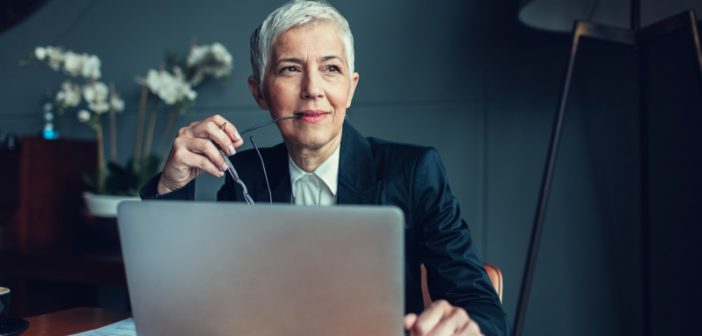
Jim Harris addresses some of the most common questions and challenges big data poses for data quality.
Jim Harris addresses some of the most common questions and challenges big data poses for data quality.
.@philsimon on bridging the IT-business divide once and for all.
As a youngster in the 70s and 80s, Star Trek inspired my imagination and fostered a great love for science, technology and reading. (See the embedded Star Trek infographic for some interesting factoids – did you know that there were 28 crew member deaths by those wearing red shirts?) Captain Kirk and the
It’s been an amazing journey with Hadoop. As we discussed in an earlier blog, Hadoop is informing the basis of a comprehensive data enterprise platform that can power an ecosystem of analytic applications to uncover rich insights on large sets of data. With YARN (Yet Another Resource Negotiator) as its
.@philsimon on the new challenges of an old problem.
In the UK, technology trends move a little slower than for our US counterparts. It was about 5 years ago when I first met a data leader at a conference on this side of the pond who was actively engaging in large scale big data projects. This wasn’t a presenter
I am currently cycling through a schema-on-read data modeling process on a specific task for one of my clients. I have been presented with a data set and have been asked to consider how that data can be best analyzed using a graph-based data management system. My process is to
In my prior two posts, I explored some of the issues associated with data integration for big data and particularly, the conceptual data lake in which source data sets are accumulated and stored, awaiting access from interested data consumers. One of the distinctive features of this approach is the transition
Integrating big data into existing data management processes and programs has become something of a siren call for organizations on the odyssey to become 21st century data-driven enterprises. To help save some lost time, this post offers a few tips for successful big data integration.
There is a time and a place for everything, but the time and place for data quality (DQ) in data integration (DI) efforts always seems like a thing everyone’s not quite sure about. I have previously blogged about the dangers of waiting until the middle of DI to consider, or become forced