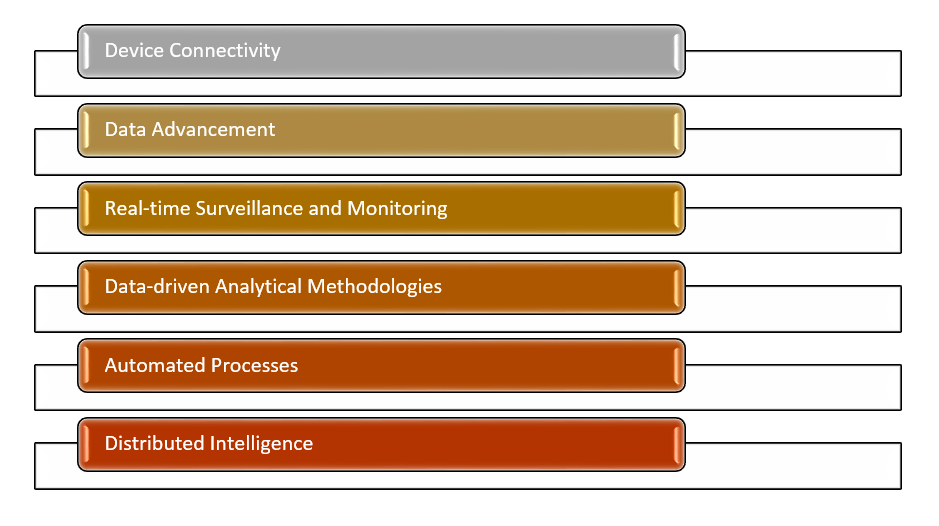
The process is based on defined stages that logically link to form an iterative and closed loop IIoT methodology to address current business problems in the upstream. Adopting this methodology enables oil and gas organizations to operationalize IIoT as an enterprise solution that matures and reflects changes in corporate behavior, maximizing upstream ROI and optimizing production across all asset portfolios.
One specific application of this IIoT methodolgy is to help oil and gas companies reach target production levels while minimizing costs. Operators and oilfield service companies have initiated IIoT strategies in particular sectors of their daily operations. They tend to embrace a bottom-up approach to deliver an efficient and substantial Return On Investment (ROI) for a focused business area. However, oil and gas operators harbor a doubting viewpoint fueled by the uncertainty behind the integration of digitalization for maximum business impact.
Owing to a confluence of events, a real digital transformation in the oil and gas industry is now feasible. The fruitful acceptance of IIoT-enabled data-driven analytics in multiple vertical spaces has fashioned a belief of comparable results in the E&P arena. Continuing advances in cloud-based storage, data transmission, real-time event stream processing and computing power deliver cost reductions in implemented IIoT architectures.
The following SPE papers provide more insights on the application of analytics for production optimization, including IIoT methologies:
- Data Mining Methodologies enhance Probabilistic Well Forecasting: SPE 167428
- Automating Decline Curve Analysis in an Integrated Reservoir Management Portal: SPE 143701
- Automating Well Performance Monitoring of Real-Time Data: SPE 141110
The oil and gas industry is diverse, and hence there is no one-size-fits-all IIoT methodology. It is apparent that we as geoscientists and engineers need to establish sophisticated rules and machine learning workflows to be nimble and efficient to tune assets in real-time with predictive and prescriptive probabilistic models.