In my last two blog posts, I outlined the challenges faced by many local authorities across the country (part 1 and part 2). The role of local authorities is to provide a range of core value-for-money services and as many additional community services as they can. Alas, the central government has reduced budget support in real terms year after year. Saving expectations for local authorities have been crippling, with the government requiring councils to cut millions from their budgets.
This will require a change in culture. Councils have an abundance of data collected from all departments, e.g., the contact centre, planning teams, housing and social care. In addition, there is the data collected by working with many partner organisations. These include the NHS, CCGs, police and the voluntary sector.
Typically, councils use data in silos, predominantly for reporting and business intelligence purposes. In its 2018 survey to chief executives in local authorities, PWC found that councils were fairly confident in their business intelligence (64%) and information governance (71%) capabilities, but much less so (48%) when it came to data and analytics. It is fair to say that the focus hitherto has been a rearview approach to data, looking at what has happened to resident services for reporting purposes. What I am proposing is making the leap to a more forward-looking, “what will happen” approach to services.
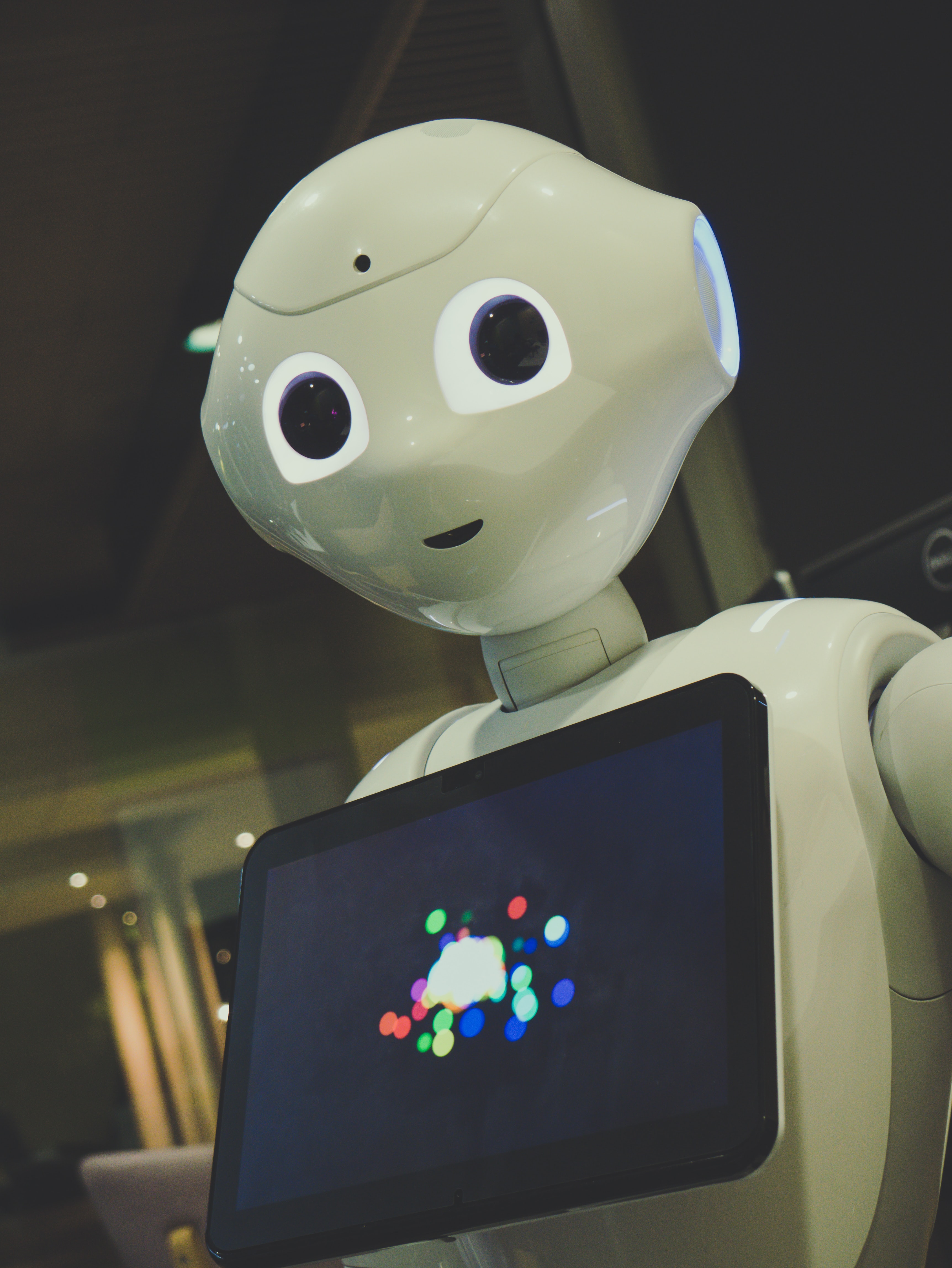
Starting small with text analytics and NLP
In this post, I explore how authorities can use text analytics and natural language processing as an entry point for moving from reporting on resident services to predicting what is likely to happen to those same services going forward. Text analytics is the analysis of unstructured text data, such as data from resident contact centres.
Councils could use data from resident contact centres to solve a number of issues. They could understand what their residents are saying. They can also highlight causes of dissatisfaction and complaint, and identify vulnerable residents through certain key phrases. Moreover, it has the potential to yield enormous benefits. For example, Nationwide Building Society was looking at reducing the frequency contact volumes. Using text analytics, they concluded that more than half of the browser-based messages received could have been avoided entirely. Local authorities could use a similar approach with contact centres to reduce volumes of interaction. Saved time could be better used in service delivery.
Unstructured text data in councils
Residents can contact their councils in different ways: contact centre, online reporting, through their local councillors and social media. These channels yield an abundance of unstructured data. Since this is predominantly text-based, it does not lend itself to conventional analysis, but may include numeric values too. Many organisations, both public and private, do very little analysis on this type of data, let alone any complex analytics, because it is too hard. Instead, a mere count of the number of complaints is made for reporting purposes and manual analysis is usually done. Manual review is very time consuming and inconsistent. By reading a sample you can very easily miss the big picture.
3 benefits from text analytics and NLP
1. Getting to the root of resident dissatisfaction
Councils receive volumes of complaints from residents. By analysing the data sources mentioned above, authorities can start to understand the data from complaints and get answers to some important questions:
- What are the main causes of complaints?
- Are there common themes of complaints?
- Are there certain words and phrases that usually indicate dissatisfaction with a particular service?
- What are the primary reasons people get in touch with the council?
- Which complaints are likely to escalate?
- Could any of the contacts have been avoided?
Answering such questions will lead to an improvement in resident satisfaction by allowing a swifter resolution to their complaints. Understanding if any queries could have been avoided altogether means council staff can spend their time on other council priorities.
Nationwide Building Society, in its analysis of member contacts, found that a number of them were for proof of travel insurance, a benefit offered to their members. This information was readily available on the organisation's website but was not easy to find. Some simple corrective measures allowed members to help themselves – and additionally reduced the burden on the contact centre. Council contact centres could use a similar approach to ascertain if some queries had easy resolutions.
2. Improved future service planning
Councils could gather additional intel from analysing the same data to enrich service planning for the future. A major UK insurance company was losing thousands of policies every year due to customer complaints. Using SAS Text Analytics, it was able to find out which type of complaint drove higher attrition rates and which customer was likely to leave based on the wording of the complaint. The company used the results to implement a retention programme.
The objectives of an insurance company and a local authority are invariably different. However, the concept of using resident feedback to uncover emerging trends to develop constructive strategies that improve the future delivery of public services is comparable.
3. Identifying vulnerable residents
Another benefit of analysing contact centre data is the ability to identify vulnerable residents by picking up keywords, such as "illness," "unemployment," "homeless," etc. Identifying families or individuals in distress means councils can take preventive action to divert the correct action at an early stage. This saves time and resources and possible repeated contacts.
Having looked at a number of benefits from analysing contact centre data with text analytics and NLP, we can briefly look at how text analytics works.
SAS Text Analytics and NLP
Certain analytical solutions give you the ability to ingest text data of any format and analyse unstructured data to ascertain root cause analysis, such as what residents are complaining about. And if you can easily deploy the solution at scale, in the cloud or on-premises, that's even better. SAS Visual Text Analytics uses a hybrid approach to analyse unstructured data that encompasses both machine learning (the analytics bit) and linguistic rules (the language bit). You can use SAS to determine complaint resolution success, find out how many interactions it took for resolution, and determine the mood of the resident involved.
Natural language processing can offer invaluable benefits to councils and increase resident satisfaction, especially for a sector facing such serious budgetary constraints. Click To TweetIn conclusion, NLP can offer invaluable benefits to councils and increase resident satisfaction, especially for a sector facing such serious budgetary constraints. Authorities will start to see their return on investment fairly quickly with improved services and alleviated funding pressures. The applications of text analytics and NLP are plentiful. Councils can use them to improve complaint resolution and identify vulnerable residents. And they can also expand applications to other departments, such as housing and social care, to improve operational efficiency.
For more information on reducing residents’ complaints and improving intelligence for policy and service planning, see this infographic.