It’s a challenging time to be a risk manager.
The world is facing a global climate crisis, enduring the worst economic downturn since the great depression, war and a recovery period from a global pandemic.
In addition to these challenges, changes in customer behaviour and expectations give financial services no choice but to create more robust risk modelling and decisioning processes. This is an urgent call to action to react faster to changes. Banks and financial institutions can accomplish this by tapping into new data sources to understand customer behaviour better so they can open their services to new, more financially inclusive segments.
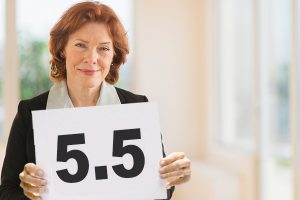
With this in mind, risk managers are seeking ways to improve their risk modelling and decisioning capabilities via more advanced data, analytics and technology management tools and methodologies. Although financial institutions are at different maturity levels in each of these capabilities – and the actions each needs to take – there are common pillars of intelligent risk management today.
Combining alternative data with traditional data sources
Risk managers are exploring the value of internal and external non-traditional data sources for better risk modelling and decisioning. These include customers’ ATM usage, call centre feedback, underwriter, and collector conversations with customers, especially about their cash flow.
Using AI/ML models for deeper insights
Machine learning models are highly automated, less labor intensive and excel at capturing non-additive relationships in data. They can be highly automated and less labour intensive. They are mighty where domain knowledge is weaker. Risk managers prefer ML models to explore the added value of using non-traditional data sources.
Streamlining credit decision processes
After risk decisioning data and models are changed, streamlining credit decisioning processes becomes a natural next best action. New data sources and models require new credit decision strategies, which will require a revision to credit processes. This revision should enable streamlining and designing customer-centric credit journeys.
Using an agile, integrated risk modelling and decisioning platform
Risk managers need a single, integrated, real-time credit risk modeling and decision management platform to implement the first three pillars above successfully. The platform should combine data management, model development, model management and decisioning, and enhanced capabilities like natural language processing (NLP), machine and deep learning and optimization.
To explore these concepts more, see how SAS customers have successfully transformed their risk management capabilities.