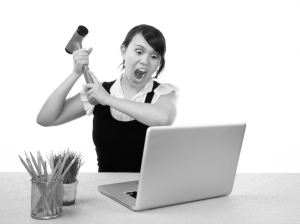
I've always had a morbid fascination with infomercials. Late night television is rife with products to make life better – from turning on the lights to making food instantaneously with zero cleanup. To convince you that you can’t live without their latest gadget, the story always starts out showing someone struggling to perform a seemingly simple task. This hapless individual, inevitably shown in black and white, grows increasingly frustrated before finally giving up and shouting that there must be a better way. The sponsor then introduces their revolutionary product in a rainbow of color. The recently miserable person is now all smiles, surrounded by friends happily using the gadget to perform the task with ease.
Similar to how an infomercial gadget is advertised, data lakes initially promised freedom from rigid schemas and inflexible pipelines – hallmarks of the enterprise data warehouse (EDW). Yet data lakes often fail to deliver on this flexibility and ease of use. To those facing the common challenges of daily interactions with data lakes, frustration levels run high.
Where did the promises go awry?
Promise #1: Stop wasting time with expensive warehouses. Store everything and never worry about data models again.
In the early days, businesses were promised that they could store all manner of data in their data lake. It would free them from the constraints of data models and expensive, proprietary hardware. It would be a repository for everything, hosted on cheap, commodity storage. Consumers would find the valuable data and derive insights without needing to learn a complex taxonomy or having to worry about limited EDW resources.
This doesn’t necessarily happen. One problem with the “store everything” concept is that when data isn't curated and cataloged, it becomes difficult to separate worthwhile data from worthless noise. Without governance to document data lineage and timeliness, useful data gets stale, is never replaced, and just exacerbates the problem. Endless volumes of unsorted data cause more problems than they solve.
Promise #2: Get data that’s not available in enterprise data stores.
Loading data into an enterprise data warehouse involves rigid data quality and referential integrity checks. Yet users often need data that cannot undergo this process. Data sets may be experimental and not worth the effort of certifying, or the data representation may not fit the enterprise data model. Traditional data warehouse pipelines have a long lead time and it just isn’t practical to load every departmental sourced data set.
Data lakes seem to solve this problem nicely. Bypassing the rigor and inflexible ETL of the warehouse, data lakes ingest and make data available with minimal drama. Simply load the data and it's available to use!
While this sounds appealing, the reality of operating in a tactical, departmental data lake is not so simple. Spotty and inconsistent data quality causes problems when a data set must be joined with similar data from the EDW. Tactical decisions to resolve these issues only exacerbate underlying problems. The once lightweight and responsive departmental data lake soon bogs down with multiple MDM and data quality solutions and the content ends up further isolated from the rest of the enterprise’s data.
Promise #3: No more endless waiting for IT.
The ability to operate free of the overhead and scheduling constraints of corporate IT is a compelling reason why many data lakes are established. Data owners rationalize the lack of governance and standardization, believing these repositories are limited in purpose and will never need to be supported by IT.
The reality is completely different. Inefficiencies arise due to the lack of local expertise on the team. The absence of centralized risk and security models – and razor thin metadata – make interoperability a nightmare when teams need to interface with the rest of the enterprise's data assets. The promised data lake becomes more of a data puddle, isolated and restricting.
Resolve: Move past infomercial promises to capture the real value of data lakes.
The unfortunate truth is that there is no single tool, technology or process that you can buy, build or implement to make data and analytics tasks effortless and inexpensive. Data is messy and ever-changing. Business users demand agility, performance and stability in equal measure to feed the analytics engines that drive insights. For enterprise data and analytics, there is no silver bullet.
Rather than a frustrating problem that can be solved by a new gadget, think about data and analytics as a living, managed ecosystem. Data is ingested, processed and consumed in much the same way plants ingest nutrients from the earth before being consumed by animals as food. Raw data sources provide nutrients for the processing engines that feed downstream analytics and operational systems. But unlike a natural ecosystem, a data and analytics ecosystem must be carefully planned and managed. It must be monitored for health and periodically pruned and updated. Conscious planning and design ensure that all parts of the ecosystem are in balance and at peak functioning.
Building a data and analytics ecosystem must be undertaken with a solid strategic vision. This vision should include a broad spectrum of capabilities that can address current and future business needs. It can serve as a road map to plan, grow and realize business value from the investment. One way to plan a data and analytics strategy is to start with a capability model. Here is an example developed by Core Compete.
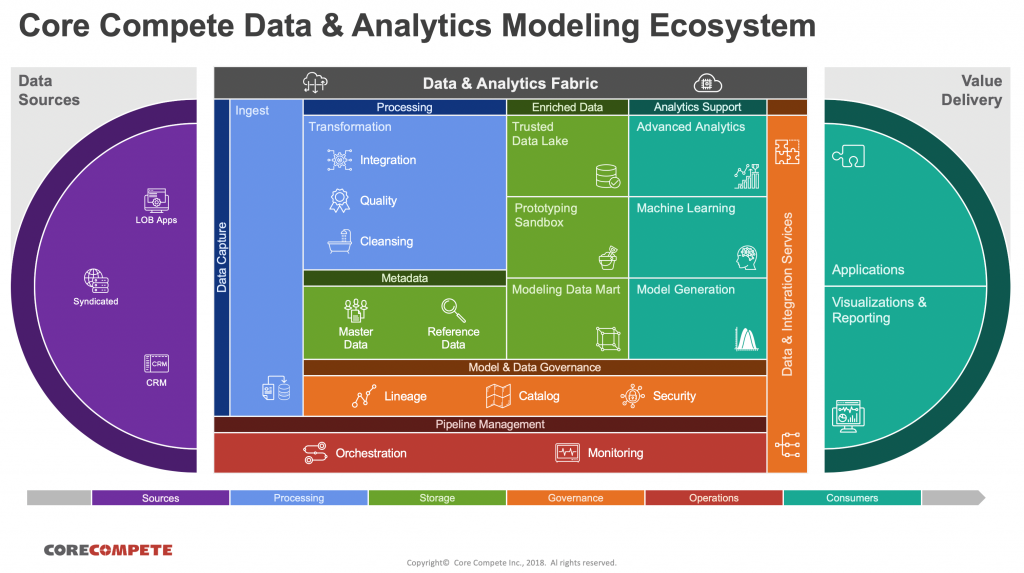
A capability model is a useful tool to kick-start discussions on data and analytics strategy, and it can serve as a sounding board for “Have you thought about XYZ?” types of questions. It is not an architecture, nor is it dependent upon one technology or vendor.
In the diagram, you can see that data lakes have a central position in the ecosystem. They provide support for unstructured data, inexpensive storage and operational flexibility. However, they are not standalone tools. While important, they are just one part of a comprehensive, strategic architecture. To be effective, data lakes need to be supported by robust governance, well-designed integration patterns, and consolidated orchestration and data management protocols.
Enterprise data and analytics is a complicated subject with no one “right” answer. In the next blog post, we’ll explore how to avoid pitfalls so that the data lake will produce fewer frustrated users and more satisfied data scientists and IT professionals.
Read an article: Data lake and data warehouse – Know the difference